Open Access
ARTICLE
Fake News Detection Based on Cross-Modal Ambiguity Computation and Multi-Scale Feature Fusion
1 State Key Laboratory of Media Convergence and Communication, Communication University of China, Beijing, 100024, China
2 School of Computer Science and Engineering, Guangxi Normal University, Guilin, 541004, China
3 Department of Computer Science, Nipissing University, North Bay, ON P1B 8L7, Canada
* Corresponding Authors: Wenqian Shang. Email: ; Tong Yi. Email:
Computers, Materials & Continua 2025, 83(2), 2659-2675. https://doi.org/10.32604/cmc.2025.060025
Received 22 October 2024; Accepted 21 February 2025; Issue published 16 April 2025
Abstract
With the rapid growth of social media, the spread of fake news has become a growing problem, misleading the public and causing significant harm. As social media content is often composed of both images and text, the use of multimodal approaches for fake news detection has gained significant attention. To solve the problems existing in previous multi-modal fake news detection algorithms, such as insufficient feature extraction and insufficient use of semantic relations between modes, this paper proposes the MFFFND-Co (Multimodal Feature Fusion Fake News Detection with Co-Attention Block) model. First, the model deeply explores the textual content, image content, and frequency domain features. Then, it employs a Co-Attention mechanism for cross-modal fusion. Additionally, a semantic consistency detection module is designed to quantify semantic deviations, thereby enhancing the performance of fake news detection. Experimentally verified on two commonly used datasets, Twitter and Weibo, the model achieved F1 scores of 90.0% and 94.0%, respectively, significantly outperforming the pre-modified MFFFND (Multimodal Feature Fusion Fake News Detection with Attention Block) model and surpassing other baseline models. This improves the accuracy of detecting fake information in artificial intelligence detection and engineering software detection.Keywords
Cite This Article
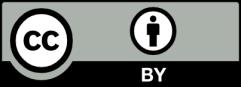
This work is licensed under a Creative Commons Attribution 4.0 International License , which permits unrestricted use, distribution, and reproduction in any medium, provided the original work is properly cited.