Open Access
ARTICLE
White Matter Lesions in Young-Middle Aged Migraineurs with Patent Foreman Ovale: A Case-Control Study
Department of Cardiology, The First Affiliated Hospital of Nanjing Medical University, Nanjing, 210000, China
* Corresponding Authors: Jing Shi. Email: ; Wei Sun. Email:
Congenital Heart Disease 2024, 19(3), 279-291. https://doi.org/10.32604/chd.2024.051190
Received 29 February 2024; Accepted 29 April 2024; Issue published 26 July 2024
Abstract
Background: White matter lesion (WML) is common in aging brain and is associated with cognitive impairment and dementia. However, recent studies reported an association between patent foramen ovale (PFO) and WML in migraineurs, especially in young, middle-aged migraineurs. Our retrospective, case-control study aims to describe the clinical characteristics of WML in this population and to explore potential risk factors. Methods: 226 patients with migraine and PFO were consecutively initially screened. Relevant factors were selected by the least absolute shrinkage and selection operator (LASSO) regression and multivariable logistic regression model. A Nomogram was employed to visualize the prediction model conveniently. The discrimination and calibration abilities were evaluated using the Receiver Operating Characteristic (ROC) curve, the Hosmer-Lemeshow test, and calibration curves. Results: One hundred and nineteen participants were ultimately enrolled in our study, with a median age of 36.9 ± 12.7 years and 80.7% of females. Brain magnetic resonance imaging MRI showed 67 (56.3%) patients had WML, whereas 52 (43.7%) patients were categorized into the non-WML group. LASSO regression screened out potential variables and subsequent multivariate analysis finally identified age, mean platelet volume, and fibrinogen (FIB) as independent predictive factors of WML. The area under the ROC curve (AUC) was 0.807. Hosmer-Lemeshow test and calibration curve verified a consistency between the predicted and actual probability. Conclusion: The predictive nomogram established and validated in our study may assist clinicians in screening WML among young middle-aged migraineurs with PFO and developing individualized preventive and treatment strategies.Keywords
Supplementary Material
Supplementary Material FileMigraine is a primary, chronic neurovascular headache with diverse symptoms, including recurrent unilateral disability, gastrointestinal symptoms, and sensitivity to light and sound stimuli. With a conservatively estimated 1-year prevalence of 15% worldwide, migraine accounts for the leading cause of disability among young, middle-aged adults and causes a heavy burden on global health, society, and the economy [1]. Foramen ovale is a physiological bypass of lesser circulation in the embryonic atrial septum. If there is still no anatomical closure after three years of birth, it is clinically called patent foramen ovale (PFO), which occurs in approximately 25% of adults and is the most common congenital heart disease [2]. Previous epidemiologic evidence has reported a bidirectional link between PFO and migraine. Several clinical trials have demonstrated the efficacy and safety of interventional occlusion for PFO in treating migraine [3]. Interventional closure of PFO is conditionally recommended in regional guidelines for those who show low responsiveness to medication after a comprehensive evaluation of the clinical decision group [4].
White matter lesion (WML) is a structural damage to the white matter of the brain characterized by foci of ischemic demyelination and gliosis and is manifested as abnormal white matter hyperintensities in T2-weighted sequences (T2WI) or fluid-attenuated inversion recovery (FLAIR) in magnetic resonance imaging (MRI) [5]. Pathologic mechanisms underlying WML are still unclear. Chronic microvascular damage due to brain aging, malnutrition, cerebrovascular disorders, and other neurologic diseases may cause WML. Multiple studies have verified the close association between WML and cognitive degradation, functional loss, and increasing mortality.
Previously, it was believed that migraine generally has no imaging brain structure abnormalities. However, with the development of image technology, WML associated with migraine is gradually being recognized and paid attention to, especially in young, middle-aged patients without harmful cardiovascular factors [6,7]. Growing evidence reported a possible link between migraine, PFO, and WML. PFO may play a role in the pathogenesis of WML in migraine [8]. Considering the high prevalence of PFO and migraine among young, middle-aged adults and that such a period is critical for brain vascular health and vascular risk factors control, young and middle-aged may serve as a crucial window for preventing cerebrovascular diseases. Currently, scarce literature exists regarding WML in young, middle-aged migraine patients with PFO, and relevant risk factors have not been well clarified in this population.
Hence, we conducted a cross-sectional, case-control study including young, middle-aged patients with migraine and PFO. Our study aimed to report the prevalence and clinical characteristics of WML in this population. We then explored the risk factors of WML and established a nomogram model to predict WML.
2.1 Study Population and Data Source
Between January 2019 and December 2021, a total of 226 patients consecutively admitted to the Cardiovascular department of the First Affiliated Hospital of Nanjing Medical University were initially screened. These patients were diagnosed with chronic and recurrent migraine with at least moderate right to left shunt (RLS). They decided to undergo interventional closure after a joint discussion by a consultation group composed of physicians from the Neurology and Cardiovascular department following the guidelines on management of PFO. Inclusion criteria were as follows: (1) diagnosed with migraine based on the International Classification of Headache Disorders, 3rd edition beta version [9]; (2) moderate to severe right-to-left shunt (RLS) confirmed by contrast-enhanced transcranial doppler (c-TCD); (3) age between 18 and 65 years; (4) complete brain MRI record during hospitalization or one week before hospitalization. Exclusion criteria were as follows: (1) secondary headache after severe trauma, cerebrovascular diseases, intracranial tumors, etc.; (2) missing data on brain MRI or c-TCD, or those who were not able to perform the Valsalva maneuver (VM) due to cognitive disorder or comorbidities; (3) age <18 or >65 years. The flowchart of the selection process is shown in Fig. 1.
