Open Access
ARTICLE
A New Cybersecurity Approach Enhanced by xAI-Derived Rules to Improve Network Intrusion Detection and SIEM
1 Centro Direzionale, Department of Engineering, University of Naples ‘Parthenope’, Isola C4, Napoli, 80133, Italy
2 Department of Computer and Information Science, Software and Systems, Linköping University, Linköping, 58183, Sweden
3 ITTI Sp. z o.o., Poznań, 61-612, Poland
4 Faculty of Telecommunications, Computer Science and Electrical Engineering, Bydgoszcz University of Science and Technology, Bydgoszcz, 85-796, Poland
* Corresponding Author: Michał Choraś. Email:
(This article belongs to the Special Issue: Artificial Intelligence Current Perspectives and Alternative Paths: From eXplainable AI to Generative AI and Data Visualization Technologies)
Computers, Materials & Continua 2025, 83(2), 1607-1621. https://doi.org/10.32604/cmc.2025.062801
Received 28 December 2024; Accepted 05 March 2025; Issue published 16 April 2025
Abstract
The growing sophistication of cyberthreats, among others the Distributed Denial of Service attacks, has exposed limitations in traditional rule-based Security Information and Event Management systems. While machine learning–based intrusion detection systems can capture complex network behaviours, their “black-box” nature often limits trust and actionable insight for security operators. This study introduces a novel approach that integrates Explainable Artificial Intelligence—xAI—with the Random Forest classifier to derive human-interpretable rules, thereby enhancing the detection of Distributed Denial of Service (DDoS) attacks. The proposed framework combines traditional static rule formulation with advanced xAI techniques—SHapley Additive exPlanations and Scoped Rules - to extract decision criteria from a fully trained model. The methodology was validated on two benchmark datasets, CICIDS2017 and WUSTL-IIOT-2021. Extracted rules were evaluated against conventional Security Information and Event Management Systems rules with metrics such as precision, recall, accuracy, balanced accuracy, and Matthews Correlation Coefficient. Experimental results demonstrate that xAI-derived rules consistently outperform traditional static rules. Notably, the most refined xAI-generated rule achieved near-perfect performance with significantly improved detection of DDoS traffic while maintaining high accuracy in classifying benign traffic across both datasets.Keywords
Cite This Article
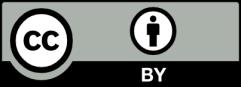
This work is licensed under a Creative Commons Attribution 4.0 International License , which permits unrestricted use, distribution, and reproduction in any medium, provided the original work is properly cited.