Open Access
ARTICLE
Deep Learning Algorithm for Person Re-Identification Based on Dual Network Architecture
1 College of Information Engineering, Harbin University, Harbin, 150086, China
2 Heilongjiang Provincial Key Laboratory of the Intelligent Perception and Intelligent Software, Harbin University, Harbin, 150086, China
3 College of Computer and Control Engineering, Northeast Forestry University, Harbin, 150040, China
4 Heilongjiang Forestry Intelligent Equipment Engineering Research Center, Northeast Forestry University, Harbin, 150040, China
5 Faculty of Computing and Information Technology, King Abdulaziz University, Jeddah, 21589, Saudi Arabia
* Corresponding Authors: Honge Ren. Email: ; Linda Mohaisen. Email:
Computers, Materials & Continua 2025, 83(2), 2889-2905. https://doi.org/10.32604/cmc.2025.061421
Received 24 November 2024; Accepted 13 January 2025; Issue published 16 April 2025
Abstract
Changing a person’s posture and low resolution are the key challenges for person re-identification (ReID) in various deep learning applications. In this paper, we introduce an innovative architecture using a dual attention network that includes an attention module and a joint measurement module of spatial-temporal information. The proposed approach can be classified into two main tasks. Firstly, the spatial attention feature map is formed by aggregating features in the spatial dimension. Additionally, the same operation is carried out on the channel dimension to form channel attention feature maps. Therefore, the receptive field size is adjusted adaptively to mitigate the changing person posture issue. Secondly, we use a joint measurement method for the spatial-temporal information to fully harness the data, and it can also naturally integrate the information into the visual features of supervised ReID and hence overcome the low resolution problem. The experimental results indicate that our proposed algorithm markedly improves the accuracy in addressing changing human postures and low-resolution issues compared with contemporary leading techniques. The proposed method shows superior outcomes on widely recognized benchmarks, which are the Market-1501, MSMT17, and DukeMTMC-reID datasets. Furthermore, the proposed algorithm attains a Rank-1 accuracy of 97.4% and 94.9% mAP (mean Average Precision) on the Market-1501 dataset. Moreover, it achieves a 94.2% Rank-1 accuracy and 91.8% mAP on the DukeMTMC-reID dataset.Keywords
Cite This Article
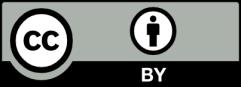
This work is licensed under a Creative Commons Attribution 4.0 International License , which permits unrestricted use, distribution, and reproduction in any medium, provided the original work is properly cited.