Open Access
ARTICLE
Stochastic Augmented-Based Dual-Teaching for Semi-Supervised Medical Image Segmentation
1 School of Computer Science and Engineering, Chongqing University of Technology, Chongqing, 400054, China
2 College of Artificial Intelligence, Chongqing Technology and Business University, Chongqing, 400067, China
* Corresponding Author: Yang Yuan. Email:
(This article belongs to the Special Issue: Deep Learning in Computer-Aided Diagnosis Based on Medical Image)
Computers, Materials & Continua 2025, 82(1), 543-560. https://doi.org/10.32604/cmc.2024.056478
Received 23 July 2024; Accepted 06 October 2024; Issue published 03 January 2025
Abstract
Existing semi-supervised medical image segmentation algorithms use copy-paste data augmentation to correct the labeled-unlabeled data distribution mismatch. However, current copy-paste methods have three limitations: (1) training the model solely with copy-paste mixed pictures from labeled and unlabeled input loses a lot of labeled information; (2) low-quality pseudo-labels can cause confirmation bias in pseudo-supervised learning on unlabeled data; (3) the segmentation performance in low-contrast and local regions is less than optimal. We design a Stochastic Augmentation-Based Dual-Teaching Auxiliary Training Strategy (SADT), which enhances feature diversity and learns high-quality features to overcome these problems. To be more precise, SADT trains the Student Network by using pseudo-label-based training from Teacher Network 1 and supervised learning with labeled data, which prevents the loss of rare labeled data. We introduce a bi-directional copy-paste mask with progressive high-entropy filtering to reduce data distribution disparities and mitigate confirmation bias in pseudo-supervision. For the mixed images, Deep-Shallow Spatial Contrastive Learning (DSSCL) is proposed in the feature spaces of Teacher Network 2 and the Student Network to improve the segmentation capabilities in low-contrast and local areas. In this procedure, the features retrieved by the Student Network are subjected to a random feature perturbation technique. On two openly available datasets, extensive trials show that our proposed SADT performs much better than the state-of-the-art semi-supervised medical segmentation techniques. Using only 10% of the labeled data for training, SADT was able to acquire a Dice score of 90.10% on the ACDC (Automatic Cardiac Diagnosis Challenge) dataset.Keywords
Cite This Article
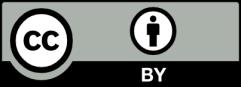
This work is licensed under a Creative Commons Attribution 4.0 International License , which permits unrestricted use, distribution, and reproduction in any medium, provided the original work is properly cited.