Open Access
ARTICLE
Retinexformer+: Retinex-Based Dual-Channel Transformer for Low-Light Image Enhancement
1 School of Information Engineering, Southwest University of Science and Technology, Mianyang, 621000, China
2 Criminal Investigation Department, Sichuan Police College, Luzhou, 646000, China
3 School of Electronics and Information, Mianyang Polytechnic, Mianyang, 621000, China
* Corresponding Author: Hongying Zhang. Email:
(This article belongs to the Special Issue: Data and Image Processing in Intelligent Information Systems)
Computers, Materials & Continua 2025, 82(2), 1969-1984. https://doi.org/10.32604/cmc.2024.057662
Received 24 August 2024; Accepted 30 October 2024; Issue published 17 February 2025
Abstract
Enhancing low-light images with color distortion and uneven multi-light source distribution presents challenges. Most advanced methods for low-light image enhancement are based on the Retinex model using deep learning. Retinexformer introduces channel self-attention mechanisms in the IG-MSA. However, it fails to effectively capture long-range spatial dependencies, leaving room for improvement. Based on the Retinexformer deep learning framework, we designed the Retinexformer+ network. The “+” signifies our advancements in extracting long-range spatial dependencies. We introduced multi-scale dilated convolutions in illumination estimation to expand the receptive field. These convolutions effectively capture the weakening semantic dependency between pixels as distance increases. In illumination restoration, we used Unet++ with multi-level skip connections to better integrate semantic information at different scales. The designed Illumination Fusion Dual Self-Attention (IF-DSA) module embeds multi-scale dilated convolutions to achieve spatial self-attention. This module captures long-range spatial semantic relationships within acceptable computational complexity. Experimental results on the Low-Light (LOL) dataset show that Retexformer+ outperforms other State-Of-The-Art (SOTA) methods in both quantitative and qualitative evaluations, with the computational complexity increased to an acceptable 51.63 G FLOPS. On the LOL_v1 dataset, RetinexFormer+ shows an increase of 1.15 in Peak Signal-to-Noise Ratio (PSNR) and a decrease of 0.39 in Root Mean Square Error (RMSE). On the LOL_v2_real dataset, the PSNR increases by 0.42 and the RMSE decreases by 0.18. Experimental results on the Exdark dataset show that Retexformer+ can effectively enhance real-scene images and maintain their semantic information.Keywords
Cite This Article
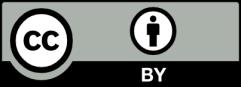
This work is licensed under a Creative Commons Attribution 4.0 International License , which permits unrestricted use, distribution, and reproduction in any medium, provided the original work is properly cited.