Open Access
ARTICLE
Single-cell transcriptomics reveals T-cell heterogeneity and immunomodulatory role of CD4+ T native cells in Candida albicans infection
1 National Engineering Research Center of Immunological Products, Department of Microbiology and Biochemical Pharmacy, College of Pharmacy, Army Medical University, Chongqing, 400038, China
2 Department of Clinical Laboratory, The 980th Hospital of PLA Joint Logistics Support Force (Bethune International Peace Hospital), Shijiazhuang, 050082, China
* Corresponding Authors: QUANMING ZOU. Email: ; HAO ZENG. Email:
# These authors have contributed equally to this work
(This article belongs to the Special Issue: Cell Death and Inflammation in Signaling and Diseases)
BIOCELL 2024, 48(9), 1355-1368. https://doi.org/10.32604/biocell.2024.051383
Received 04 March 2024; Accepted 12 June 2024; Issue published 04 September 2024
Abstract
Objective: Candida albicans is a common fungal pathogen that triggers complex host defense mechanisms, including coordinated innate and adaptive immune responses, to neutralize invading fungi effectively. Exploring the immune microenvironment has the potential to inform the development of therapeutic strategies for fungal infections. Methods: The study analyzed individual immune cell profiles in peripheral blood mononuclear cells from Candida albicans-infected mice and healthy control mice using single-cell transcriptomics, fluorescence quantitative PCR, and Western blotting. We investigated intergroup differences in the dynamics of immune cell subpopulation infiltration, pathway enrichment, and differentiation during Candida albicans infection. Results: Our findings indicate that infiltration of CD4+ naive cells, regulatory T (Treg) cells, and Microtubules (MT)-associated cells increased after infection, along with impaired T cell activity. Notably, CD4+ T cells and plasma cells were enhanced after infection, suggesting that antibody production is dependent on T cells. In addition, we screened 6 hub genes, transcription factor forkhead box protein 3(Foxp3), cytotoxic T-lymphocyte associated protein 4 (CTLA4), Interleukin 2 Receptor Subunit Beta (Il2rb), Cd28, C-C Motif Chemokine Ligand 5 (Ccl5), and Cd27 for alterations associated with CD4+ T cell differentiation. Conclusions: These results provide a comprehensive immunological landscape of the mechanisms of Candida albicans infection and greatly advance our understanding of adaptive immunity in fungal infections.Keywords
List of Abbreviations
APCs | Antigen-presenting cells |
Ccl5 | C-C Motif Chemokine Ligand 5 |
CCR5 | CC chemokine receptor 5 |
CTLA4 | Cytotoxic T-lymphocyte antigen 4 |
CXCR4 | C-X-C chemokine receptor type 4 |
DCs | Dendritic cells |
Eno1 | Enolase 1 |
Foxp3 | Transcription factor forkhead box protein 3 |
GEM | Gel Bead-in-Emulsion |
GSEA | Gene set enrichment analysis |
HAVCR2 | Hepatitis A virus cellular receptor 2;HCV, hepatitis C virus |
IFN-γ | Interferon-γ |
Il2rb | Interleukin 2 Receptor Subunit Beta |
LAG3 | lymphocyte activation gene-3; MT-associated, Microtubules-associated |
MAPK | Mitogen activated protein kinase |
NES | Normalized enrichment score |
PBMCs | Peripheral blood mononuclear cells |
PCA | Principal component analysis |
PRRs | Pattern recognition receptors |
scRNA-seq | Single-cell RNA sequencing |
Treg | Regulatory T |
TLR | Toll-like receptor |
TNF | Tumor necrosis factor |
UMAP | Uniform flow approximation and projection |
Blood-borne infections are presumed to result from intracellular changes in tissues induced by external injury, changes in intercellular communication, cellular heterogeneity, and structural imbalances. Specific immune subpopulations must expand dramatically during infection to effectively neutralize the pathogen threat. Significant expansion of antigen-specific T cells, which may increase more than a thousand-fold, reflects the adaptive capacity of the immune system in response to threats [1–4]. From acute or chronic infections to vaccinations, cancers, and autoimmune diseases, the balance between T-cell memory development and immunoregulatory mechanisms, including T-cell effector depletion and regulatory T-cell suppression, ultimately determines the outcome of protection or susceptibility [5,6].
