Open Access
ARTICLE
Multi-Scale Feature Fusion Network Model for Wireless Capsule Endoscopic Intestinal Lesion Detection
School of Computer and Artificial Intelligence, Changzhou University, Changzhou, 213164, China
* Corresponding Author: Hui Wang. Email:
(This article belongs to the Special Issue: Medical Imaging Based Disease Diagnosis Using AI)
Computers, Materials & Continua 2025, 82(2), 2415-2429. https://doi.org/10.32604/cmc.2024.058250
Received 08 September 2024; Accepted 19 November 2024; Issue published 17 February 2025
Abstract
WCE (Wireless Capsule Endoscopy) is a new technology that combines computer vision and medicine, allowing doctors to visualize the conditions inside the intestines, achieving good diagnostic results. However, due to the complex intestinal environment and limited pixel resolution of WCE videos, lesions are not easily detectable, and it takes an experienced doctor 1–2 h to analyze a complete WCE video. The use of computer-aided diagnostic methods, assisting or even replacing manual WCE diagnosis, has significant application value. In response to the issue of intestinal lesion detection in WCE videos, this paper proposes a multi-scale feature fusion network model TSD-YOLO based on the YOLO (You Only Look Once) architecture: (I) a Tiny Detection Layer to avoid the loss of shallow feature information for tiny-scale targets; (II) integrating a simple, parameter-free attention module (SimAM) at the neck to better extract local lesion features and fuse features; (III) incorporating a new loss function DIoU (Distance Intersection over Union) to better achieve boundary box regression for target detection. This model was validated using the WCE dataset from Kyushu University Hospital. For the dataset containing 18,000 images, the evaluation metrics of our model for 12 types of lesions, outperformed existing reported results from advanced models on this dataset, and the mAP (mean Average Precision) and precision evaluation metrics improved by 3.7% and 0.9% over the benchmark model.Keywords
Cite This Article
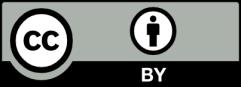
This work is licensed under a Creative Commons Attribution 4.0 International License , which permits unrestricted use, distribution, and reproduction in any medium, provided the original work is properly cited.