Figure 1: Flowchart diagram of the study population according to inclusion criteria
Demographic information, laboratory, and instrumental examination were acquired from our inpatient electronic medical record system. Clinical data such as smoking, alcohol, migraine characteristics (onset of age, duration of headache, aura or not, and family history), and comorbid diseases such as hypertension, and diabetes mellitus (DM), were obtained by one-to-one questionnaire. The variables of interest included age, sex, laboratory test including platelet count (PLT), platelet crit (PCT), mean platelet volume (MPV), platelet distribution width (PDW), creatine kinase (CK), total cholesterol (TC), triglycerides (TG), low-density lipoprotein cholesterol (LDLc), fibrinogen (FIB), hypertension, DM, hyperlipemia, smoking, alcohol, RLS volume, permanent or latent RLS, the onset age of migraine, and duration of an attack.
The Ethics Committee of the First Affiliated Hospital of Nanjing Medical University approved the study procedures (2021-NT-17), and all the enrolled patients signed informed consent. All methods were carried out following the approved guidelines.
c-TCD examinations were performed by a 2-MHz probe and single channel connected to transcranial Doppler ultrasound (MVU-6300, Delica, China). Before detection and examination, the patient was informed to lie supine and practice the VM action. Agitated saline with microbubbles was rapidly injected through the cubital vein, and ultrasound changes in the middle cerebral artery were detected by experienced neurologists. The magnitude of RLS was quantified based on the maximum number of microbubbles (MBs) according to RLS international quantitative standard: Grade 0, null MBs; Grade I, 1 MBs; Grade II = 2 ≤ MBs ≤ 5; Grade III = 6 ≤ MBs ≤ 20; and Grade IV, MBs ≥ 20, with a curtain or shower pattern [10]. Moreover, RLS could be further divided into permanent and latent: permanent occurred during rest, and latent was triggered after a VM action (Fig. S1). In our study, only patients with Grades III and IV c-TCD were considered moderate to severe RLS and were admitted for interventional closure, which complied with the guidelines of PFO in China [11].
All the patients underwent 1.5-tesla brain MRI (uMR 560, UNITED IMAGING Company, Shanghai, China) during hospitalization or one week before hospitalization, which consists of T1-weighted sequences (T1WI), T2-weighted sequences (T2WI), and fluid-attenuated inversion recovery (FLAIR) sequences. All the imaging data of migraine patients were reviewed separately by an MRI diagnostic physician and a trained neurologist. Cases were divided into two groups according to the presence of WML. WML was defined as bilateral symmetry, multifocal spot or flake lesions in the deep or subcortical white matter, manifested as hyperintense signals on T2WI and FLAIR, and iso-intensity or slightly low signals on T1WI. WML can be classified into periventricular WML (PvWMLs) and deep WML (DWMLs): pvWMLs located in frontal, posterior horns and bands, whereas dWMLs in deep white matter and not attached to lateral ventricle lesions. The Fazekas scale (0~6 points) was utilized to assess the severity of WML by calculating scores of PvWMLs and dWMLs separately and summing the total points [12] (Fig. S2). PvWMLs: (1) 0 point: no lesion; (2) 1 point: cap or pencil-shaped thin layer lesions; (3) 2 points: smooth halo lesions; (4) 3 points: irregular large confluent lesions extending into the deep white matter. DWMLs: (1) 0 point: no lesion; (2) 1 point: punctate foci lesion; (3) 2 points: the beginning fusion of foci; (4) 3 points: large areas of lesion fusion. Any discrepancies of total points ≥2 points underwent a consensus.
Continuous variables were represented as mean (standard deviation), and categorical variables were expressed as percentage distributions. Chi-square test and one-way ANOVA were employed to analyze differences in continuous and categorical variables between patients with WMLs and without WMLs, respectively. The “glmnet” package in R4.2 was used to perform the least absolute shrinkage and selection operator (LASSO) regression and screen for potential covariates based on the λ. Variables selected by Lasso regression were included in multivariable logistic regression analysis. Multivariable logistic regression with a step-by-step forward regression was further used to explore the association between the former potential variables and WMLs. All variables that were found to be independently significant in the multivariable analysis (p < 0.05) were included in the final model and used to construct a nomogram for predicting the probability of WML via “rms” package in R4.2. According to the regression coefficient, each variable corresponded to a definite value in reference to the nomogram, and the total points for each patient are the sum. Clinicians may predict WML in a particular case by checking the relationship between total points and probability visualized at the bottom of the nomogram. Several evaluation methods were used to describe the discrimination and calibration of the model using the Bootstrap method after repeat sampling 1000 times for internal verification. The receiver operating characteristic (ROC) curve was drawn, and the area under the curve (AUC) was used to evaluate discriminative ability. Typically, AUC ranges from 0 to 1, and a value greater than 0.7 indicates a reasonable estimation performance. H-L goodness of fit and the calibration curve was adopted to evaluate the calibration ability of the model. The net reclassification index (NRI) and decision curve analysis (DCA) were depicted to reflect the clinical benefit of the nomogram compared with the treat-all strategy (representing the highest clinical cost) and the treat-none strategy (representing the lowest clinical benefit) [13]. All statistical analysis was performed via R software. p-value < 0.05 was set as statistically significant for all analyses.
