Open Access
ARTICLE
XGBoost-Based Power Grid Fault Prediction with Feature Enhancement: Application to Meteorology
1 State Grid Jiangsu Electric Power Co., Ltd., Information & Telecommunication Branch, Nanjing, 210024, China
2 State Grid Jiangsu Electric Power Co., Ltd., Information & Yangzhou Power Supply Branch, Yangzhou, 225000, China
3 School of Information Engineering, Yangzhou University, Yangzhou, 225127, China
* Corresponding Authors: Chen Zhang. Email: ; Junwu Zhu. Email:
Computers, Materials & Continua 2025, 82(2), 2893-2908. https://doi.org/10.32604/cmc.2024.057074
Received 07 August 2024; Accepted 30 October 2024; Issue published 17 February 2025
Abstract
The prediction of power grid faults based on meteorological factors is of great significance to reduce economic losses caused by power grid faults. However, the existing methods fail to effectively extract key features and accurately predict fault types due to the complexity of meteorological factors and their nonlinear relationships. In response to these challenges, we propose the Feature-Enhanced XGBoost power grid fault prediction method (FE-XGBoost). Specifically, we first combine the gradient boosting decision tree and recursive feature elimination method to extract essential features from meteorological data. Then, we incorporate a piecewise linear chaotic map to enhance the optimization accuracy of the sparrow search algorithm. Finally, we construct an XGBoost-based model for the classification prediction of power grid meteorological faults and optimize the hyperparameters such as the optimal tree depth, optimal learning rate, and optimal number of iterations using an enhanced sparrow search algorithm. Experimental results demonstrate that our method outperforms the baseline models in predicting power grid faults accurately.Keywords
Cite This Article
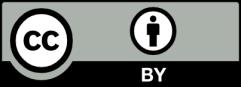
This work is licensed under a Creative Commons Attribution 4.0 International License , which permits unrestricted use, distribution, and reproduction in any medium, provided the original work is properly cited.