Open Access
ARTICLE
VTAN: A Novel Video Transformer Attention-Based Network for Dynamic Sign Language Recognition
1 School of Mathematics and Computer Science, Nanchang University, Nanchang, 330031, China
2 Institute of Metaverse, Nanchang University, Nanchang, 330031, China
3 Jiangxi Provincial Key Laboratory of Virtual Reality, Nanchang University, Nanchang, 330031, China
* Corresponding Author: Weidong Min. Email:
Computers, Materials & Continua 2025, 82(2), 2793-2812. https://doi.org/10.32604/cmc.2024.057456
Received 18 August 2024; Accepted 22 November 2024; Issue published 17 February 2025
Abstract
Dynamic sign language recognition holds significant importance, particularly with the application of deep learning to address its complexity. However, existing methods face several challenges. Firstly, recognizing dynamic sign language requires identifying keyframes that best represent the signs, and missing these keyframes reduces accuracy. Secondly, some methods do not focus enough on hand regions, which are small within the overall frame, leading to information loss. To address these challenges, we propose a novel Video Transformer Attention-based Network (VTAN) for dynamic sign language recognition. Our approach prioritizes informative frames and hand regions effectively. To tackle the first issue, we designed a keyframe extraction module enhanced by a convolutional autoencoder, which focuses on selecting information-rich frames and eliminating redundant ones from the video sequences. For the second issue, we developed a soft attention-based transformer module that emphasizes extracting features from hand regions, ensuring that the network pays more attention to hand information within sequences. This dual-focus approach improves effective dynamic sign language recognition by addressing the key challenges of identifying critical frames and emphasizing hand regions. Experimental results on two public benchmark datasets demonstrate the effectiveness of our network, outperforming most of the typical methods in sign language recognition tasks.Keywords
Cite This Article
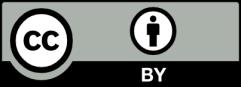
This work is licensed under a Creative Commons Attribution 4.0 International License , which permits unrestricted use, distribution, and reproduction in any medium, provided the original work is properly cited.