Between January 2019 and December 2021, a total of 226 patients diagnosed with migraine and PFO were admitted to the Cardiovascular department of the first affiliated hospital of Nanjing Medical University. After removing those aged <18 years, aged >65 years, and having missing data on MRI and cTCD, 119 participants were ultimately enrolled in our study. The demographic and clinical characteristics are shown in Table 1.
The mean age of the enrolled participants was 36.9 years, and Males:Females was 19.3%:80.7%. According to brain MRI, 67 (56.3%) patients were categorized into the WML group, whereas 52 (43.7%) were in the non-WML group. Among the WML group, most patients had a Fazekas scale of 1–2 points whereas 8 patients reached 3 points. No obvious association was observed between larger RLS volume and a higher degree of WML assessed by the Fazekas scale (Fig. S3). Compared with the non-WML group, MPV, FIB, and duration of migraine were significantly higher in the WML group (all p < 0.05).
Considering the large number of variables included in this study and limited outcome events, Lasso regression was used to process variables systematically and to select potential variables that can predict the occurrence of WML. 10-fold cross-validation computes the estimate of the optimal parameter of λ. With larger λ, weak factors shrink towards zero, and those that remained in the model were considered strong predictors (Fig. 2A). To prevent over-fitting, a λ of four was selected, which gives a model with good performance but a minimum number of variables (Fig. 2B). Four factors (age, FIB, MPV, CK) with nonzero coefficients were selected by LASSO regression and preliminarily considered as the statistically significant factors related to WML. Later entered into logistic regression models using forward stepwise, CK was left out, whereas age, MPV, and FIB were finally chosen as important independent diagnostic factors for WML in patients with migraine and PFO (Table 2). The results showed that age (odds ratio [OR], 1.087; 95% confidence interval [CI], 1.046–1.135; p < 0.001), MPV (odds ratio [OR], 1.488; 95% confidence interval [CI], 1.054–2.169; p = 0.029), FIB (odds ratio [OR], 3.237; 95% confidence interval [CI], 1.246–9.306; p = 0.021) were significantly associated with increased risk of WML.
Figure 2: Texture feature selection using the least absolute shrinkage and selection operator (LASSO) binary logistic regression model. (A) Identification of the optimal penalization coefficient lambda (λ) in the LASSO model with 10-fold cross-validation. The dotted line on the left indicates the number of variables corresponding to the minimum λ (when the model has the highest fitting effect), and the number was 4. (B) A Path diagram of the regression coefficient. 23 variables were included, 3 variables were finally selected based on the optimal penalty coefficient, which was age, MPV, and FIB
A predictive nomogram of WML was constructed based on the results selected from multivariable logistic analysis (Fig. 3). As shown in the nomogram, older patients with higher MPV and FIB were more likely to have WML. Each risk factor was divided into several segments and assigned a corresponding point on the top based on its coefficient in the regression analysis. The total score was the sum of each point, and therefore, clinicians could estimate the risk of WML at the patient presentation by checking in reference to the scale below. Take a hypothetical case illustrating our nomogram usage for example: if a 35-year-old patient with migraine and PFO came to the outpatient clinic of the Neurology department with his FIB and MPV of 3.2 and 11 by a laboratory test, then the point assigned to each risk factor was 40, 50, and 40, respectively, which generated a total score of 130 and a predicted probability of 75% for WML. With this helpful tool, clinicians may preliminary judge one’s risk of WML and provide appropriate medical strategies such as brain MRI scans or computed tomography (CT).
Figure 3: Nomogram of probability to develop WML. The nomogram is used by summing all points identified on the scale for each variable. The total points projected on the bottom scales indicate the probabilities of WML
Bootstrap sampling method with 1000 times self-sampling was used for internal validation to evaluate the stability and credibility of the prediction model. The ROC curve showed that the AUC of the prediction model was 80.7% (95% CI: 73.0%–88.4%) (Fig. 4A). The sensitivity and specificity corresponding to the best cut-off value are 82.7% and 71.6%, respectively. The calibration curves of the nomogram depicted a mean absolute error (MAE) of 0.052, indicating excellent consistencies between the predicted and apparent probability (Fig. 4B). The Hosmer–Lemeshow test explored no significant difference between the predicted and actual probability (X2 = 11.01, p = 0.20). Therefore, the prediction model exhibited considerable discriminative and calibrating abilities for WML. Moreover, the DCA curve assessed the clinical utility, which overtopped and had no intersection with the two extreme curves, suggesting a net benefit across a broader range of threshold probabilities than the treat-none and treat-all schemes (Fig. S4).
