Open Access
ARTICLE
Cyclical Training Framework with Graph Feature Optimization for Knowledge Graph Reasoning
1 School of Artificial Intelligence, Jilin University, Changchun, 130012, China
2 Engineering Research Center of Knowledge-Driven Human-Machine Intelligence, MOE, Changchun, 130012, China
3 College of Computer Science and Technology, Jilin University, Changchun, 130012, China
* Corresponding Author: Yuan Tian. Email:
Computers, Materials & Continua 2025, 83(2), 1951-1971. https://doi.org/10.32604/cmc.2025.060134
Received 24 October 2024; Accepted 13 January 2025; Issue published 16 April 2025
Abstract
Knowledge graphs (KGs), which organize real-world knowledge in triples, often suffer from issues of incompleteness. To address this, multi-hop knowledge graph reasoning (KGR) methods have been proposed for interpretable knowledge graph completion. The primary approaches to KGR can be broadly classified into two categories: reinforcement learning (RL)-based methods and sequence-to-sequence (seq2seq)-based methods. While each method has its own distinct advantages, they also come with inherent limitations. To leverage the strengths of each method while addressing their weaknesses, we propose a cyclical training method that alternates for several loops between the seq2seq training phase and the policy-based RL training phase using a transformer architecture. Additionally, a multimodal data encoding (MDE) module is introduced to improve the representation of entities and relations in KGs. The MDE module treats entities and relations as distinct modalities, processing each with a dedicated network specialized for its respective modality. It then combines the representations of entities and relations in a dynamic and fine-grained manner using a gating mechanism. The experimental results from the knowledge graph completion task highlight the effectiveness of the proposed framework. Across five benchmark datasets, our framework achieves an average improvement of 1.7% in the Hits@1 metric and a 0.8% average increase in the Mean Reciprocal Rank (MRR) compared to other strong baseline methods. Notably, the maximum improvement in Hits@1 exceeds 4%, further demonstrating the effectiveness of the proposed approach.Keywords
Cite This Article
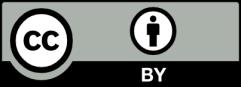
This work is licensed under a Creative Commons Attribution 4.0 International License , which permits unrestricted use, distribution, and reproduction in any medium, provided the original work is properly cited.