Open Access
ARTICLE
Event-Driven Attention Network: A Cross-Modal Framework for Efficient Image-Text Retrieval in Mass Gathering Events
1 School of Computer Science and Engineering, University of Electronic Science and Technology of China, Chengdu, 611731, China
2 School of Information and Software Engineering, University of Electronic Science and Technology of China, Chengdu, 611731, China
3 Kashi Institute of Electronics and Information Industry, Kashi, 844508, China
* Corresponding Author: Ye Li. Email:
# Both Kamil Yasen and Heyan Jin contributed equally to this work
Computers, Materials & Continua 2025, 83(2), 3277-3301. https://doi.org/10.32604/cmc.2025.061037
Received 15 November 2024; Accepted 09 January 2025; Issue published 16 April 2025
Abstract
Research on mass gathering events is critical for ensuring public security and maintaining social order. However, most of the existing works focus on crowd behavior analysis areas such as anomaly detection and crowd counting, and there is a relative lack of research on mass gathering behaviors. We believe real-time detection and monitoring of mass gathering behaviors are essential for migrating potential security risks and emergencies. Therefore, it is imperative to develop a method capable of accurately identifying and localizing mass gatherings before disasters occur, enabling prompt and effective responses. To address this problem, we propose an innovative Event-Driven Attention Network (EDAN), which achieves image-text matching in the scenario of mass gathering events with good results for the first time. Traditional image-text retrieval methods based on global alignment are difficult to capture the local details within complex scenes, limiting retrieval accuracy. While local alignment-based methods are more effective at extracting detailed features, they frequently process raw textual features directly, which often contain ambiguities and redundant information that can diminish retrieval efficiency and degrade model performance. To overcome these challenges, EDAN introduces an Event-Driven Attention Module that adaptively focuses attention on image regions or textual words relevant to the event type. By calculating the semantic distance between event labels and textual content, this module effectively significantly reduces computational complexity and enhances retrieval efficiency. To validate the effectiveness of EDAN, we construct a dedicated multimodal dataset tailored for the analysis of mass gathering events, providing a reliable foundation for subsequent studies. We conduct comparative experiments with other methods on our dataset, the experimental results demonstrate the effectiveness of EDAN. In the image-to-text retrieval task, EDAN achieved the best performance on the R@5 metric, while in the text-to-image retrieval task, it showed superior results on both R@10 and R@5 metrics. Additionally, EDAN excelled in the overall Rsum metric, achieving the best performance. Finally, ablation studies further verified the effectiveness of event-driven attention module.Keywords
Cite This Article
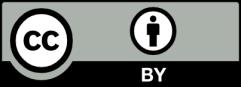
This work is licensed under a Creative Commons Attribution 4.0 International License , which permits unrestricted use, distribution, and reproduction in any medium, provided the original work is properly cited.