Figure 4: (A) ROC curve for the nomogram based on the cohort. The AUC and its 95% confidence interval are shown in the plots. The AUC is 0.807, with 95% CI 73.0%–88.4%. The sensitivity and specificity corresponding to the best cut-off value are 82.7% and 71.6%, respectively. AUC, the area under the curve; ROC, receiver operating characteristic. (B) Calibration curves of the nomogram for predicting WML from the cohort. The Hosmer–Lemeshow test had a p-value of 0.20
WML is a common neurological disease mainly occurring in the elderly, with its incidence rising sharply with age. Substantial evidence has linked WML with deterioration of cognitive ability, impaired language and motor function, and elevated occurrence of neurodegenerative diseases such as dementia and Alzheimer’s disease [14]. However, recent studies reported a considerable prevalence of WML in patients with migraine and PFO, re-arousing people’s attention to such degenerative neurological disease. Our study reported the prevalence and clinical characteristics of WML in young, middle-aged adults with migraine and PFO in a single medical center. We then tried to establish a prediction model of WML and visualize it in a nomogram. Several independent variables were selected by LASSO regression and multivariable logistic regression and were finally included in the model. Measured by standard deviation along nomogram scales, age ranked the most critical risk factor, followed by MPV and FIB. Internal verification proved satisfactory discrimination, calibration capabilities, and clinical application value.
Age was the most decisive associated factor of WML. As age grows, the prevalence of WML pointedly increases. Cognitive Function and Ageing Study (CFAS) neuropathology cohort, a longitudinal cohort aimed to investigate cognitive and frailty behaviors in the elderly, was launched in England. Among 465 consecutive subjects aged over 65 years, WML was found in 87% of healthy-looking individuals and 95% of demented individuals [15]. Such a result was similar to previous studies, which suggested an overall prevalence of 90% across life and a coherence between the volume of lesions and increasing age [16]. Histopathologic research using post-mortem samples from CFAS study suggested WML is an active outward manifestation of aging-related white matter abnormality with a complex pathogenesis via crosstalk of ischemia/hypoxia, microglial activation, blood-brain barrier (BBB) alterations, and glial cell injury [17]. With the increase of age, the myelinated nerve fibers in the white matter gradually decrease, and the length of myelinated axons gradually shorten. Additionally, elderly patients are accompanied by cerebral atherosclerosis, vascular endothelial dysfunction of cerebral microcirculation, and chronic cerebral hypoperfusion, leading to BBB’s damage and the extravasation of toxic substances [18].
MPV is defined as the average size of the circulating platelet and reflects platelet activity. As a surrogate biomarker for thrombosis, vascular injury, and inflammatory process, MPV is closely related to cardiovascular diseases (CVDs) risk factors and exhibits excellent diagnostic and prognostic value in CVDs, such as coronary artery disease and ischemic stroke [19,20]. A Korean study including 870 patients with WML on brain MRI was aimed to investigate the association between MPV and WML in the elderly population [21]. Results showed that compared to the lowest tertile, the highest MPV tertile had a 50% increased risk of WML (OR: 1.51, 95% CI: 1.04–2.20). MPV level was parallel to the severity of WML evaluated by Fazekas scores. Consistently, another cross-sectional study also observed higher MPV values in the WML group and an independent positive association between MPV and WML. A possible explanation may lie in enhanced platelet activation, acknowledged as a primary pathology of cerebral small vessel disease (CSVD), including silent lacunar infarction, cerebral microbleeds, and cerebral WML. Large platelets contain more platelet-derived granules and behave more actively in function than smaller ones in vasoactive and prothrombotic secretions, thromboxane synthesis, and expression of glycoprotein IIb/IIIa receptors [22]. Endothelial dysfunction, chronic low-grade inflammation, and atherothrombosis were promoted, which participated in the progression of WML [23].
Fibrinogen is involved in many physiological processes, including hemostasis, platelet aggregation, and endothelial cell interaction [24]. As a determinant of blood viscosity and state of hypercoagulability, fibrinogen could reflect atherosclerosis progression and exhibit significant clinical value in ASCVDs [25] and thrombotic diseases [26]. A cross-sectional study comprising 958 individuals in Japan demonstrated that each 1-SD increase in fibrinogen level was associated with a 26% increased risk of silent cerebrovascular lesions. The odds ratio with 95% CI for WML and lacunar infarcts in the highest tertile compared with the lowest tertile were 1.78 (1.20–2.65) and 1.70 (1.09–2.65), respectively [27]. Similarly, a significant association between fibrinogen and WML was reported in a community study containing 667 local elderly residents, independent of conventional risk factors and high sensitivity to C-reactive protein [28]. Further analysis discerned a non-significant tendency between higher fibrinogen levels and worse cognitive function assessed by Mini-Mental State Examination (MMSE) scores. The exact pathological mechanisms between fibrinogen and WML are still blurry. First, fibrinogen represents the inflammation and hypercoagulability level throughout the whole body and is also a significant determinant of plasma viscosity and platelet aggregation. Fibrinogen is involved in vascular endothelial cell proliferation, injury, and atherosclerosis progression, contributing to atherogenesis and ischemia in small brain vessels [29]. Second, platelet aggregation may cause vascular injury and endothelial dysfunction, which was assumed to be responsible for BBB dysfunction and subsequent fibrinogen extravasation. Extravascular fibrinogen deposition in white matter was found in glial cells, neurons, interstitial pools, and parenchyma by neuropathology studies [30]. Such deposition may exert potential toxic effects on white matter, causing hypoperfusion and aggravating BBB dysfunction. However, whether fibrinogen-lowing therapies could reduce the risk of WML or cerebrovascular diseases still needs future research with large samples to clarify.
