Open Access
ARTICLE
CHDTEPDB: Transcriptome Expression Profile Database and Interactive Analysis Platform for Congenital Heart Disease
1 The First Department of Cardiology, The First Affiliated Hospital of Harbin Medical University, Harbin, 150001, China
2 Central Laboratory, The First Affiliated Hospital of Harbin Medical University, Harbin, 150001, China
3 Oncology Ward 2, Beidahuang Industry Group General Hospital (Heilongjiang Second Cancer Hospital), Harbin, 150001, China
4 Research and Development Department, Hangzhou Mugu Technology Co., Ltd., Hangzhou, 311113, China
* Corresponding Author: Jiyi Zhao. Email:
Congenital Heart Disease 2023, 18(6), 693-701. https://doi.org/10.32604/chd.2024.048081
Received 27 November 2023; Accepted 29 December 2023; Issue published 19 January 2024
Abstract
CHDTEPDB (URL: ) is a manually integrated database for congenital heart disease (CHD) that stores the expression profiling data of CHD derived from published papers, aiming to provide rich resources for investigating a deeper correlation between human CHD and aberrant transcriptome expression. The development of human diseases involves important regulatory roles of RNAs, and expression profiling data can reflect the underlying etiology of inherited diseases. Hence, collecting and compiling expression profiling data is of critical significance for a comprehensive understanding of the mechanisms and functions that underpin genetic diseases. CHDTEPDB stores the expression profiles of over 200 sets of 7 types of CHD and provides users with more convenient basic analytical functions. Due to the differences in clinical indicators such as disease type and unavoidable detection errors among various datasets, users are able to customize their selection of corresponding data for personalized analysis. Moreover, we provide a submission page for researchers to submit their own data so that increasing expression profiles as well as some other histological data could be supplemented to the database. CHDTEPDB is a user-friendly interface that allows users to quickly browse, retrieve, download, and analyze their target samples. CHDTEPDB will significantly improve the current knowledge of expression profiling data in CHD and has the potential to be exploited as an important tool for future research on the disease.Keywords
Congenital heart disease (CHD) refers to a structural abnormality of the heart and/or great vessels already present at birth [1]. CHD as one of the most common birth defects worldwide affects about 1% of newborns [2]. Currently, more adults than children suffer from CHD as a result of major medical and technological advances in recent years. While infant mortality due to congenital heart disease in the last four decades decreased by almost 3-fold, adult congenital heart disease prevalence increased by more than 2-fold [3]. Advances in genomic technology have revealed a number of genetic etiologies that affect CHD, which also points to complex mechanisms in the genetics of CHD and addresses an urgent need for establishing high-quality expression profiling databases to facilitate the research on CHD. Existing research shows that mining genetics can help discover new etiologies, improve diagnosis and counseling, understand disease mechanisms of CHD, and may provide patients with precision medicine [4].
In recent years, a considerable number of CHD-related databases have been developed, for example, Cheyne [5]: A Curated Database for Congenital Heart Disease Genes, This is a database solely focused on Low-throughput experimentally proved gene with congenital heart disease, while lacking specialized standardization and analysis tools for transcriptome data; Pediatric Heart Network (PHN) [6] is a consortium that focuses on improving outcomes for individuals with congenital and pediatric-acquired heart disease. It has completed nearly 20 clinical studies, enrolling over 3,500 patients, but it is not specifically designed for transcriptomic data analysis and may lack the detailed genetic and molecular data required for such studies; STS Congenital Heart Surgery Database (CHSD) [7] stands out as one of the most extensive clinical outcomes. It offers an extensive dataset, which is invaluable for understanding the clinical dimensions of congenital heart diseases. The primary focus of the STS CHSD is on clinical outcomes rather than molecular or genetic data, which are crucial for transcriptomic analysis.; UK Congenital Heart Disease Database (NCHDA) [8] provides a vast amount of clinical data from various centers, which could be beneficial for broad clinical research in congenital heart disease and offers detailed profiles of every congenital heart disease center in the UK, including the number and types of procedures carried out and survival rates, which can be valuable for clinical outcome studies. The primary aim of NCHDA is to audit clinical outcomes rather than to provide molecular or genetic data, which are crucial for detailed transcriptomic analysis, for transcriptomic studies that require in-depth genetic or molecular information, the database may not be sufficiently detailed. These databases prioritize surgery and clinical work, with minimal involvement in molecular-level omics research, but there is a lack of expression profile databases for the disease. CHD is a typical and highly prevalent genetic disease, and the study of it demands high-quality expression profiling data. To bridge such a gap, we established the present database.
