Open Access
ARTICLE
Multi-Head Attention Enhanced Parallel Dilated Convolution and Residual Learning for Network Traffic Anomaly Detection
1 College of Computer Engineering, Jimei University, Xiamen, 361021, China
2 Xiamen Jikuai Technology Co., Ltd., Xiamen, 361000, China
* Corresponding Author: Jian Mao. Email:
(This article belongs to the Special Issue: Fortifying the Foundations: Novel Approaches to Cyber-Physical Systems Intrusion Detection and Industrial 4.0 Security)
Computers, Materials & Continua 2025, 82(2), 2159-2176. https://doi.org/10.32604/cmc.2024.058396
Received 11 September 2024; Accepted 08 November 2024; Issue published 17 February 2025
Abstract
Abnormal network traffic, as a frequent security risk, requires a series of techniques to categorize and detect it. Existing network traffic anomaly detection still faces challenges: the inability to fully extract local and global features, as well as the lack of effective mechanisms to capture complex interactions between features; Additionally, when increasing the receptive field to obtain deeper feature representations, the reliance on increasing network depth leads to a significant increase in computational resource consumption, affecting the efficiency and performance of detection. Based on these issues, firstly, this paper proposes a network traffic anomaly detection model based on parallel dilated convolution and residual learning (Res-PDC). To better explore the interactive relationships between features, the traffic samples are converted into two-dimensional matrix. A module combining parallel dilated convolutions and residual learning (res-pdc) was designed to extract local and global features of traffic at different scales. By utilizing res-pdc modules with different dilation rates, we can effectively capture spatial features at different scales and explore feature dependencies spanning wider regions without increasing computational resources. Secondly, to focus and integrate the information in different feature subspaces, further enhance and extract the interactions among the features, multi-head attention is added to Res-PDC, resulting in the final model: multi-head attention enhanced parallel dilated convolution and residual learning (MHA-Res-PDC) for network traffic anomaly detection. Finally, comparisons with other machine learning and deep learning algorithms are conducted on the NSL-KDD and CIC-IDS-2018 datasets. The experimental results demonstrate that the proposed method in this paper can effectively improve the detection performance.Keywords
Cite This Article
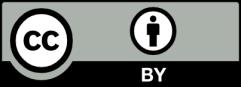
This work is licensed under a Creative Commons Attribution 4.0 International License , which permits unrestricted use, distribution, and reproduction in any medium, provided the original work is properly cited.