The young, middle-aged participants enrolled in our study provided several enlightenments. Unlike previous research focusing on WML in the elderly, WML in the young-middle population draws growing interest nowadays. The principal reason lies in the lack of long-term unhealthy living habits and poorly controlled CVD risk factors in young people, contrary to the elderly. An epidemiological study containing 428 voluntary community-based healthy individuals aged 44–48 years demonstrated a prevalence of 50.9% for WML and an average WML cluster of 1.37 per brain [31]. Another registry study (PORTYWHITE) focused on young WML adults in 12 neurological outpatient centers in Portugal. Seventy-seven patients aged between 18 and 55 were enrolled, and 88.3% had vascular risk factors (53.2% with hypertension and 36.4% with previous stroke) [32]. The percentage of females combined with migraine was higher in individuals without vascular risk factors. The author recommended thorough screening for potential vascular risk factors even though WML was occasionally discerned by MRI. Theoretically, the earlier the beginning period of WML, the worse consequences one may acquire in the future. Such brain damage is predominantly irreversible and may continuously progress throughout the lifetime [33]. A meta-analysis consisting of 22 longitudinal studies reported the association between WML and increased risk of cerebrovascular events, including stroke (HR 3.3, 95% CI 2.6 to 4.4), dementia (HR 1.9, 95% CI 1.3 to 2.8), and death (HR 2.0, 95% CI 1.6 to 2.7) [34]. A decline in global cognitive function, organizational performance, and movement speed was also reported.
Migraine is the most common medical condition that predisposes young adults to developing and progressing WML, even among pediatric patients [35]. Observational studies found a twofold to fourfold increased risk of WML in migraine patients and a consistency between WML load and migraine severity [36]. Additionally, the accuracy of WML in predicting future symptomatic brain ischemia and stroke risk in migraine patients was also reported [37]. Currently, no precise data exist regarding the prevalence of WML in patients with migraine. Epidemiological studies concluded a proportion of 6% to 46%, compared with 4%–14% in the general population. Aura before the episode, attack frequency, and migraine duration were regarded as indicators of brain damage. A cohort of 17 migraine patients (median age 41.7 years; 15 females and two males) in Hungary demonstrated that after a follow-up for three years, an increasing number of newly occurred WMLs and volume of hyperintensities were observed in nearly all the patients due perhaps to uncontrolled repeated migraine attacks [38]. A history of hypertension, higher systolic blood pressure values, and migraine with aura were also assumed predictive factors for the progression of WML [39]. Pathological mechanisms underlying migraine-related WML are yet unclear. Increased platelet aggregability, clotting activation, intravascular microthrombosis, and reactive astrocytic gliosis-induced injury may be implicated [8]. Recent studies reported the association between PFO and WML in migraine patients. Iwasaki et al. reported a higher prevalence of PFO in the WML (+) group and an independent predictive role of RLS in the presence of WML after adjustment for age and migraine duration [8]. A recent meta-analysis comprising 1125 subjects with migraine found that 58.7% of patients with PFO and migraine had WML, similar to our study of 56.3%. The presence of PFO was significantly associated with WML [40]. The possible relationship between PFO and WML in patients with migraine may presumably be attributed to paradoxical microembolism, defined as the bypass of subclinical emboli in the vein from the pulmonary circulation into the arterial system and is the most typical feature of pathophysiology surrounding PFO [41]. On the contrary, several studies reported little association between PFO and WML in patients with migraine [42]. Based on current evidence, no definite consensus was reached on the role of RLS in WML. To some extent, WML may represent signs of PFO-related paradoxical microembolic events in the brain. The existence of RLS was more an accelerator than a pathogenic factor in the development of WML.
In our study, the prevalence of WML in young, middle-aged adults was 56.3%. In such a period, it is relatively practical to control vascular risk factors and modify unhealthy lifestyles. Such a period is critical in preventing and postponing WML. For young adults with headaches admitted to the outpatient neurological department, a meticulous series of examinations is usually prescribed in consideration of possible brain tumors or other systemic abnormalities. However, for those with routine migraine attacks, standardized diagnosis and treatment are still of pivotal significance. A brain MRI is recommended to discern WML as early identification of WML in young adults shall make a difference in preventing and alleviating brain damage in public health prevention settings via enhanced routine medical follow-up and control of later-appearing CVD risk factors or comorbidities [43]. Moreover, screening for PFO (e.g., c-TCD, contrast transthoracic echocardiography) is also necessary. For those who show low responsiveness to medication or recurrent migraine, transcatheter interventional closure might be suitable to reduce the adverse brain damage caused by large RLS and episodic migraine attacks [41]. Longitudinal cohorts targeting young, middle-aged migraineurs are needed to clarify the causal relationship between the progression of age-exclusive WML and long-term clinical outcomes.
There are several advantages of our study. In this study, we developed a diagnostic model based on the relevant indicators to estimate the risk of WML in young middle-aged patients with migraine and PFO. We used a nomogram to visualize the probability via a simple graphical representation, making the prediction model applicable in clinical settings. Moreover, the young nature of enrolled participants conforms to the recent growing attention on WML in the young population rather than aging WML. Our study supplies limited existing evidence on WML’s prevalence and related factors in young populations. However, there are still some limitations in our study. First, as this study is retrospective, selection bias is inevitable. Second, the subjects enrolled in our study were from a single center, and the sample size was limited, which may limit the generalization of our nomogram and restrict its application to patients in other regions. Third, the nature of our cross-sectional study hinders us from inferring any causal relationship between WML and risk factors. No follow-up data were available regarding cognitive function, cerebrovascular diseases, and specific-cause mortality. Cross-link, high-quality longitudinal cohorts constructed under the cooperation between the Neurology department and Cardiovascular department targeting long-term outcomes are needed in the future.
