Open Access
ARTICLE
Leveraging Edge Optimize Vision Transformer for Monkeypox Lesion Diagnosis on Mobile Devices
1 Chitkara University Institute of Engineering and Technology, Chitkara University, Rajpura, 140401, Punjab, India
2 Centre for Research Impact and Outcome, Chitkara University, Rajpura, 140401, Punjab, India
3 Department of Computer Engineering & Applications, G.L.A. University, Mathura, 281406, India
4 Department of Data Science, University of Salford, Manchester, M54WT, UK
5 University Centre for Research and Development, Chandigarh University, Mohali, 140413, Punjab, India
6 Division of Research and Development, Lovely Professional University, Phagwara, 144411, India
7 Department of Management, College of Business Administration, Princess Nourah bint Abdulrahman University, P.O. Box 84428, Riyadh, 11671, Saudi Arabia
* Corresponding Authors: Bhisham Sharma. Email: ; Surbhi Bhatia Khan. Email:
(This article belongs to the Special Issue: Medical Imaging Based Disease Diagnosis Using AI)
Computers, Materials & Continua 2025, 83(2), 3227-3245. https://doi.org/10.32604/cmc.2025.062376
Received 17 December 2024; Accepted 28 February 2025; Issue published 16 April 2025
Abstract
Rapid and precise diagnostic tools for Monkeypox (Mpox) lesions are crucial for effective treatment because their symptoms are similar to those of other pox-related illnesses, like smallpox and chickenpox. The morphological similarities between smallpox, chickenpox, and monkeypox, particularly in how they appear as rashes and skin lesions, which can sometimes make diagnosis challenging. Chickenpox lesions appear in many simultaneous phases and are more diffuse, often beginning on the trunk. In contrast, monkeypox lesions emerge progressively and are typically centralized on the face, palms, and soles. To provide accessible diagnostics, this study introduces a novel method for automated monkeypox lesion classification using the HMTNet (Hybrid Mobile Transformer Network). The convolutional layers and Vision Transformers (ViT) are combined to enhance the spatial features. In addition, we replace the classical MHSA (Multi-head self-attention) with the WMHSA (Window-based Multi-Head Self-Attention) to effectively capture long-range dependencies within image patches and depth-wise separable convolutions for local feature extraction. We trained and validated HMTNet on the two datasets for binary and multiclass classification. The model achieved 98.38% accuracy for multiclass classification using cross-validation and 99.25% accuracy for binary classification. These findings show that the model has the potential to be a useful diagnostic tool for monkeypox, especially in environments with limited resources.Keywords
Cite This Article
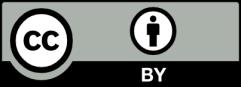
This work is licensed under a Creative Commons Attribution 4.0 International License , which permits unrestricted use, distribution, and reproduction in any medium, provided the original work is properly cited.