Open Access
ARTICLE
APWF: A Parallel Website Fingerprinting Attack with Attention Mechanism
1 College of Computer Science and Technology, Changchun University, Changchun, 130022, China
2 School of Cyberspace Science and Technology, Beijing Institute of Technology, Beijing, 100081, China
3 Key Laboratory of Intelligent Rehabilitation and Barrier-Free for the Disabled, Changchun University, Changchun, 130022, China
4 China Academy of Information and Communications Technology, Beijing, 100083, China
5 Key Laboratory of Mobile Application Innovation and Governance Technology, Ministry of Industry and Information Technology, Beijing, 100846, China
* Corresponding Author: Yi Wu. Email:
Computers, Materials & Continua 2025, 82(2), 2027-2041. https://doi.org/10.32604/cmc.2024.058178
Received 06 September 2024; Accepted 07 November 2024; Issue published 17 February 2025
Abstract
Website fingerprinting (WF) attacks can reveal information about the websites users browse by de-anonymizing encrypted traffic. Traditional website fingerprinting attack models, focusing solely on a single spatial feature, are inefficient regarding training time. When confronted with the concept drift problem, they suffer from a sharp drop in attack accuracy within a short period due to their reliance on extensive, outdated training data. To address the above problems, this paper proposes a parallel website fingerprinting attack (APWF) that incorporates an attention mechanism, which consists of an attack model and a fine-tuning method. Among them, the APWF model innovatively adopts a parallel structure, fusing temporal features related to both the front and back of the fingerprint sequence, along with spatial features captured through channel attention enhancement, to enhance the accuracy of the attack. Meanwhile, the APWF method introduces isomorphic migration learning and adjusts the model by freezing the optimal model weights and fine-tuning the parameters so that only a small number of the target, samples are needed to adapt to web page changes. A series of experiments show that the attack model can achieve 83% accuracy with the help of only 10 samples per category, which is a 30% improvement over the traditional attack model. Compared to comparative modeling, APWF improves accuracy while reducing time costs. After further fine-tuning the freezing model, the method in this paper can maintain the accuracy at 92.4% in the scenario of 56 days between the training data and the target data, which is only 4% less loss compared to the instant attack, significantly improving the robustness and accuracy of the model in coping with conceptual drift.Keywords
Cite This Article
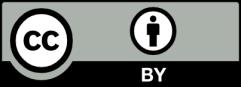
This work is licensed under a Creative Commons Attribution 4.0 International License , which permits unrestricted use, distribution, and reproduction in any medium, provided the original work is properly cited.