Open Access
ARTICLE
HyTiFRec: Hybrid Time-Frequency Dual-Branch Transformer for Sequential Recommendation
1 School of Information Science and Engineering, Zhejiang Sci-Tech University, Hangzhou, 310018, China
2 Department of Vehicle Engineering, Army Academy of Armored Forces, Beijing, 100072, China
3 Performance and Training Center, Army Academy of Armored Forces, Beijing, 100072, China
* Corresponding Authors: Peng Wu. Email: ; Xiaoming Zhang. Email:
(This article belongs to the Special Issue: Advances in Deep Learning and Neural Networks: Architectures, Applications, and Challenges)
Computers, Materials & Continua 2025, 83(2), 1753-1769. https://doi.org/10.32604/cmc.2025.062599
Received 22 December 2024; Accepted 08 February 2025; Issue published 16 April 2025
Abstract
Recently, many Sequential Recommendation methods adopt self-attention mechanisms to model user preferences. However, these methods tend to focus more on low-frequency information while neglecting high-frequency information, which makes them ineffective in balancing users’ long- and short-term preferences. At the same time, many methods overlook the potential of frequency domain methods, ignoring their efficiency in processing frequency information. To overcome this limitation, we shift the focus to the combination of time and frequency domains and propose a novel Hybrid Time-Frequency Dual-Branch Transformer for Sequential Recommendation, namely HyTiFRec. Specifically, we design two hybrid filter modules: the learnable hybrid filter (LHF) and the window hybrid filter (WHF). We combine these with the Efficient Attention (EA) module to form the dual-branch structure to replace the self-attention components in Transformers. The EA module is used to extract sequential and global information. The LHF and WHF modules balance the proportion of different frequency bands, with LHF globally modulating the spectrum in the frequency domain and WHF retaining frequency components within specific local frequency bands. Furthermore, we use a time domain residual information addition operation in the hybrid filter module, which reduces information loss and further facilitates the hybrid of time-frequency methods. Extensive experiments on five widely-used real-world datasets show that our proposed method surpasses state-of-the-art methods.Keywords
Cite This Article
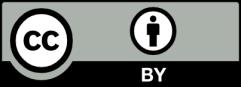
This work is licensed under a Creative Commons Attribution 4.0 International License , which permits unrestricted use, distribution, and reproduction in any medium, provided the original work is properly cited.