CHDTEPDB is developed to store and integrate comprehensive expression profiling data of CHD to provide simple bioinformatics analyses based on these data. The data contained in the present CHDTEPDB were manually collected and curated on the basis of previously published papers. Currently, over 200 sets of data with 800 samples have been collected, covering 7 different common types of CHD including patent ductus arteriosus, atrial septal defect, ventricular septal defect, aortopulmonary septal defect, pulmonary valve stenosis, patent foramen ovale and tetralogy of Fallot. It is expected that the present database developed specifically for CHD could contribute to the biological, and genetic studies and clinical treatment of the disease in the future.
As illustrated in Fig. 1, we initiated the process by analyzing and collecting relevant literature, integrating the identified requirements. Subsequently, we conducted searches and downloads from the PubMed and NCBI databases. The acquired congenital heart disease data underwent basic standardization, followed by thorough analysis and storage. Our established database facilitates users to perform searches, browse, and download data through the website. Additionally, we conduct traffic analysis on the data to understand user preferences and requirements (Fig. 1).
Figure 1: Workflow chart
Simultaneously, we developed an analysis platform that allows users to leverage the database’s existing data or upload personalized data for analysis. Upon completion of the analysis, users are provided with cloud computing results or the required visualization outcomes.
Considering that there is no database of this type available at present, and to collect as much reliable and comprehensive data as possible, we first organized the logic of data collection as follows: firstly, due to the complex causes and symptoms of congenital heart disease, it is mainly divided into four categories, including septal Defects.
obstructive defects, cyanotic defects, and less common types [9]. Secondly, a series of keywords relevant to CHD were listed, for instance, “congenital heart disease”, “patent ductus arteriosus (PDA)”, “atrial septal defect (ASD)”, “ventricular septal defect (VSD)”, “aortopulmonary septal defect”, “pulmonary valve stenosis”, “patent foramen ovale (PFO)”, “tetralogy of fallot (TOF)”. All published articles describing CHD were downloaded and initially screened for those containing sequencing data. Thirdly, the data in the papers were processed, labeled, and standardized. Specifically, articles with reliable content of sequencing data were selected, followed by annotating useful information. For example, annotating different types of CHD including “patent ductus arteriosus (PDA)”, “atrial septal defect (ASD)”, “ventricular septal defect (VSD)”, “aortopulmonary septal defect”, “pulmonary valve stenosis”, “patent foramen ovale (PFO)”, “tetralogy of fallot (TOF)”. Next, preliminary standardization of expression profiles, including gene re-annotation and value transformation, etc., was performed. Finally, after integration, a database was established by categorizing different entries.
After completing the work as described above, we curated a total of 3,559 related publications. Through screening, we excluded papers that did not contain valid information, including those lacking transcriptomic expression profiles, patient clinical data, or those not providing open access to their data. As a result, we obtained over 200 datasets comprising a total of 800 samples. Subsequently, we performed common preprocessing on the data, and applied corresponding methods to different datasets, including batch effect removal, re-annotation, and standardization using gene symbols. For numerical conversions, we utilized common formats such as FPKM, TPM, and RPKM, and performed log transformations (log(x + 0.0001)).
We showcase the limma (Fig. 2), a differential analysis tool. After obtaining the results, users can swiftly generate a heatmap for differentially expressed genes and access image editing features.
Figure 2: Users can perform fundamental bioinformatics tasks such as differential analysis, data standardization, and transcriptome reannotation
3 Analysis and Visualization Tools
To further facilitate scientific research, the platform also provides basic bioinformatics analytical tools for expression profiling data, including standardization tools, variant analysis, heat map plotting, etc. as well as commonly used graphics functions. Our platform allows a direct selection of datasets from the database for analysis and also supports users to upload their own data for analysis. After completing corresponding analyses, common graphics functions such as plotting volcano diagrams are provided. Users are able to use our visualization tools quickly and efficiently to edit images and download required content.
In the analytical tools section we offer, an integration of several prevalent R packages is presented, encompassing DESeq2, edgeR, limma, and RankProd. This amalgamation facilitates users to swiftly conduct standard analyses on RNA-seq data, including normalization and differential analysis. Additionally, the integration of clusterProfiler in our toolkit provides comprehensive enrichment analysis capabilities. As depicted in Fig. 3 our differential analysis tool offers an intuitive interface that guides the user through the necessary steps for data input and analysis parameter selection. Following the completion of the analysis, users are afforded the capability to download the results, which are meticulously compiled into a document. This functionality not only facilitates immediate access and review of the analytical outcomes but also allows for the archival of results for subsequent examination and verification.
