Open Access
ARTICLE
MixerKT: A Knowledge Tracing Model Based on Pure MLP Architecture
1 Key Laboratory of Education Informatization for Nationalities, Ministry of Education, Yunnan Normal University, Kunming, 650000, China
2 Yunnan Key Laboratory of Smart Education, Yunnan Normal University, Kunming, 650000, China
* Corresponding Author: Shu Zhang. Email:
Computers, Materials & Continua 2025, 82(1), 485-498. https://doi.org/10.32604/cmc.2024.057224
Received 11 August 2024; Accepted 07 October 2024; Issue published 03 January 2025
Abstract
In the field of intelligent education, the integration of artificial intelligence, especially deep learning technologies, has garnered significant attention. Knowledge tracing (KT) plays a pivotal role in this field by predicting students’ future performance through the analysis of historical interaction data, thereby assisting educators in evaluating knowledge mastery and tailoring instructional strategies. Traditional knowledge tracing methods, largely based on Recurrent Neural Networks (RNNs) and Transformer models, primarily focus on capturing long-term interaction patterns in sequential data. However, these models may neglect crucial short-term dynamics and other relevant features. This paper introduces a novel approach to knowledge tracing by leveraging a pure Multilayer Perceptron (MLP) architecture. We propose MixerKT, a knowledge tracing model based on the HyperMixer framework, which uniquely integrates global and local Mixer feature extractors. This architecture enables more effective extraction of both long-term interaction trends and recent learning behaviors, addressing limitations in current models that may overlook these key aspects. Empirical evaluations on two widely-used datasets, ASSISTments2009 and Algebra2005, demonstrate that MixerKT consistently outperforms several state-of-the-art models, including DKT, SAKT, and Separated Self-Attentive Neural Knowledge Tracing (SAINT). Specifically, MixerKT achieves higher prediction accuracy, highlighting its effectiveness in capturing the nuances of learners’ knowledge states. These results indicate that our model provides a more comprehensive representation of student learning patterns, enhancing the ability to predict future performance with greater precision.Keywords
Cite This Article
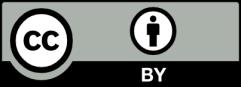
This work is licensed under a Creative Commons Attribution 4.0 International License , which permits unrestricted use, distribution, and reproduction in any medium, provided the original work is properly cited.