Open Access
ARTICLE
ACSF-ED: Adaptive Cross-Scale Fusion Encoder-Decoder for Spatio-Temporal Action Detection
1 College of Publishing, University of Shanghai for Science and Technology, Shanghai, 200093, China
2 Institute of Information Technology, Shanghai Baosight Software Co., Ltd., Shanghai, 200940, China
* Corresponding Author: Zehua Gu. Email:
(This article belongs to the Special Issue: Machine Vision Detection and Intelligent Recognition, 2nd Edition)
Computers, Materials & Continua 2025, 82(2), 2389-2414. https://doi.org/10.32604/cmc.2024.057392
Received 16 August 2024; Accepted 06 November 2024; Issue published 17 February 2025
Abstract
Current spatio-temporal action detection methods lack sufficient capabilities in extracting and comprehending spatio-temporal information. This paper introduces an end-to-end Adaptive Cross-Scale Fusion Encoder-Decoder (ACSF-ED) network to predict the action and locate the object efficiently. In the Adaptive Cross-Scale Fusion Spatio-Temporal Encoder (ACSF ST-Encoder), the Asymptotic Cross-scale Feature-fusion Module (ACCFM) is designed to address the issue of information degradation caused by the propagation of high-level semantic information, thereby extracting high-quality multi-scale features to provide superior features for subsequent spatio-temporal information modeling. Within the Shared-Head Decoder structure, a shared classification and regression detection head is constructed. A multi-constraint loss function composed of one-to-one, one-to-many, and contrastive denoising losses is designed to address the problem of insufficient constraint force in predicting results with traditional methods. This loss function enhances the accuracy of model classification predictions and improves the proximity of regression position predictions to ground truth objects. The proposed method model is evaluated on the popular dataset UCF101-24 and JHMDB-21. Experimental results demonstrate that the proposed method achieves an accuracy of 81.52% on the Frame-mAP metric, surpassing current existing methods.Keywords
Cite This Article
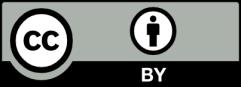
This work is licensed under a Creative Commons Attribution 4.0 International License , which permits unrestricted use, distribution, and reproduction in any medium, provided the original work is properly cited.