Open Access
ARTICLE
Dynamic Task Offloading Scheme for Edge Computing via Meta-Reinforcement Learning
1 Teacher Development and Teaching Evaluation Center, Civil Aviation Flight University of China, Guanghan, 618307, China
2 Institute of Electronics and Electrical Engineering, Civil Aviation Flight University of China, Guanghan, 618307, China
* Corresponding Author: Jiajia Liu. Email:
(This article belongs to the Special Issue: Edge-based IoT Systems with Cross-Designs of Communication, Computing, and Control)
Computers, Materials & Continua 2025, 82(2), 2609-2635. https://doi.org/10.32604/cmc.2024.058810
Received 21 September 2024; Accepted 22 November 2024; Issue published 17 February 2025
Abstract
As an important complement to cloud computing, edge computing can effectively reduce the workload of the backbone network. To reduce latency and energy consumption of edge computing, deep learning is used to learn the task offloading strategies by interacting with the entities. In actual application scenarios, users of edge computing are always changing dynamically. However, the existing task offloading strategies cannot be applied to such dynamic scenarios. To solve this problem, we propose a novel dynamic task offloading framework for distributed edge computing, leveraging the potential of meta-reinforcement learning (MRL). Our approach formulates a multi-objective optimization problem aimed at minimizing both delay and energy consumption. We model the task offloading strategy using a directed acyclic graph (DAG). Furthermore, we propose a distributed edge computing adaptive task offloading algorithm rooted in MRL. This algorithm integrates multiple Markov decision processes (MDP) with a sequence-to-sequence (seq2seq) network, enabling it to learn and adapt task offloading strategies responsively across diverse network environments. To achieve joint optimization of delay and energy consumption, we incorporate the non-dominated sorting genetic algorithm II (NSGA-II) into our framework. Simulation results demonstrate the superiority of our proposed solution, achieving a 21% reduction in time delay and a 19% decrease in energy consumption compared to alternative task offloading schemes. Moreover, our scheme exhibits remarkable adaptability, responding swiftly to changes in various network environments.Keywords
Cite This Article
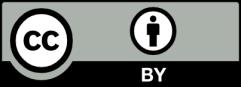
This work is licensed under a Creative Commons Attribution 4.0 International License , which permits unrestricted use, distribution, and reproduction in any medium, provided the original work is properly cited.