Open Access
ARTICLE
Image Captioning Using Multimodal Deep Learning Approach
1 Institut Supérieur de l'Electronique et du Numérique Méditerranée, ISEN Méditerranée, Toulon, 83000, France
2 College of Applied Engineering, Muzahimiyah Branch, King Saud University, Riyadh, 11421, Saudi Arabia
* Corresponding Author: Rihem Farkh. Email:
(This article belongs to the Special Issue: Metaheuristics, Soft Computing, and Machine Learning in Image Processing and Computer Vision)
Computers, Materials & Continua 2024, 81(3), 3951-3968. https://doi.org/10.32604/cmc.2024.053245
Received 28 April 2024; Accepted 01 August 2024; Issue published 19 December 2024
Abstract
The process of generating descriptive captions for images has witnessed significant advancements in last years, owing to the progress in deep learning techniques. Despite significant advancements, the task of thoroughly grasping image content and producing coherent, contextually relevant captions continues to pose a substantial challenge. In this paper, we introduce a novel multimodal method for image captioning by integrating three powerful deep learning architectures: YOLOv8 (You Only Look Once) for robust object detection, EfficientNetB7 for efficient feature extraction, and Transformers for effective sequence modeling. Our proposed model combines the strengths of YOLOv8 in detecting objects, the superior feature representation capabilities of EfficientNetB7, and the contextual understanding and sequential generation abilities of Transformers. We conduct extensive experiments on standard benchmark datasets to evaluate the effectiveness of our approach, demonstrating its ability to generate informative and semantically rich captions for diverse images. The experimental results showcase the synergistic benefits of integrating YOLOv8, EfficientNetB7, and Transformers in advancing the state-of-the-art in image captioning tasks. The proposed multimodal approach has yielded impressive outcomes, generating informative and semantically rich captions for a diverse range of images. By combining the strengths of YOLOv8, EfficientNetB7, and Transformers, the model has achieved state-of-the-art results in image captioning tasks. The significance of this approach lies in its ability to address the challenging task of generating coherent and contextually relevant captions while achieving a comprehensive understanding of image content. The integration of three powerful deep learning architectures demonstrates the synergistic benefits of multimodal fusion in advancing the state-of-the-art in image captioning. Furthermore, this approach has a profound impact on the field, opening up new avenues for research in multimodal deep learning and paving the way for more sophisticated and context-aware image captioning systems. These systems have the potential to make significant contributions to various fields, encompassing human-computer interaction, computer vision and natural language processing.Keywords
Cite This Article
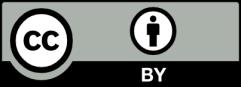
This work is licensed under a Creative Commons Attribution 4.0 International License , which permits unrestricted use, distribution, and reproduction in any medium, provided the original work is properly cited.