Open Access
ARTICLE
Unsupervised Low-Light Image Enhancement Based on Explicit Denoising and Knowledge Distillation
1 College of Computer Science and Engineering, Guilin University of Technology, Guilin, 541006, China
2 Guangxi Key Laboratory of Embedded Technology and Intelligent System, Guilin University of Technology, Guilin, 541004, China
* Corresponding Author: Shuiwang Li. Email:
(This article belongs to the Special Issue: Computer Vision and Image Processing: Feature Selection, Image Enhancement and Recognition)
Computers, Materials & Continua 2025, 82(2), 2537-2554. https://doi.org/10.32604/cmc.2024.059000
Received 25 September 2024; Accepted 14 November 2024; Issue published 17 February 2025
Abstract
Under low-illumination conditions, the quality of image signals deteriorates significantly, typically characterized by a peak signal-to-noise ratio (PSNR) below 10 dB, which severely limits the usability of the images. Supervised methods, which utilize paired high-low light images as training sets, can enhance the PSNR to around 20 dB, significantly improving image quality. However, such data is challenging to obtain. In recent years, unsupervised low-light image enhancement (LIE) methods based on the Retinex framework have been proposed, but they generally lag behind supervised methods by 5–10 dB in performance. In this paper, we introduce the Denoising-Distilled Retine (DDR) method, an unsupervised approach that integrates denoising priors into a Retinex-based training framework. By explicitly incorporating denoising, the DDR method effectively addresses the challenges of noise and artifacts in low-light images, thereby enhancing the performance of the Retinex framework. The model achieved a PSNR of 19.82 dB on the LOL dataset, which is comparable to the performance of supervised methods. Furthermore, by applying knowledge distillation, the DDR method optimizes the model for real-time processing of low-light images, achieving a processing speed of 199.7 fps without incurring additional computational costs. While the DDR method has demonstrated superior performance in terms of image quality and processing speed, there is still room for improvement in terms of robustness across different color spaces and under highly resource-constrained conditions. Future research will focus on enhancing the model’s generalizability and adaptability to address these challenges. Our rigorous testing on public datasets further substantiates the DDR method’s state-of-the-art performance in both image quality and processing speed.Keywords
Cite This Article
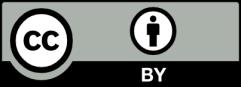
This work is licensed under a Creative Commons Attribution 4.0 International License , which permits unrestricted use, distribution, and reproduction in any medium, provided the original work is properly cited.