Candida albicans (C. albicans) is an immunosuppressed host-specific pathogenic commensal fungus that colonizes vaginal and gastrointestinal mucosa without causing significant disease. The interaction of C. albicans with the host immune system significantly impacts infection, which has led to increased research into triggering immune defense mechanisms [7]. A subtle interplay between the innate and adaptive immune responses underpins host defense against C. albicans. At its core is the adaptive T-cell response, which is essential for effective defense against C. albicans infection and maintenance of tissue.
There has been considerable evidence of an interaction between C. albicans infection and the immune response. C. albicans can cause cutaneous mucosal and systemic infections. It induces macrophage lysis through pyroptosis. Wang et al. developed a decrease in CD8+ T-cells in oral tumor tissues induced by C. albicans infection, whereas IL-17A+ CD4+ T cells and IL-17A+ γδ T cells increased [6]. Helper T-cell-mediated T-cell reactivity plays a key role in host clearance of fungi by secreting pro-inflammatory cytokines [8]. Enolase 1 (Eno1), a fungal parthenogenetic protein in C. albicans, has immunostimulatory activity and directly binds to human and mouse B cells, inducing their proliferation by up-regulating the expression of CD86 in B cells [9]. Various evidence points to an important effect of C. albicans infection on immune cells and a close interaction with the immune response [8–11].
Following infection, T cells differentiate to give rise to distinct subpopulations of effector T cells that mediate pathogen clearance. These effector T cells persist and develop into memory T cells, resulting in durable immunity. For example, CD4+ T cells of the T cell subsets play a crucial role in the generation of protective memory CD8+ T cells following infection or immunization [12]. There are gaps in understanding the relationship between blood-borne infections and alterations in T lymphocyte subsets.
In a previous cohort study, which included 25 patients infected with HIV through the bloodstream, the authors found that the decline in naïve CD4+ T-cell counts was more pronounced than the memory decline in CD4+ T cells during follow-up, suggesting an important role for naïve CD4+ T-cell counts in the maintenance of CD4+ T-cell homeostasis [13]. Specialized antigen-presenting cells (APCs) present virus-derived peptides on MHC class II molecules to naive CD4+ T cells and deliver co-stimulatory signals that drive T cell activation, facilitating viral clearance. In summary, CD4+ naive T cells play an important role in anti-infection through immune response, promotion of inflammatory response, and immunomodulation. Further studies can deeply explore the functions and regulatory mechanisms of CD4+ naïve T cells and provide a theoretical basis for developing relevant therapeutic strategies.
To understand the intricate immunological changes induced by C. albicans infection in the peripheral blood, researchers employed a mouse model of systemic C. albicans infection using single-cell RNA sequencing (scRNA-seq) technology. This study focused primarily on analyzing the heterogeneity of T-cell subpopulations. Investigations revealed dynamic immune processes, highlighting alterations in T-cell differentiation and cellular communication between T-cell subpopulations. In addition, genes associated with C. albicans bloodstream infection were screened. This was done to comprehensively understand the heterogeneous immune landscape in the Candida albicans-infected mouse model.
Expression of the immunosuppressive checkpoints hepatitis A virus cellular receptor 2 (HAVCR2) and lymphocyte activation gene-3 (LAG3) during C. albicans stimulation of peripheral blood mononuclear cells (PBMCs) was demonstrated by scRNA-seq analysis. Further understanding of the topology of immunological checkpoints in fungal infections has the potential to reveal novel therapeutic targets for C. albicans infections [14]. In addition, scRNA-seq analyses revealed a significant increase in the number and strength of interactions between infiltrating immune cells and adrenal resident cells following systemic C. albicans infection [15]. Although advances in scRNA-seq technology have deepened our understanding of C. albicans infections, the influence of various factors affecting the outcome of the final immune response, including host inflammatory responses, T-cell subpopulation heterogeneity, state of differentiation, and immune-cell interactions, remains unclear and needs to be further explored.
