Open Access
ARTICLE
Lip-Audio Modality Fusion for Deep Forgery Video Detection
1 College of Cyberspace Security, Information Engineering University, Zhengzhou, 450001, China
2 Research Institute of Intelligent Networks, Zhejiang Lab, Hangzhou, 311121, China
3 College of Computer Science and Technology, Zhejiang University, Hangzhou, 310027, China
4 Henan Key Laboratory of Cyberspace Situation Awareness, Zhengzhou, 450001, China
5 Key Laboratory of Cyberspace Security, Ministry of Education, Zhengzhou, 450001, China
* Corresponding Author: Zhiyu Wang. Email:
(This article belongs to the Special Issue: Multimedia Security in Deep Learning)
Computers, Materials & Continua 2025, 82(2), 3499-3515. https://doi.org/10.32604/cmc.2024.057859
Received 25 August 2024; Accepted 11 November 2024; Issue published 17 February 2025
Abstract
In response to the problem of traditional methods ignoring audio modality tampering, this study aims to explore an effective deep forgery video detection technique that improves detection precision and reliability by fusing lip images and audio signals. The main method used is lip-audio matching detection technology based on the Siamese neural network, combined with MFCC (Mel Frequency Cepstrum Coefficient) feature extraction of band-pass filters, an improved dual-branch Siamese network structure, and a two-stream network structure design. Firstly, the video stream is preprocessed to extract lip images, and the audio stream is preprocessed to extract MFCC features. Then, these features are processed separately through the two branches of the Siamese network. Finally, the model is trained and optimized through fully connected layers and loss functions. The experimental results show that the testing accuracy of the model in this study on the LRW (Lip Reading in the Wild) dataset reaches 92.3%; the recall rate is 94.3%; the F1 score is 93.3%, significantly better than the results of CNN (Convolutional Neural Networks) and LSTM (Long Short-Term Memory) models. In the validation of multi-resolution image streams, the highest accuracy of dual-resolution image streams reaches 94%. Band-pass filters can effectively improve the signal-to-noise ratio of deep forgery video detection when processing different types of audio signals. The real-time processing performance of the model is also excellent, and it achieves an average score of up to 5 in user research. These data demonstrate that the method proposed in this study can effectively fuse visual and audio information in deep forgery video detection, accurately identify inconsistencies between video and audio, and thus verify the effectiveness of lip-audio modality fusion technology in improving detection performance.Keywords
Cite This Article
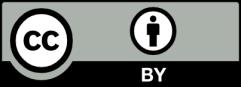
This work is licensed under a Creative Commons Attribution 4.0 International License , which permits unrestricted use, distribution, and reproduction in any medium, provided the original work is properly cited.