Open Access
ARTICLE
Machine Learning Model for Wind Power Forecasting Using Enhanced Multilayer Perceptron
1 Computer Science and Artificial Intelligence Department, College of Computing and Information Technology, University of Bisha, Bisha, 61922, Saudi Arabia
2 Department of Computer, Damietta University, Damietta, 34517, Egypt
3 College of Physics and Electronic Information Engineering, Zhejiang Normal University, Jinhua, 321004, China
4 Department of Mathematics, Faculty of Science, Zagazig University, Zagazig, 44519, Egypt
* Corresponding Author: Ahmed A. Ewees. Email:
Computers, Materials & Continua 2025, 83(2), 2287-2303. https://doi.org/10.32604/cmc.2025.061320
Received 22 November 2024; Accepted 01 February 2025; Issue published 16 April 2025
Abstract
Wind power forecasting plays a crucial role in optimizing the integration of wind energy into the grid by predicting wind patterns and energy output. This enhances the efficiency and reliability of renewable energy systems. Forecasting approaches inform energy management strategies, reduce reliance on fossil fuels, and support the broader transition to sustainable energy solutions. The primary goal of this study is to introduce an effective methodology for estimating wind power through temporal data analysis. This research advances an optimized Multilayer Perceptron (MLP) model using recently proposed metaheuristic optimization algorithms, namely the Fire Hawk Optimizer (FHO) and the Non-Monopolize Search (NO). A modified version of FHO, termed FHONO, is developed by integrating NO as a local search mechanism to enhance the exploration capability and address the shortcomings of the original FHO. The developed FHONO is then employed to optimize the MLP for enhanced wind power prediction. The effectiveness of the proposed FHONO-MLP model is validated using renowned datasets from wind turbines in France. The results of the comparative analysis between FHONO-MLP, conventional MLP, and other optimized versions of MLP show that FHONO-MLP outperforms the others, achieving an average Root Mean Square Error (RMSE) of 0.105, Mean Absolute Error (MAE) of 0.082, and Coefficient of Determination () of 0.967 across all datasets. These findings underscore the significant enhancement in predictive accuracy provided by FHONO and demonstrate its effectiveness in improving wind power forecasting.Keywords
Cite This Article
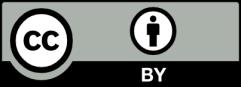
This work is licensed under a Creative Commons Attribution 4.0 International License , which permits unrestricted use, distribution, and reproduction in any medium, provided the original work is properly cited.