Open Access
ARTICLE
A Modified Deep Residual-Convolutional Neural Network for Accurate Imputation of Missing Data
Intelligent System Research Group, Faculty of Computer Science, Universitas Sriwijaya, Palembang, 30139, Indonesia
* Corresponding Author: Siti Nurmaini. Email:
Computers, Materials & Continua 2025, 82(2), 3419-3441. https://doi.org/10.32604/cmc.2024.055906
Received 10 July 2024; Accepted 21 October 2024; Issue published 17 February 2025
Abstract
Handling missing data accurately is critical in clinical research, where data quality directly impacts decision-making and patient outcomes. While deep learning (DL) techniques for data imputation have gained attention, challenges remain, especially when dealing with diverse data types. In this study, we introduce a novel data imputation method based on a modified convolutional neural network, specifically, a Deep Residual-Convolutional Neural Network (DRes-CNN) architecture designed to handle missing values across various datasets. Our approach demonstrates substantial improvements over existing imputation techniques by leveraging residual connections and optimized convolutional layers to capture complex data patterns. We evaluated the model on publicly available datasets, including Medical Information Mart for Intensive Care (MIMIC-III and MIMIC-IV), which contain critical care patient data, and the Beijing Multi-Site Air Quality dataset, which measures environmental air quality. The proposed DRes-CNN method achieved a root mean square error (RMSE) of 0.00006, highlighting its high accuracy and robustness. We also compared with Low Light-Convolutional Neural Network (LL-CNN) and U-Net methods, which had RMSE values of 0.00075 and 0.00073, respectively. This represented an improvement of approximately 92% over LL-CNN and 91% over U-Net. The results showed that this DRes-CNN-based imputation method outperforms current state-of-the-art models. These results established DRes-CNN as a reliable solution for addressing missing data.Keywords
Cite This Article
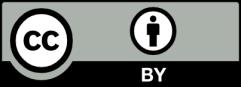
This work is licensed under a Creative Commons Attribution 4.0 International License , which permits unrestricted use, distribution, and reproduction in any medium, provided the original work is properly cited.