Open Access
ARTICLE
YOLO-LFD: A Lightweight and Fast Model for Forest Fire Detection
1 School of Artificial Intelligence, Nanjing University of Information Science and Technology, Nanjing, 210044, China
2 School of Computer Science, Nanjing University of Information Science and Technology, Nanjing, 210044, China
3 Electrical & Computer Engineering, University of Illinois at Urbana-Champaign, Urbana, IL 61801, USA
* Corresponding Author: Yangyang Zhang. Email:
(This article belongs to the Special Issue: Research on Deep Learning-based Object Detection and Its Derivative Key Technologies)
Computers, Materials & Continua 2025, 82(2), 3399-3417. https://doi.org/10.32604/cmc.2024.058932
Received 24 September 2024; Accepted 26 November 2024; Issue published 17 February 2025
Abstract
Forest fires pose a serious threat to ecological balance, air quality, and the safety of both humans and wildlife. This paper presents an improved model based on You Only Look Once version 5 (YOLOv5), named YOLO Lightweight Fire Detector (YOLO-LFD), to address the limitations of traditional sensor-based fire detection methods in terms of real-time performance and accuracy. The proposed model is designed to enhance inference speed while maintaining high detection accuracy on resource-constrained devices such as drones and embedded systems. Firstly, we introduce Depthwise Separable Convolutions (DSConv) to reduce the complexity of the feature extraction network. Secondly, we design and implement the Lightweight Faster Implementation of Cross Stage Partial (CSP) Bottleneck with 2 Convolutions (C2f-Light) and the CSP Structure with 3 Compact Inverted Blocks (C3CIB) modules to replace the traditional C3 modules. This optimization enhances deep feature extraction and semantic information processing, thereby significantly increasing inference speed. To enhance the detection capability for small fires, the model employs a Normalized Wasserstein Distance (NWD) loss function, which effectively reduces the missed detection rate and improves the accuracy of detecting small fire sources. Experimental results demonstrate that compared to the baseline YOLOv5s model, the YOLO-LFD model not only increases inference speed by 19.3% but also significantly improves the detection accuracy for small fire targets, with only a 1.6% reduction in overall mean average precision (mAP)@0.5. Through these innovative improvements to YOLOv5s, the YOLO-LFD model achieves a balance between speed and accuracy, making it particularly suitable for real-time detection tasks on mobile and embedded devices.Keywords
Cite This Article
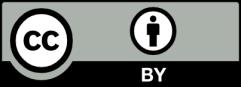
This work is licensed under a Creative Commons Attribution 4.0 International License , which permits unrestricted use, distribution, and reproduction in any medium, provided the original work is properly cited.