Open Access
ARTICLE
Vector Extraction from Design Drawings for Intelligent 3D Modeling of Transmission Towers
1 State Grid Jiangsu Electric Power Co., Ltd. Construction Branch, Nanjing, 210011, China
2 School of Automation, Southeast University, Nanjing, 210096, China
* Corresponding Author: Chenxing Wang. Email:
Computers, Materials & Continua 2025, 82(2), 2813-2829. https://doi.org/10.32604/cmc.2024.059094
Received 27 September 2024; Accepted 28 November 2024; Issue published 17 February 2025
Abstract
Accurate vector extraction from design drawings is required first to automatically create 3D models from pixel-level engineering design drawings. However, this task faces the challenges of complicated design shapes as well as cumbersome and cluttered annotations on drawings, which interfere with the vector extraction heavily. In this article, the transmission tower containing the most complex structure is taken as the research object, and a semantic segmentation network is constructed to first segment the shape masks from the pixel-level drawings. Preprocessing and postprocessing are also proposed to ensure the stability and accuracy of the shape mask segmentation. Then, based on the obtained shape masks, a vector extraction network guided by heatmaps is designed to extract structural vectors by fusing the features from node heatmap and skeleton heatmap, respectively. Compared with the state-of-the-art methods, experiment results illustrate that the proposed semantic segmentation method can effectively eliminate the interference of many elements on drawings to segment the shape masks effectively, meanwhile, the model trained by the proposed vector extraction network can accurately extract the vectors such as nodes and line connections, avoiding redundant vector detection. The proposed method lays a solid foundation for automatic 3D model reconstruction and contributes to technological advancements in relevant fields.Keywords
Cite This Article
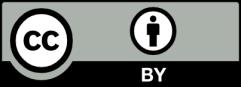
This work is licensed under a Creative Commons Attribution 4.0 International License , which permits unrestricted use, distribution, and reproduction in any medium, provided the original work is properly cited.