Open Access
ARTICLE
Computational Approach for Automated Segmentation and Classification of Region of Interest in Lateral Breast Thermograms
1 Department of Electrical, Computer and Telecommunications Engineering, Botswana International University of Science and Technology, Palapye, Private Bag 16, Botswana
2 College of Computer and Information Sciences, Prince Sultan University, Riyadh, 11586, Saudi Arabia
3 Robotics and Internet of Things Lab, Prince Sultan University, Riyadh, 11586, Saudi Arabia
* Corresponding Authors: Dennies Tsietso. Email: ; Muhammad Babar. Email:
(This article belongs to the Special Issue: Privacy-Aware AI-based Models for Cancer Diagnosis)
Computers, Materials & Continua 2024, 80(3), 4749-4765. https://doi.org/10.32604/cmc.2024.052793
Received 15 April 2024; Accepted 02 August 2024; Issue published 12 September 2024
Abstract
Breast cancer is one of the major health issues with high mortality rates and a substantial impact on patients and healthcare systems worldwide. Various Computer-Aided Diagnosis (CAD) tools, based on breast thermograms, have been developed for early detection of this disease. However, accurately segmenting the Region of Interest (ROI) from thermograms remains challenging. This paper presents an approach that leverages image acquisition protocol parameters to identify the lateral breast region and estimate its bottom boundary using a second-degree polynomial. The proposed method demonstrated high efficacy, achieving an impressive Jaccard coefficient of 86% and a Dice index of 92% when evaluated against manually created ground truths. Textural features were extracted from each view’s ROI, with significant features selected via Mutual Information for training Multi-Layer Perceptron (MLP) and K-Nearest Neighbors (KNN) classifiers. Our findings revealed that the MLP classifier outperformed the KNN, achieving an accuracy of 86%, a specificity of 100%, and an Area Under the Curve (AUC) of 0.85. The consistency of the method across both sides of the breast suggests its viability as an auto-segmentation tool. Furthermore, the classification results suggests that lateral views of breast thermograms harbor valuable features that can significantly aid in the early detection of breast cancer.Keywords
Cite This Article
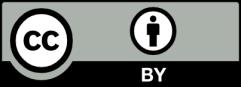
This work is licensed under a Creative Commons Attribution 4.0 International License , which permits unrestricted use, distribution, and reproduction in any medium, provided the original work is properly cited.