Open Access
ARTICLE
Context-Aware Feature Extraction Network for High-Precision UAV-Based Vehicle Detection in Urban Environments
1 Department of Electrical Engineering, College of Engineering, Northern Border University, Arar, 91431, Saudi Arabia
2 Department of Civil Engineering, College of Engineering, Northern Border University, Arar, 91431, Saudi Arabia
3 Faculty of Computing and Information Technology, Northern Border University, Rafha, 91911, Saudi Arabia
4 College of Science, Northern Border University, Arar, 91431, Saudi Arabia
* Corresponding Author: Yahia Said. Email:
(This article belongs to the Special Issue: The Next-generation Deep Learning Approaches to Emerging Real-world Applications)
Computers, Materials & Continua 2024, 81(3), 4349-4370. https://doi.org/10.32604/cmc.2024.058903
Received 23 September 2024; Accepted 07 November 2024; Issue published 19 December 2024
Abstract
The integration of Unmanned Aerial Vehicles (UAVs) into Intelligent Transportation Systems (ITS) holds transformative potential for real-time traffic monitoring, a critical component of emerging smart city infrastructure. UAVs offer unique advantages over stationary traffic cameras, including greater flexibility in monitoring large and dynamic urban areas. However, detecting small, densely packed vehicles in UAV imagery remains a significant challenge due to occlusion, variations in lighting, and the complexity of urban landscapes. Conventional models often struggle with these issues, leading to inaccurate detections and reduced performance in practical applications. To address these challenges, this paper introduces CFEMNet, an advanced deep learning model specifically designed for high-precision vehicle detection in complex urban environments. CFEMNet is built on the High-Resolution Network (HRNet) architecture and integrates a Context-aware Feature Extraction Module (CFEM), which combines multi-scale feature learning with a novel Self-Attention and Convolution layer setup within a Multi-scale Feature Block (MFB). This combination allows CFEMNet to accurately capture fine-grained details across varying scales, crucial for detecting small or partially occluded vehicles. Furthermore, the model incorporates an Equivalent Feed-Forward Network (EFFN) Block to ensure robust extraction of both spatial and semantic features, enhancing its ability to distinguish vehicles from similar objects. To optimize computational efficiency, CFEMNet employs a local window adaptation of Multi-head Self-Attention (MSA), which reduces memory overhead without sacrificing detection accuracy. Extensive experimental evaluations on the UAVDT and VisDrone-DET2018 datasets confirm CFEMNet’s superior performance in vehicle detection compared to existing models. This new architecture establishes CFEMNet as a benchmark for UAV-enabled traffic management, offering enhanced precision, reduced computational demands, and scalability for deployment in smart city applications. The advancements presented in CFEMNet contribute significantly to the evolution of smart city technologies, providing a foundation for intelligent and responsive traffic management systems that can adapt to the dynamic demands of urban environments.Keywords
Cite This Article
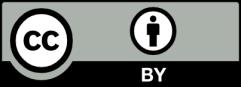
This work is licensed under a Creative Commons Attribution 4.0 International License , which permits unrestricted use, distribution, and reproduction in any medium, provided the original work is properly cited.