Open Access
ARTICLE
An Arrhythmia Intelligent Recognition Method Based on a Multimodal Information and Spatio-Temporal Hybrid Neural Network Model
1 School of Computer Science, Zhongyuan University of Technology, Zhengzhou, 450007, China
2 International Joint Laboratory for AI Interpretability and Reasoning Applications, Zhengzhou, 450007, China
3 School of Foreign Languages, Zhengzhou University of Economics and Business, Zhengzhou, 450099, China
* Corresponding Author: Runchuan Li. Email:
(This article belongs to the Special Issue: Emerging Trends and Applications of Deep Learning for Biomedical Signal and Image Processing)
Computers, Materials & Continua 2025, 82(2), 3443-3465. https://doi.org/10.32604/cmc.2024.059403
Received 07 October 2024; Accepted 26 November 2024; Issue published 17 February 2025
Abstract
Electrocardiogram (ECG) analysis is critical for detecting arrhythmias, but traditional methods struggle with large-scale Electrocardiogram data and rare arrhythmia events in imbalanced datasets. These methods fail to perform multi-perspective learning of temporal signals and Electrocardiogram images, nor can they fully extract the latent information within the data, falling short of the accuracy required by clinicians. Therefore, this paper proposes an innovative hybrid multimodal spatiotemporal neural network to address these challenges. The model employs a multimodal data augmentation framework integrating visual and signal-based features to enhance the classification performance of rare arrhythmias in imbalanced datasets. Additionally, the spatiotemporal fusion module incorporates a spatiotemporal graph convolutional network to jointly model temporal and spatial features, uncovering complex dependencies within the Electrocardiogram data and improving the model’s ability to represent complex patterns. In experiments conducted on the MIT-BIH arrhythmia dataset, the model achieved 99.95% accuracy, 99.80% recall, and a 99.78% F1 score. The model was further validated for generalization using the clinical INCART arrhythmia dataset, and the results demonstrated its effectiveness in terms of both generalization and robustness.Keywords
Cite This Article
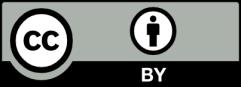
This work is licensed under a Creative Commons Attribution 4.0 International License , which permits unrestricted use, distribution, and reproduction in any medium, provided the original work is properly cited.