Open Access
ARTICLE
Real-Time Proportional-Integral-Derivative (PID) Tuning Based on Back Propagation (BP) Neural Network for Intelligent Vehicle Motion Control
1 School of Information Science and Technology, Northwest University, Xi’an, 710127, China
2 State-Province Joint Engineering and Research Center of Advanced Networking and Intelligent Information Services, Northwest University, Xi’an, 710127, China
3 Generative Artificial Intelligence and Mixed Reality Key Laboratory of Higher Education Institutions in Shaanxi Province, Xi’an, 710127, China
4 Shaanxi Silk Road Cultural Heritage Digital Protection and Inheritance Collaborative Innovation Center, Xi’an, 710127, China
5 School of Art, Northwest University, Xi’an, 710127, China
6 Network and Data Center, Northwest University, Xi’an, 710127, China
* Corresponding Author: Qiyao Hu. Email:
(This article belongs to the Special Issue: Collaborative Edge Intelligence and Its Emerging Applications)
Computers, Materials & Continua 2025, 83(2), 2375-2401. https://doi.org/10.32604/cmc.2025.061894
Received 05 December 2024; Accepted 24 January 2025; Issue published 16 April 2025
Abstract
Over 1.3 million people die annually in traffic accidents, and this tragic fact highlights the urgent need to enhance the intelligence of traffic safety and control systems. In modern industrial and technological applications and collaborative edge intelligence, control systems are crucial for ensuring efficiency and safety. However, deficiencies in these systems can lead to significant operational risks. This paper uses edge intelligence to address the challenges of achieving target speeds and improving efficiency in vehicle control, particularly the limitations of traditional Proportional-Integral-Derivative (PID) controllers in managing nonlinear and time-varying dynamics, such as varying road conditions and vehicle behavior, which often result in substantial discrepancies between desired and actual speeds, as well as inefficiencies due to manual parameter adjustments. The paper uses edge intelligence to propose a novel PID control algorithm that integrates Backpropagation (BP) neural networks to enhance robustness and adaptability. The BP neural network is first trained to capture the nonlinear dynamic characteristics of the vehicle. The trained network is then combined with the PID controller to form a hybrid control strategy. The output layer of the neural network directly adjusts the PID parameters (, , ), optimizing performance for specific driving scenarios through self-learning and weight adjustments. Simulation experiments demonstrate that our BP neural network-based PID design significantly outperforms traditional methods, with the response time for acceleration from 0 to 1 m/s improved from 0.25 s to just 0.065 s. Furthermore, real-world tests on an intelligent vehicle show its ability to make timely adjustments in response to complex road conditions, ensuring consistent speed maintenance and enhancing overall system performance.Keywords
Cite This Article
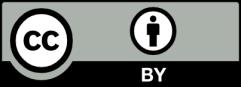
This work is licensed under a Creative Commons Attribution 4.0 International License , which permits unrestricted use, distribution, and reproduction in any medium, provided the original work is properly cited.