Open Access
ARTICLE
Multimodal Neural Machine Translation Based on Knowledge Distillation and Anti-Noise Interaction
1 School of Software, Zhengzhou University of Light Industry, Zhengzhou, 450001, China
2 School of Computer Science and Technology, Zhengzhou University of Light Industry, Zhengzhou, 450001, China
* Corresponding Author: Zengchao Zhu. Email:
Computers, Materials & Continua 2025, 83(2), 2305-2322. https://doi.org/10.32604/cmc.2025.061145
Received 18 November 2024; Accepted 22 January 2025; Issue published 16 April 2025
Abstract
Within the realm of multimodal neural machine translation (MNMT), addressing the challenge of seamlessly integrating textual data with corresponding image data to enhance translation accuracy has become a pressing issue. We saw that discrepancies between textual content and associated images can lead to visual noise, potentially diverting the model’s focus away from the textual data and so affecting the translation’s comprehensive effectiveness. To solve this visual noise problem, we propose an innovative KDNR-MNMT model. The model combines the knowledge distillation technique with an anti-noise interaction mechanism, which makes full use of the synthesized graphic knowledge and local image interaction masks, aiming to extract more effective visual features. Meanwhile, the KDNR-MNMT model adopts a multimodal adaptive gating fusion strategy to enhance the constructive interaction of different modal information. By integrating a perceptual attention mechanism, which uses cross-modal interaction cues within the Transformer framework, our approach notably enhances the quality of machine translation outputs. To confirm the model’s performance, we carried out extensive testing and assessment on the extensively utilized Multi30K dataset. The outcomes of our experiments prove substantial enhancements in our model’s BLEU and METEOR scores, with respective increases of 0.78 and 0.99 points over prevailing methods. This accomplishment affirms the potency of our strategy for mitigating visual interference and heralds groundbreaking advancements within the multimodal NMT domain, further propelling the evolution of this scholarly pursuit.Keywords
Cite This Article
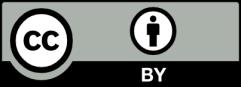
This work is licensed under a Creative Commons Attribution 4.0 International License , which permits unrestricted use, distribution, and reproduction in any medium, provided the original work is properly cited.