Open Access
ARTICLE
DMF: A Deep Multimodal Fusion-Based Network Traffic Classification Model
1 Henan Key Laboratory of Network Cryptography Technology, Information Engineering University, Zhengzhou, 450001, China
2 School of Computer Science and Engineering, University of Electronic Science and Technology of China, Chengdu, 611731, China
* Corresponding Author: Qingjun Yuan. Email:
Computers, Materials & Continua 2025, 83(2), 2267-2285. https://doi.org/10.32604/cmc.2025.061767
Received 02 December 2024; Accepted 24 January 2025; Issue published 16 April 2025
Abstract
With the rise of encrypted traffic, traditional network analysis methods have become less effective, leading to a shift towards deep learning-based approaches. Among these, multimodal learning-based classification methods have gained attention due to their ability to leverage diverse feature sets from encrypted traffic, improving classification accuracy. However, existing research predominantly relies on late fusion techniques, which hinder the full utilization of deep features within the data. To address this limitation, we propose a novel multimodal encrypted traffic classification model that synchronizes modality fusion with multiscale feature extraction. Specifically, our approach performs real-time fusion of modalities at each stage of feature extraction, enhancing feature representation at each level and preserving inter-level correlations for more effective learning. This continuous fusion strategy improves the model’s ability to detect subtle variations in encrypted traffic, while boosting its robustness and adaptability to evolving network conditions. Experimental results on two real-world encrypted traffic datasets demonstrate that our method achieves a classification accuracy of 98.23% and 97.63%, outperforming existing multimodal learning-based methods.Keywords
Cite This Article
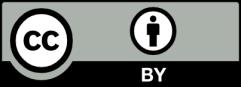
This work is licensed under a Creative Commons Attribution 4.0 International License , which permits unrestricted use, distribution, and reproduction in any medium, provided the original work is properly cited.