Figure 3: Differential analysis tool interface: The screenshot presents the user interface of our web-based differential analysis platform. The left panel displays a list of sample data with corresponding group assignments, here labeled as MP’. The right panel provides options for expression data file input, group comparison selections (case vs. control), and a choice of analytical methods, including limma, rankProd, and t-test. Additionally, users can select the type of log transformation to apply, with options for log2(x) or none. The ‘submit’ button initiates the analysis process
Furthermore, our platform not only allows users to select and analyze data from our database but also permits the upload and analysis of their personalized datasets. This flexibility and range of options aim to enhance user efficiency and adaptability in handling common RNA-seq data analysis tasks, ensuring a streamlined and effective workflow.
Furthermore, our platform is equipped with a suite of visualization tools designed to complement the aforementioned analytical tools. These include heat maps, volcano plots (Fig. 4), and box plots, which are instrumental in showcasing the results of differential analysis, whether derived from standard datasets or user-customized data. Additionally, for the display of enrichment analysis results, we provide bar charts and bubble plots. The platform also features other commonly used graphical representations such as line graphs and radar charts. These visualization tools are integral to our platform, enabling users to effectively interpret and present complex data in a comprehensible and visually appealing manner.
Figure 4: We present the box-plot and volcano-plot drawing tool, where users can customize multiple attributes in the image to achieve their desired visualizations. This includes parameters such as box width, transparency, significance display, outlining, and more. Users can swiftly obtain their preferred image styles, and the boxplot drawing tool offers an export feature, enabling users to obtain high-resolution images
The user-friendly interface of CHDTEPDB enables users to quickly browse, retrieve, and efficiently upload and download and analyze their data. In the browsing function, items could be obtained by selecting datasets, specific types of heart disease, or other features of the dataset; in the retrieval function, desired keywords could be directly entered to perform a global fuzzy search on the available data, thereby realizing a quick acquisition of the information of interest; in the corresponding dataset, data could be rapidly downloaded. Moreover, our database also supports users to upload their own data, which will be displayed in the new version after approval, with an indicated source at the same time. Additionally, commonly used data processing tools for expression profiles and corresponding graphics functions are provided, aiming to improve the efficiency of analysis and research.
All datasets utilized in this study were sourced exclusively from the publicly accessible portions of previously published articles. Rigorous measures were undertaken to ensure that the integration of these datasets does not compromise patient confidentiality or expose any private information. Additionally, clear citations are provided for all data used, facilitating traceability and acknowledgment of original sources. The website hosting these datasets is committed to providing permanent, free access to these resources.
All data in CHDTEPDB are collected from published papers, stored, and managed by MySQL. Web pages and servers are built using Spring Boot. Data processing is written in JAVA and R languages. The URL of the current database is available at http://chdtepdb.com/.
In recent decades, transcriptome expression profiling has played a critical role in various types of diseases [10,11], but the complexity and specificity of preterm heart diseases hinder direct access to the tissue data of the corresponding sample. Despite the fact that some diagnostic and predictive biomarkers have been identified, effectively predicting CHD in the fetal period is still not possible.
The purpose of establishing the present CHDTEPDB is to provide high-quality data for CHD, apart from this, a special category is set up to support the analysis of transcriptome expression profiling data. The current CHDTEPDB platform could also offer up-to-date evidence for researchers.
Continuous advances in CHD and the current imperfection in databases have encouraged the development of the present database platform to record and integrate valuable data relevant to CHD. Overall, CHDTEPDB not only provides integrated data for CHD, but also offers a corresponding computational platform. Such a unique database has the potential to facilitate future research on CHD.
The variety and quantity of various biological data have increased significantly with the development of high-throughput technologies, allowing nearly all researchers to establish their own data from different omics. However, researchers are not always able to fully realize the potential of or apply their data during analysis because of the thresholds in statistics, programming skills, and computer arithmetic as well as the huge data volume and complexity. The uniqueness of CHD is also accompanied by a lack of bioinformatics applications. We aim to create a CHD-centered database platform, gradually incorporating more data from other omics to contribute to the scientific and technological advances in the field of CHD and help researchers achieve more research results.
Acknowledgement: Not applicable.
Funding Statement: This study was funded by Harbin Medical University Outstanding Talent Startup Fund and the Natural Science Foundation of Heilongjiang Province of China (Grant No. LH2020H032). No specific grant number is associated with the funding from Harbin Medical University. Ziguang Song is the host of the projects funded by Harbin Medical University. Jiyi Zhao is the host of the grant from the Natural Science Foundation of Heilongjiang Province of China.