Age, mean platelet volume, and fibrinogen were independent risk factors of WML in young middle-aged migraineurs with PFO. The nomogram in our study may assist clinicians in screening WML and developing individualized preventive and treatment strategies.
Acknowledgement: None.
Funding Statement: None.
Author Contributions: WS and JS conceived and designed the study; YH and JYS analyzed the data; YH wrote the paper; YXL and HZ reviewed and revised the paper. All authors provided critical revisions of the manuscript and approved the final manuscript.
Availability of Data and Materials: Additional data and materials may be requested from the corresponding author upon reasonable request.
Ethics Approval: The studies involving human participants were reviewed and approved by the Research Ethics Committee of the First Affiliated Hospital of Nanjing Medical University (2021-NT-17). The patients provided their written informed consent to participate in this study.
Conflicts of Interest: The authors declare that they have no conflicts of interest to report regarding the present study.
Supplementary Materials: The supplementary material is available online at https://doi.org/10.32604/chd.2024.051190.
References
1. Ashina M, Katsarava Z, Do TP, Buse DC, Pozo-Rosich P, Özge A, et al. Migraine: epidemiology and systems of care. Lancet. 2021;397(10283):1485–95. doi:10.1016/S0140-6736(20)32160-7. [Google Scholar] [PubMed] [CrossRef]
2. Hagen PT, Scholz DG, Edwards WD. Incidence and size of patent foramen ovale during the first 10 decades of life: an autopsy study of 965 normal hearts. Mayo Clin Proc. 1984;59(1):17–20. doi:10.1016/S0025-6196(12)60336-X. [Google Scholar] [PubMed] [CrossRef]
3. Mojadidi MK, Kumar P, Mahmoud AN, Elgendy IY, Shapiro H, West B, et al. Pooled analysis of PFO occluder device trials in patients with PFO and migraine. J Am Coll Cardiol. 2021;77(6):667–76. doi:10.1016/j.jacc.2020.11.068. [Google Scholar] [PubMed] [CrossRef]
4. Teshome MK, Najib K, Nwagbara CC, Akinseye OA, Ibebuogu UN. Patent foramen ovale: a comprehensive review. Curr Probl Cardiol. 2020;45(2):100392. doi:10.1016/j.cpcardiol.2018.08.004. [Google Scholar] [PubMed] [CrossRef]
5. Cabrera J, Urmeneta Ulloa J, Jímenez de la Peña M, Rubio Alonso M, López Gavilán M, Bayona Horta S, et al. White-matter lesions and cortical cerebral blood flow evaluation by 3D arterial spin-labeled perfusion MRI in asymptomatic divers: correlation with patent foramen ovale ocurrence. J Clin Med. 2023;12(8):2866. doi:10.3390/jcm12082866. [Google Scholar] [PubMed] [CrossRef]
6. Kruit MC, van Buchem MA, Hofman PA, Bakkers JT, Terwindt GM, Ferrari MD, et al. Migraine as a risk factor for subclinical brain lesions. JAMA. 2004;291(4):427–34. doi:10.1001/jama.291.4.427. [Google Scholar] [PubMed] [CrossRef]
7. Dinia L, Bonzano L, Albano B, Finocchi C, Del Sette M, Saitta L, et al. White matter lesions progression in migraine with aura: a clinical and MRI longitudinal study. J Neuroimaging. 2013;23(1):47–52. doi:10.1111/jon.2013.23.issue-1. [Google Scholar] [CrossRef]
8. Iwasaki A, Suzuki K, Takekawa H, Takashima R, Suzuki A, Suzuki S, et al. The relationship between right-to-left shunt and brain white matter lesions in Japanese patients with migraine: a single center study. J Headache Pain. 2017;18(1):3. doi:10.1186/s10194-016-0714-x. [Google Scholar] [PubMed] [CrossRef]
9. Headache Classification Committee of the International Headache Society (IHS). The international classification of headache disorders, 3rd edition (beta version). Cephalalgia. 2013;33(9):629–808. doi:10.1177/0333102413485658. [Google Scholar] [PubMed] [CrossRef]
10. Ning M, Lo EH, Ning PC, Xu SY, McMullin D, Demirjian Z, et al. The brain’s heart-therapeutic opportunities for patent foramen ovale (PFO) and neurovascular disease. Pharmacol Ther. 2013;139(2):111–23. doi:10.1016/j.pharmthera.2013.03.007. [Google Scholar] [PubMed] [CrossRef]
11. National Interventional Quality Control Center for Structural Heart Disease of National Health Commission, Interventional Quality Control Center for Structural Heart Disease of National Cardiovascular Disease Center, Working Group of Guidelines for Percutaneous Interventional Treatment of Congenital Heart Disease of Cardiology Society of Chinese Medical Association, Working Group of Guidelines for Percutaneous Interventional Treatment of Congenital Heart Disease of Thoracic Cardiovascular Surgery Society of Chinese Medical Association. Guidelines for percutaneous interventional treatment of common congenital heart diseases (2021 edition). Nat Med J China. 2021;101(38):3054–76. [Google Scholar]
