Open Access
ARTICLE
DCS-SOCP-SVM: A Novel Integrated Sampling and Classification Algorithm for Imbalanced Datasets
School of Mathematics and Statistics, Xidian University, Xi’an, 710071, China
* Corresponding Author: Xuewen Mu. Email:
Computers, Materials & Continua 2025, 83(2), 2143-2159. https://doi.org/10.32604/cmc.2025.060739
Received 08 November 2024; Accepted 28 January 2025; Issue published 16 April 2025
Abstract
When dealing with imbalanced datasets, the traditional support vector machine (SVM) tends to produce a classification hyperplane that is biased towards the majority class, which exhibits poor robustness. This paper proposes a high-performance classification algorithm specifically designed for imbalanced datasets. The proposed method first uses a biased second-order cone programming support vector machine (B-SOCP-SVM) to identify the support vectors (SVs) and non-support vectors (NSVs) in the imbalanced data. Then, it applies the synthetic minority over-sampling technique (SV-SMOTE) to oversample the support vectors of the minority class and uses the random under-sampling technique (NSV-RUS) multiple times to undersample the non-support vectors of the majority class. Combining the above-obtained minority class data set with multiple majority class datasets can obtain multiple new balanced data sets. Finally, SOCP-SVM is used to classify each data set, and the final result is obtained through the integrated algorithm. Experimental results demonstrate that the proposed method performs excellently on imbalanced datasets.Keywords
Cite This Article
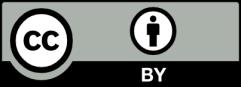
This work is licensed under a Creative Commons Attribution 4.0 International License , which permits unrestricted use, distribution, and reproduction in any medium, provided the original work is properly cited.