Open Access
ARTICLE
A Lightweight Convolutional Neural Network with Squeeze and Excitation Module for Security Authentication Using Wireless Channel
1 College of Computer Science, Beijing Information Science and Technology University, Beijing, 100192, China
2 School of Information and Communication Engineering, Beijing University of Posts and Telecommunications, Beijing, 100876, China
3 School of Mathematical Sciences, Peking University, Beijing, 100871, China
* Corresponding Author: Xiaoying Qiu. Email:
(This article belongs to the Special Issue: Applications of Artificial Intelligence for Information Security)
Computers, Materials & Continua 2025, 83(2), 2025-2040. https://doi.org/10.32604/cmc.2025.061869
Received 05 December 2024; Accepted 10 February 2025; Issue published 16 April 2025
Abstract
Physical layer authentication (PLA) in the context of the Internet of Things (IoT) has gained significant attention. Compared with traditional encryption and blockchain technologies, PLA provides a more computationally efficient alternative to exploiting the properties of the wireless medium itself. Some existing PLA solutions rely on static mechanisms, which are insufficient to address the authentication challenges in fifth generation (5G) and beyond wireless networks. Additionally, with the massive increase in mobile device access, the communication security of the IoT is vulnerable to spoofing attacks. To overcome the above challenges, this paper proposes a lightweight deep convolutional neural network (CNN) equipped with squeeze and excitation module (SE module) in dynamic wireless environments, namely SE-ConvNet. To be more specific, a convolution factorization is developed to reduce the complexity of PLA models based on deep learning. Moreover, an SE module is designed in the deep CNN to enhance useful features and maximize authentication accuracy. Compared with the existing solutions, the proposed SE-ConvNet enabled PLA scheme performs excellently in mobile and time-varying wireless environments while maintaining lower computational complexity.Keywords
Cite This Article
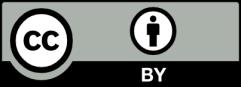
This work is licensed under a Creative Commons Attribution 4.0 International License , which permits unrestricted use, distribution, and reproduction in any medium, provided the original work is properly cited.