Open Access
ARTICLE
Malicious Document Detection Based on GGE Visualization
Henan Province Key Laboratory of Information Security, Information Engineering University, Zhengzhou, 450000, China
* Corresponding Author: Yi Sun. Email:
Computers, Materials & Continua 2025, 82(1), 1233-1254. https://doi.org/10.32604/cmc.2024.057710
Received 26 August 2024; Accepted 30 October 2024; Issue published 03 January 2025
Abstract
With the development of anti-virus technology, malicious documents have gradually become the main pathway of Advanced Persistent Threat (APT) attacks, therefore, the development of effective malicious document classifiers has become particularly urgent. Currently, detection methods based on document structure and behavioral features encounter challenges in feature engineering, these methods not only have limited accuracy, but also consume large resources, and usually can only detect documents in specific formats, which lacks versatility and adaptability. To address such problems, this paper proposes a novel malicious document detection method-visualizing documents as GGE images (Grayscale, Grayscale matrix, Entropy). The GGE method visualizes the original byte sequence of the malicious document as a grayscale image, the information entropy sequence of the document as an entropy image, and at the same time, the grayscale level co-occurrence matrix and the texture and spatial information stored in it are converted into grayscale matrix image, and fuses the three types of images to get the GGE color image. The Convolutional Block Attention Module-EfficientNet-B0 (CBAM-EfficientNet-B0) model is then used for classification, combining transfer learning and applying the pre-trained model on the ImageNet dataset to the feature extraction process of GGE images. As shown in the experimental results, the GGE method has superior performance compared with other methods, which is suitable for detecting malicious documents in different formats, and achieves an accuracy of 99.44% and 97.39% on Portable Document Format (PDF) and office datasets, respectively, and consumes less time during the detection process, which can be effectively applied to the task of detecting malicious documents in real-time.Keywords
Cite This Article
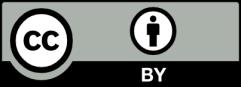
This work is licensed under a Creative Commons Attribution 4.0 International License , which permits unrestricted use, distribution, and reproduction in any medium, provided the original work is properly cited.