12. Zeng W, Chen Y, Zhu Z, Gao S, Xia J, Chen X, et al. Severity of white matter hyperintensities: lesion patterns, cognition, and microstructural changes. J Cereb Blood Flow Metab. 2020;40(12):2454–63. doi:10.1177/0271678X19893600. [Google Scholar] [PubMed] [CrossRef]
13. Pencina MJ, D’Agostino SrRB, Steyerberg EW. Extensions of net reclassification improvement calculations to measure usefulness of new biomarkers. Stat Med. 2011;30(1):11–21. doi:10.1002/sim.v30.1. [Google Scholar] [CrossRef]
14. Breteler MM, van Swieten JC, Bots ML, Grobbee DE, Claus JJ, van den Hout JH, et al. Cerebral white matter lesions, vascular risk factors, and cognitive function in a population-based study: the Rotterdam Study. Neurology. 1994;44(7):1246–52. doi:10.1212/WNL.44.7.1246. [Google Scholar] [PubMed] [CrossRef]
15. Matthews FE, Brayne C, Lowe J, McKeith I, Wharton SB, Ince P. Epidemiological pathology of dementia: attributable-risks at death in the medical research council cognitive function and ageing study. PLoS Med. 2009;6(11):e1000180. doi:10.1371/journal.pmed.1000180. [Google Scholar] [PubMed] [CrossRef]
16. de Leeuw FE, de Groot JC, Achten E, Oudkerk M, Ramos LM, Heijboer R, et al. Prevalence of cerebral white matter lesions in elderly people: a population based magnetic resonance imaging study. The Rotterdam Scan Study. J Neurol Neurosurg Psychiatry. 2001;70(1):9–14. doi:10.1136/jnnp.70.1.9. [Google Scholar] [PubMed] [CrossRef]
17. Wharton SB, Simpson JE, Brayne C, Ince PG. Age-associated white matter lesions: the MRC cognitive function and ageing study. Brain Pathol. 2015;25(1):35–43. doi:10.1111/bpa.2015.25.issue-1. [Google Scholar] [CrossRef]
18. Lam BYK, Yiu B, Ampil E, Chen CL, Dikot Y, Dominguez JC, et al. High burden of cerebral white matter lesion in 9 Asian cities. Sci Rep. 2021;11(1):11587. doi:10.1038/s41598-021-90746-x. [Google Scholar] [PubMed] [CrossRef]
19. Chu SG, Becker RC, Berger PB, Bhatt DL, Eikelboom JW, Konkle B, et al. Mean platelet volume as a predictor of cardiovascular risk: a systematic review and meta-analysis. J Thromb Haemost. 2010;8(1):148–56. doi:10.1111/j.1538-7836.2009.03584.x. [Google Scholar] [PubMed] [CrossRef]
20. Vizioli L, Muscari S, Muscari A. The relationship of mean platelet volume with the risk and prognosis of cardiovascular diseases. Int J Clin Pract. 2009;63(10):1509–15. doi:10.1111/ijcp.2009.63.issue-10. [Google Scholar] [CrossRef]
21. Choi JW, Lee KO, Jang YJ, Kim HK, Seo T, Roh YJ, et al. High mean platelet volume is associated with cerebral white matter hyperintensities in non-stroke individuals. Yonsei Med J. 2023;64(1):35–41. doi:10.3349/ymj.2022.0368. [Google Scholar] [PubMed] [CrossRef]
22. Thompson CB, Eaton KA, Princiotta SM, Rushin CA, Valeri CR. Size dependent platelet subpopulations: relationship of platelet volume to ultrastructure, enzymatic activity, and function. Br J Haematol. 1982;50(3):509–19. doi:10.1111/bjh.1982.50.issue-3. [Google Scholar] [CrossRef]
23. Kang SJ, Park BJ, Shim JY, Lee HR, Hong JM, Lee YJ. Mean platelet volume (MPV) is associated with leukoaraiosis in the apparently healthy elderly. Arch Gerontol Geriatr. 2012;54(2):e118–21. doi:10.1016/j.archger.2011.10.010. [Google Scholar] [PubMed] [CrossRef]
24. Kannel WB. Influence of fibrinogen on cardiovascular disease. Drugs. 1997;54(Suppl 3):32–40. [Google Scholar] [PubMed]
25. Eidelman RS, Hennekens CH. Fibrinogen: a predictor of stroke and marker of atherosclerosis. Eur Heart J. 2003;24(6):499–500. doi:10.1016/S0195-668X(02)00810-2. [Google Scholar] [PubMed] [CrossRef]
26. Maners J, Gill D, Pankratz N, Laffan MA, Wolberg AS, de Maat MPM, et al. A Mendelian randomization of γ′ and total fibrinogen levels in relation to venous thromboembolism and ischemic stroke. Blood. 2020;136(26):3062–9. doi:10.1182/blood.2019004781. [Google Scholar] [PubMed] [CrossRef]
27. Aono Y, Ohkubo T, Kikuya M, Hara A, Kondo T, Obara T, et al. Plasma fibrinogen, ambulatory blood pressure, and silent cerebrovascular lesions: the Ohasama study. Arterioscler Thromb Vasc Biol. 2007;27(4):963–8. doi:10.1161/01.ATV.0000258947.17570.38. [Google Scholar] [PubMed] [CrossRef]