Author Contributions: Jiyi Zha conceived the platform and idea. Weitao Shen completed the technical construction of the platform and the realization of each function and wrote the manuscript. Ziguang Song, Jiangbo Yu are responsible for collecting and collating data. Mengmeng Wang, Chengcheng Wang, Tianyi Lu, Gaojun Shan, Guo Dong, Yiru Wang completed the collection and arrangement of methods and materials for the platform. All authors contributed to the development of CHDTEPDB.
Availability of Data and Materials: All data utilized in this study are derived from open-access datasets published in previous literature. Additionally, CHDTEPDB will continuously provide free data services, including complimentary analysis and plotting tools. These resources are permanently accessible to support further research and replication of our findings.
Ethics Approval: This study was conducted using publicly accessible data from published sources, confirmed as open access. No new human or animal subjects were involved, and the original data collection complied with ethical standards. Therefore, ethical approval was not required. We adhered to all relevant data protection laws and ethical guidelines, ensuring integrity and transparency in our research.
Conflicts of Interest: The authors declare that they have no conflicts of interest to report regarding the present study.
References
1. Sun, R., Liu, M., Lu, L., Zheng, Y., Zhang, P. (2015). Congenital heart disease: Causes, diagnosis, symptoms, and treatments. Cell Biochemistry and Biophysics, 72(3), 857–860. [Google Scholar] [PubMed]
2. Xu, W., Shao, Z., Lou, H., Qi, J., Zhu, J. et al. (2022). Prediction of congenital heart disease for newborns: Comparative analysis of holt-winters exponential smoothing and autoregressive integrated moving average models. BMC Medical Research Methodology, 22(1), 257. [Google Scholar] [PubMed]
3. Mutluer, F. O., Çeliker, A. (2018). General concepts in adult congenital heart disease. Balkan Medical Journal, 35(1), 18–29. [Google Scholar] [PubMed]
4. Yasuhara, J., Garg, V. (2021). Genetics of congenital heart disease: A narrative review of recent advances and clinical implications. Transl Pediatr, 10(9), 2366–2386. [Google Scholar] [PubMed]
5. Yang, A., Alankarage, D., Cuny, H., Ip, E. K. K., Almog, M. et al. (2022). CHDgene: A curated database for congenital heart disease genes. Circulation Genomic and Precision Medicine, 15(3), e003539. [Google Scholar] [PubMed]
6. Burns, K. M., Pemberton, V. L., Pearson, G. D. (2015). The pediatric heart network: Meeting the challenges to multicenter studies in pediatric heart disease. Current Opinion in Pediatrics, 27(5), 548–554. [Google Scholar] [PubMed]
7. Mayer, J. E. (2023). An alternative perspective on the STS congenital heart surgery database (CHSD). The Annals of Thoracic Surgery, 115(2), 296–297. [Google Scholar] [PubMed]
8. Dorobantu, D. M., Sharabiani, M. T. A., Taliotis, D., Parry, A. J., Tulloh, R. M. R. et al. (2020). Age over 35 years is associated with increased mortality after pulmonary valve replacement in repaired tetralogy of Fallot: Results from the UK national congenital heart disease audit database. European Journal of Cardio-Thoracic Surgery, 58(4), 825–831. [Google Scholar] [PubMed]
9. Franklin, R. C. G., Beland, M. J., Colan, S. D., Walters, H. L., Aiello, V. D. et al. (2017). Nomenclature for congenital and paediatric cardiac disease: The international paediatric and congenital cardiac code (IPCCC) and the eleventh iteration of the international classification of diseases (ICD-11). Cardiol Young, 27(10), 1872–1938. [Google Scholar] [PubMed]
10. Hosseini-Gerami, L., Higgins, I. A., Collier, D. A., Laing, E., Evans, D. et al. (2023). Benchmarking causal reasoning algorithms for gene expression-based compound mechanism of action analysis. BMC Bioinformatics, 24(1), 154. [Google Scholar] [PubMed]
11. Hong, M., Tao, S., Zhang, L., Diao, L. T., Huang, X. et al. (2020). RNA sequencing: New technologies and applications in cancer research. Journal of Hematology & Oncology, 13(1), 166. [Google Scholar]
Cite This Article
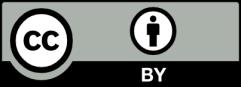
This work is licensed under a Creative Commons Attribution 4.0 International License , which permits unrestricted use, distribution, and reproduction in any medium, provided the original work is properly cited.