Open Access
ARTICLE
MSSTGCN: Multi-Head Self-Attention and Spatial-Temporal Graph Convolutional Network for Multi-Scale Traffic Flow Prediction
School of Computer Science, Hubei University of Technology, Wuhan, 430068, China
* Corresponding Author: Xinlu Zong. Email:
(This article belongs to the Special Issue: Graph Neural Networks: Methods and Applications in Graph-related Problems)
Computers, Materials & Continua 2025, 82(2), 3517-3537. https://doi.org/10.32604/cmc.2024.057494
Received 19 August 2024; Accepted 07 November 2024; Issue published 17 February 2025
Abstract
Accurate traffic flow prediction has a profound impact on modern traffic management. Traffic flow has complex spatial-temporal correlations and periodicity, which poses difficulties for precise prediction. To address this problem, a Multi-head Self-attention and Spatial-Temporal Graph Convolutional Network (MSSTGCN) for multiscale traffic flow prediction is proposed. Firstly, to capture the hidden traffic periodicity of traffic flow, traffic flow is divided into three kinds of periods, including hourly, daily, and weekly data. Secondly, a graph attention residual layer is constructed to learn the global spatial features across regions. Local spatial-temporal dependence is captured by using a T-GCN module. Thirdly, a transformer layer is introduced to learn the long-term dependence in time. A position embedding mechanism is introduced to label position information for all traffic sequences. Thus, this multi-head self-attention mechanism can recognize the sequence order and allocate weights for different time nodes. Experimental results on four real-world datasets show that the MSSTGCN performs better than the baseline methods and can be successfully adapted to traffic prediction tasks.Keywords
Cite This Article
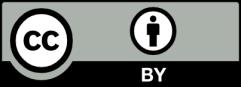
This work is licensed under a Creative Commons Attribution 4.0 International License , which permits unrestricted use, distribution, and reproduction in any medium, provided the original work is properly cited.