28. Wada M, Takahashi Y, Iseki C, Kawanami T, Daimon M, Kato T. Plasma fibrinogen, global cognitive function, and cerebral small vessel disease: results of a cross-sectional study in community-dwelling Japanese elderly. Intern Med. 2011;50(9):999–1007. doi:10.2169/internalmedicine.50.4752. [Google Scholar] [PubMed] [CrossRef]
29. Bernbaum M, Menon BK, Fick G, Smith EE, Goyal M, Frayne R, et al. Reduced blood flow in normal white matter predicts development of leukoaraiosis. J Cereb Blood Flow Metab. 2015;35(10):1610–5. doi:10.1038/jcbfm.2015.92. [Google Scholar] [PubMed] [CrossRef]
30. Hainsworth AH, Minett T, Andoh J, Forster G, Bhide I, Barrick TR, et al. Neuropathology of white matter lesions, blood-brain barrier dysfunction, and dementia. Stroke. 2017;48(10):2799–804. doi:10.1161/STROKEAHA.117.018101. [Google Scholar] [PubMed] [CrossRef]
31. Wen W, Sachdev PS, Li JJ, Chen X, Anstey KJ. White matter hyperintensities in the forties: their prevalence and topography in an epidemiological sample aged 44–48. Hum Brain Mapp. 2009;30(4):1155–67. doi:10.1002/hbm.v30:4. [Google Scholar] [CrossRef]
32. Viana-Baptista M, Cruz ESV, Caetano A, Marto JP, Azevedo E, Ferreira C, et al. Vascular white matter lesions in young adults: a neurology outpatient clinic registry. Eur Neurol. 2019;82(1–3):23–31. [Google Scholar] [PubMed]
33. Schmidt R, Fazekas F, Kapeller P, Schmidt H, Hartung HP. MRI white matter hyperintensities: three-year follow-up of the Austrian stroke prevention study. Neurology. 1999;53(1):132–9. doi:10.1212/WNL.53.1.132. [Google Scholar] [PubMed] [CrossRef]
34. Debette S, Markus HS. The clinical importance of white matter hyperintensities on brain magnetic resonance imaging: systematic review and meta-analysis. BMJ. 2010;341:c3666. doi:10.1136/bmj.c3666. [Google Scholar] [PubMed] [CrossRef]
35. Eidlitz-Markus T, Zeharia A, Haimi-Cohen Y, Konen O. MRI white matter lesions in pediatric migraine. Cephalalgia. 2013;33(11):906–13. doi:10.1177/0333102413480955. [Google Scholar] [PubMed] [CrossRef]
36. Swartz RH, Kern RZ. Migraine is associated with magnetic resonance imaging white matter abnormalities: a meta-analysis. Arch Neurol. 2004;61(9):1366–8. doi:10.1001/archneur.61.9.1366. [Google Scholar] [PubMed] [CrossRef]
37. Etminan M, Takkouche B, Isorna FC, Samii A. Risk of ischaemic stroke in people with migraine: systematic review and meta-analysis of observational studies. BMJ. 2005;330(7482):63. doi:10.1136/bmj.38302.504063.8F. [Google Scholar] [PubMed] [CrossRef]
38. Erdélyi-Bótor S, Aradi M, Kamson DO, Kovács N, Perlaki G, Orsi G, et al. Changes of migraine-related white matter hyperintensities after 3 years: a longitudinal MRI study. Headache. 2015;55(1):55–70. doi:10.1111/head.2015.55.issue-1. [Google Scholar] [CrossRef]
39. Jiang J, Yao K, Huang X, Zhang Y, Shen F, Weng S. Longitudinal white matter hyperintensity changes and cognitive decline in patients with minor stroke. Aging Clin Exp Res. 2022;34(5):1047–54. doi:10.1007/s40520-021-02024-5. [Google Scholar] [PubMed] [CrossRef]
40. Yeo JYP, Goh CXY, Tan YK, Sim BTS, Chan BLX, Syn NL, et al. Evaluating the relationship between right-to-left shunt and white matter hyperintensities in migraine patients: a systematic review and meta-analysis. Front Neurol. 2022;13:972336. doi:10.3389/fneur.2022.972336. [Google Scholar] [PubMed] [CrossRef]
41. Park HK, Lee SY, Kim SE, Yun CH, Kim SH. Small deep white matter lesions are associated with right-to-left shunts in migraineurs. J Neurol. 2011;258(3):427–33. doi:10.1007/s00415-010-5771-5. [Google Scholar] [PubMed] [CrossRef]
42. Jiang XH, Wang SB, Tian Q, Zhong C, Zhang GL, Li YJ, et al. Right-to-left shunt and subclinical ischemic brain lesions in Chinese migraineurs: a multicentre MRI study. BMC Neurol. 2018;18(1):18. doi:10.1186/s12883-018-1022-7. [Google Scholar] [PubMed] [CrossRef]
43. Chen Y, Wang X, Guan L, Wang Y. Role of white matter hyperintensities and related risk factors in vascular cognitive impairment: a review. Biomolecules. 2021;11(8):1102. doi:10.3390/biom11081102. [Google Scholar] [PubMed] [CrossRef]
Cite This Article
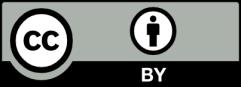
This work is licensed under a Creative Commons Attribution 4.0 International License , which permits unrestricted use, distribution, and reproduction in any medium, provided the original work is properly cited.