Open Access
ARTICLE
Masked Face Restoration Model Based on Lightweight GAN
College of Information and Cyber Security, People’s Public Security University of China, Beijing, 100038, China
* Corresponding Author: Tianliang Lu. Email:
Computers, Materials & Continua 2025, 82(2), 3591-3608. https://doi.org/10.32604/cmc.2024.057554
Received 21 August 2024; Accepted 05 November 2024; Issue published 17 February 2025
Abstract
Facial recognition systems have become increasingly significant in public security efforts. They play a crucial role in apprehending criminals and locating missing children and elderly individuals. Nevertheless, in practical applications, around 30% to 50% of images are obstructed to varied extents, for as by the presence of masks, glasses, or hats. Repairing the masked facial images will enhance face recognition accuracy by 10% to 20%. Simultaneously, market research indicates a rising demand for efficient facial recognition technology within the security and surveillance sectors, with projections suggesting that the global facial recognition market would exceed US$3 billion by 2025. Therefore, finding a prompt and efficient solution to fix the masked face and enhance its accuracy has become a pressing issue that has to be resolved. Currently, the generative adversarial network has shown excellent performance in the field of image restoration, with high precision and good quality of restoration results, but it consumes a lot of computing resources. Based on this, this paper proposes a model architecture that uses the U-Net network to replace the generator in the generative adversarial network, and replaces all traditional convolutional layers with Depthwise Separable Convolutional (DWSC) to make the entire network lightweight. Ultimately, We utilise the Peak Signal-to-Noise Ratio (PSNR) value to assess the efficacy of the developed model. We select samples with occlusion levels ranging from 10%–15% and 20%–30%, yielding PSNR values of 35.51 and 30.33, respectively. In contrast, the PSNR values of the three predominant algorithms in image restoration—PM, ShiftNet, and PICNet—are all below 30, demonstrating the superiority of the model presented in this paper. However, the model presented in this work possesses certain drawbacks. This work employs solely black rectangles to replicate real-life occlusions. Future study should utilise tangible objects, like as sunglasses and masks, to directly imitate occlusions, so enhancing the accuracy of the restoration effect. The model presented in this study can be further expanded from image restoration to video restoration to investigate the potential for dynamic occlusion repair.Keywords
Cite This Article
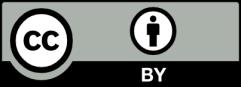
This work is licensed under a Creative Commons Attribution 4.0 International License , which permits unrestricted use, distribution, and reproduction in any medium, provided the original work is properly cited.