Open Access
ARTICLE
Integration of Federated Learning and Graph Convolutional Networks for Movie Recommendation Systems
1 Department of Software Convergence, Soonchunhyang University, Asan, 31538, Republic of Korea
2 Department of Computer Software and Engineering, Soonchunhyang University, Asan, 31538, Republic of Korea
3 AI·SW Education Institute, Soonchunhyang University, Asan, 31538, Republic of Korea
* Corresponding Authors: Dae-Young Kim. Email: ; Kyuwon Park. Email:
(This article belongs to the Special Issue: Advances in AI Techniques in Convergence ICT)
Computers, Materials & Continua 2025, 83(2), 2041-2057. https://doi.org/10.32604/cmc.2025.061166
Received 18 November 2024; Accepted 01 March 2025; Issue published 16 April 2025
Abstract
Recommendation systems (RSs) are crucial in personalizing user experiences in digital environments by suggesting relevant content or items. Collaborative filtering (CF) is a widely used personalization technique that leverages user-item interactions to generate recommendations. However, it struggles with challenges like the cold-start problem, scalability issues, and data sparsity. To address these limitations, we develop a Graph Convolutional Networks (GCNs) model that captures the complex network of interactions between users and items, identifying subtle patterns that traditional methods may overlook. We integrate this GCNs model into a federated learning (FL) framework, enabling the model to learn from decentralized datasets. This not only significantly enhances user privacy— a significant improvement over conventional models but also reassures users about the safety of their data. Additionally, by securely incorporating demographic information, our approach further personalizes recommendations and mitigates the cold-start issue without compromising user data. We validate our RSs model using the open MovieLens dataset and evaluate its performance across six key metrics: Precision, Recall, Area Under the Receiver Operating Characteristic Curve (ROC-AUC), F1 Score, Normalized Discounted Cumulative Gain (NDCG), and Mean Reciprocal Rank (MRR). The experimental results demonstrate significant enhancements in recommendation quality, underscoring that combining GCNs with CF in a federated setting provides a transformative solution for advanced recommendation systems.Keywords
Cite This Article
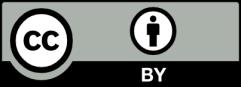
This work is licensed under a Creative Commons Attribution 4.0 International License , which permits unrestricted use, distribution, and reproduction in any medium, provided the original work is properly cited.