Open Access
ARTICLE
Joint Generation of Distractors for Multiple-Choice Questions: A Text-to-Text Approach
Departamento de Ciencias de la Computación, Universidad de Alcalá, Alcalá de Henares, Madrid, 28801, Spain
* Corresponding Author: Eva Garcia-Lopez. Email:
Computers, Materials & Continua 2025, 83(2), 1683-1705. https://doi.org/10.32604/cmc.2025.062004
Received 08 December 2024; Accepted 18 March 2025; Issue published 16 April 2025
Abstract
Generation of good-quality distractors is a key and time-consuming task associated with multiple-choice questions (MCQs), one of the assessment items that have dominated the educational field for years. Recent advances in language models and architectures present an opportunity for helping teachers to generate and update these elements to the required speed and scale of widespread increase in online education. This study focuses on a text-to-text approach for joints generation of distractors for MCQs, where the context, question and correct answer are used as input, while the set of distractors corresponds to the output, allowing the generation of three distractors in a single model inference. By fine-tuning FlanT5 models and LongT5 with TGlobal attention using a RACE-based dataset, the potential of this approach is explored, demonstrating an improvement in the BLEU and ROUGE-L metrics when compared to previous works and a GPT-3.5 baseline. Additionally, BERTScore is introduced in the evaluation, showing that the fine-tuned models generate distractors semantically close to the reference, but the GPT-3.5 baseline still outperforms in this area. A tendency toward duplicating distractors is noted, although models fine-tuned with Low-Rank Adaptation (LoRA) and 4-bit quantization showcased a significant reduction in duplicated distractors.Keywords
Cite This Article
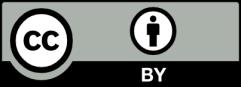
This work is licensed under a Creative Commons Attribution 4.0 International License , which permits unrestricted use, distribution, and reproduction in any medium, provided the original work is properly cited.