Open Access
ARTICLE
A Novel Approach to Enhanced Cancelable Multi-Biometrics Personal Identification Based on Incremental Deep Learning
1 Department of Computer and Information Sciences, Northumbria University, London, E1 7HT, UK
2 Department of Information Technology, College of Computer and Information Sciences, Princess Nourah bint Abdulrahman University, Riyadh, 11671, Saudi Arabia
3 Department of Computer Science, College of Computer and Information Sciences, Princess Nourah bint Abdulrahman University, Riyadh, 11671, Saudi Arabia
* Corresponding Authors: Souham Meshoul. Email: ; Mohamed Batouche. Email:
Computers, Materials & Continua 2025, 83(2), 1727-1752. https://doi.org/10.32604/cmc.2025.063227
Received 08 January 2025; Accepted 04 March 2025; Issue published 16 April 2025
Abstract
The field of biometric identification has seen significant advancements over the years, with research focusing on enhancing the accuracy and security of these systems. One of the key developments is the integration of deep learning techniques in biometric systems. However, despite these advancements, certain challenges persist. One of the most significant challenges is scalability over growing complexity. Traditional methods either require maintaining and securing a growing database, introducing serious security challenges, or relying on retraining the entire model when new data is introduced—a process that can be computationally expensive and complex. This challenge underscores the need for more efficient methods to scale securely. To this end, we introduce a novel approach that addresses these challenges by integrating multimodal biometrics, cancelable biometrics, and incremental learning techniques. This work is among the first attempts to seamlessly incorporate deep cancelable biometrics with dynamic architectural updates, applied incrementally to the deep learning model as new users are enrolled, achieving high performance with minimal catastrophic forgetting. By leveraging a One-Dimensional Convolutional Neural Network (1D-CNN) architecture combined with a hybrid incremental learning approach, our system achieves high recognition accuracy, averaging 98.98% over incrementing datasets, while ensuring user privacy through cancelable templates generated via a pre-trained CNN model and random projection. The approach demonstrates remarkable adaptability, utilizing the least intrusive biometric traits like facial features and fingerprints, ensuring not only robust performance but also long-term serviceability.Keywords
Cite This Article
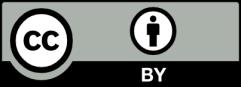
This work is licensed under a Creative Commons Attribution 4.0 International License , which permits unrestricted use, distribution, and reproduction in any medium, provided the original work is properly cited.