Open Access
ARTICLE
An Attention-Based CNN Framework for Alzheimer’s Disease Staging with Multi-Technique XAI Visualization
1 Department of Computer Engineering, Karabuk University, Karabük, 78050, Türkiye
2 Department of Computer Science, College of Computer Science and Mathematics, Tikrit University, Tikrit, 34001, Iraq
* Corresponding Author: Emrullah Sonuç. Email:
(This article belongs to the Special Issue: Medical Imaging Based Disease Diagnosis Using AI)
Computers, Materials & Continua 2025, 83(2), 2947-2969. https://doi.org/10.32604/cmc.2025.062719
Received 25 December 2024; Accepted 05 March 2025; Issue published 16 April 2025
Abstract
Alzheimer’s disease (AD) is a significant challenge in modern healthcare, with early detection and accurate staging remaining critical priorities for effective intervention. While Deep Learning (DL) approaches have shown promise in AD diagnosis, existing methods often struggle with the issues of precision, interpretability, and class imbalance. This study presents a novel framework that integrates DL with several eXplainable Artificial Intelligence (XAI) techniques, in particular attention mechanisms, Gradient-Weighted Class Activation Mapping (Grad-CAM), and Local Interpretable Model-Agnostic Explanations (LIME), to improve both model interpretability and feature selection. The study evaluates four different DL architectures (ResMLP, VGG16, Xception, and Convolutional Neural Network (CNN) with attention mechanism) on a balanced dataset of 3714 MRI brain scans from patients aged 70 and older. The proposed CNN with attention model achieved superior performance, demonstrating 99.18% accuracy on the primary dataset and 96.64% accuracy on the ADNI dataset, significantly advancing the state-of-the-art in AD classification. The ability of the framework to provide comprehensive, interpretable results through multiple visualization techniques while maintaining high classification accuracy represents a significant advancement in the computational diagnosis of AD, potentially enabling more accurate and earlier intervention in clinical settings.Keywords
Cite This Article
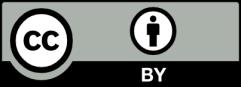
This work is licensed under a Creative Commons Attribution 4.0 International License , which permits unrestricted use, distribution, and reproduction in any medium, provided the original work is properly cited.