Open Access
ARTICLE
PIAFGNN: Property Inference Attacks against Federated Graph Neural Networks
1 College of Computer Science and Technology, Nanjing University of Aeronautics and Astronautics, Nanjing, 321002, China
2 Collaborative Innovation Center of Novel Software Technology and Industrialization, Nanjing, 210023, China
* Corresponding Author: Bing Chen. Email:
Computers, Materials & Continua 2025, 82(2), 1857-1877. https://doi.org/10.32604/cmc.2024.057814
Received 28 August 2024; Accepted 28 November 2024; Issue published 17 February 2025
Abstract
Federated Graph Neural Networks (FedGNNs) have achieved significant success in representation learning for graph data, enabling collaborative training among multiple parties without sharing their raw graph data and solving the data isolation problem faced by centralized GNNs in data-sensitive scenarios. Despite the plethora of prior work on inference attacks against centralized GNNs, the vulnerability of FedGNNs to inference attacks has not yet been widely explored. It is still unclear whether the privacy leakage risks of centralized GNNs will also be introduced in FedGNNs. To bridge this gap, we present PIAFGNN, the first property inference attack (PIA) against FedGNNs. Compared with prior works on centralized GNNs, in PIAFGNN, the attacker can only obtain the global embedding gradient distributed by the central server. The attacker converts the task of stealing the target user’s local embeddings into a regression problem, using a regression model to generate the target graph node embeddings. By training shadow models and property classifiers, the attacker can infer the basic property information within the target graph that is of interest. Experiments on three benchmark graph datasets demonstrate that PIAFGNN achieves attack accuracy of over 70% in most cases, even approaching the attack accuracy of inference attacks against centralized GNNs in some instances, which is much higher than the attack accuracy of the random guessing method. Furthermore, we observe that common defense mechanisms cannot mitigate our attack without affecting the model’s performance on mainly classification tasks.Keywords
Cite This Article
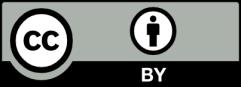
This work is licensed under a Creative Commons Attribution 4.0 International License , which permits unrestricted use, distribution, and reproduction in any medium, provided the original work is properly cited.