Open Access
ARTICLE
PD-YOLO: Colon Polyp Detection Model Based on Enhanced Small-Target Feature Extraction
1 New Engineering Industry College, Putian University, Putian, 351100, China
2 Engineering Research Center of Big Data Application in Private Health Medicine (Putian University), Fujian Province University, Putian, 351100, China
3 Computer College, Guangdong University of Science & Technology, Dongguan, 523668, China
* Corresponding Author: Rong-Guei Tsai. Email:
Computers, Materials & Continua 2025, 82(1), 913-928. https://doi.org/10.32604/cmc.2024.058467
Received 12 September 2024; Accepted 24 October 2024; Issue published 03 January 2025
Abstract
In recent years, the number of patients with colon disease has increased significantly. Colon polyps are the precursor lesions of colon cancer. If not diagnosed in time, they can easily develop into colon cancer, posing a serious threat to patients’ lives and health. A colonoscopy is an important means of detecting colon polyps. However, in polyp imaging, due to the large differences and diverse types of polyps in size, shape, color, etc., traditional detection methods face the problem of high false positive rates, which creates problems for doctors during the diagnosis process. In order to improve the accuracy and efficiency of colon polyp detection, this question proposes a network model suitable for colon polyp detection (PD-YOLO). This method introduces the self-attention mechanism CBAM (Convolutional Block Attention Module) in the backbone layer based on YOLOv7, allowing the model to adaptively focus on key information and ignore the unimportant parts. To help the model do a better job of polyp localization and bounding box regression, add the SPD-Conv (Symmetric Positive Definite Convolution) module to the neck layer and use deconvolution instead of upsampling. The experimental results indicate that the PD-YOLO algorithm demonstrates strong robustness in colon polyp detection. Compared to the original YOLOv7, on the Kvasir-SEG dataset, PD-YOLO has shown an increase of 5.44 percentage points in AP@0.5, showcasing significant advantages over other mainstream methods.Keywords
Cite This Article
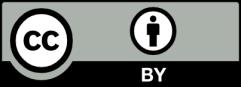
This work is licensed under a Creative Commons Attribution 4.0 International License , which permits unrestricted use, distribution, and reproduction in any medium, provided the original work is properly cited.