Open Access
ARTICLE
End-to-End Audio Pattern Recognition Network for Overcoming Feature Limitations in Human-Machine Interaction
1 School of Information Science and Engineering, Yanshan University, Qinhuangdao, 066004, China
2 The Key Laboratory for Special Fiber and Fiber Sensor of Hebei Province, Yanshan University, Qinhuangdao, 066004, China
3 School of Electrical Engineering, Yanshan University, Qinhuangdao, 066004, China
4 Key Laboratory of Industrial Computer Control Engineering of Hebei Province, Yanshan University, Qinhuangdao, 066004, China
* Corresponding Author: Yaqian Li. Email:
Computers, Materials & Continua 2025, 83(2), 3187-3210. https://doi.org/10.32604/cmc.2025.061920
Received 06 December 2024; Accepted 18 February 2025; Issue published 16 April 2025
Abstract
In recent years, audio pattern recognition has emerged as a key area of research, driven by its applications in human-computer interaction, robotics, and healthcare. Traditional methods, which rely heavily on handcrafted features such as Mel filters, often suffer from information loss and limited feature representation capabilities. To address these limitations, this study proposes an innovative end-to-end audio pattern recognition framework that directly processes raw audio signals, preserving original information and extracting effective classification features. The proposed framework utilizes a dual-branch architecture: a global refinement module that retains channel and temporal details and a multi-scale embedding module that captures high-level semantic information. Additionally, a guided fusion module integrates complementary features from both branches, ensuring a comprehensive representation of audio data. Specifically, the multi-scale audio context embedding module is designed to effectively extract spatiotemporal dependencies, while the global refinement module aggregates multi-scale channel and temporal cues for enhanced modeling. The guided fusion module leverages these features to achieve efficient integration of complementary information, resulting in improved classification accuracy. Experimental results demonstrate the model’s superior performance on multiple datasets, including ESC-50, UrbanSound8K, RAVDESS, and CREMA-D, with classification accuracies of 93.25%, 90.91%, 92.36%, and 70.50%, respectively. These results highlight the robustness and effectiveness of the proposed framework, which significantly outperforms existing approaches. By addressing critical challenges such as information loss and limited feature representation, this work provides new insights and methodologies for advancing audio classification and multimodal interaction systems.Keywords
Cite This Article
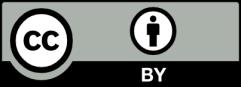
This work is licensed under a Creative Commons Attribution 4.0 International License , which permits unrestricted use, distribution, and reproduction in any medium, provided the original work is properly cited.