Currently, culture methods are commonly used in clinical trials to detect pathogenic fungi, including Candida [16]. Three strains of C. albicans SC5314 were inoculated onto a solid medium (CHROMagar BD, 212961, Beijing, China) and incubated at 37°C for 24–48 h. One green colony was selected and mixed thoroughly with sterile saline. The fungal cells were counted under a microscope (Olympus Corporation, BX53, Tokyo, Japan), and the concentration was adjusted to 1 × 107 CFU for each mouse.
Nine 8-week-old inbred Balb/c female mice weighing 20 ± 1 g were randomized into three groups (HFK Bioscience, Beijing, China).100 μL of C. albicans suspension was injected into the tail vein of the sample. Each mouse in the experimental group was injected with 1 × 106 CFU C. albicans in the tail vein, and the control group was injected with saline. Mice in both experimental groups were executed after 24 and 48 h C. albicans infection, respectively. Then blood was removed, and spleen single cells were collected for isolation. The study protocol was approved by the Ethics Committee of the 980th Hospital of the Joint Logistics Support Force of the Chinese People’s Liberation Army (2022-KY-129) and followed the principles of the Declaration of Helsinki.
Preparation of single-cell suspensions
Single-cell RNA sequencing (scRNA-seq) requires the preparation of highly active single-cell suspensions to obtain reliable sequencing results [17]. We found that the immune response was at its highest at 48 h after C. albicans infection, so the 3 samples in the group and 3 healthy samples were chosen for subsequent single-cell sequencing. A 50 mL centrifuge tube containing a 70 μm cell sieve is wetted with 3 mL of Hank’s solution (Sigma-Aldrich, SM-2001-C, 100 mL, Shanghai, China). The spleen tissue is placed in the sieve, gently crushed with a 5 mL sterile syringe (Kang Long, 0142, 5 mL, Guangzhou, China), and rinsed with sterile Hank’s solution to ensure complete release of the cells. After centrifugation at 300 g for 10 min, the supernatant is discarded, and the cells are resuspended with 1 mL of Hank’s solution. Take 20 μL, add 980 μL PBS (Macklin, P903593-500 ml, 50 mmol/L pH7.4, Shanghai, China), count under the microscope (Olympus Corporation, BX53, Tokyo, Japan), and adjust the cell concentration to 1 × 107 /mL with Hank’s solution.
To avoid the lymphocyte isolate interfering with neutrophil fractionation, 100 μL was set aside in a separate tube for staining. The remaining cells after PBMC isolation were analyzed in tubes, centrifuged, and re-suspended in 2 mL of Hank’s solution. Then, we added 3 mL of lymphocyte isolate to a sterile centrifuge tube (Beyotime, FCT151, 5 mL, Shanghai, China). Slowly add the cell suspension along the wall of the tube and centrifuge (Sigma-Aldrich, Z654736GB-1EA, St. Louis, MO, USA) for 15 min (2000 r/min). Carefully aspirate the second circular layer of creamy white lymphocytes and transfer to 5 mL of Hank’s solution, centrifuge at 1500 rpm for 20 min, wash twice, resuspend the cells in 1 mL of culture medium, and count under the microscope to a concentration of 1 × 107/mL.
By analyzing gene expression in individual cells, single-cell RNA sequencing (scRNA-seq) can address cellular heterogeneity and cell-type gene expression dynamics. 15 Cell counts are performed on single-cell solutions to ensure that the cell concentration is in accordance with the Chromium Single Cell 3’s Reagent v3 Kit (Illumina, MS-102-3003, 600T, San Diego, CA, USA). Observe the morphology and activity of the cells by microscopy to ensure that the cells used for the experiment are of good quality. Ensure that all manipulations are performed under sterile conditions [18]. Chromium Single Cell 3 Reagent v3 Kit For preparation of scRNA-seq libraries. Single-cell solutions are placed on the Chromium Single Cell Controller to generate single-cell latex beads (GEM).
In this study, the R software package Seurat (v4.1.0) was used to import and process scRNA-seq data [19]. Data processing included read-in, data quality control, gene and cell filtering, data normalization, identification of highly variable genes, data scaling, principal component analysis (PCA), and the uniform flow approximation and projection (UMAP) algorithm. Unless otherwise noted, Seurat runs with default parameters. First, a Seurat object is constructed using the CreateSeuratObject function, excluding poor quality cells-retaining cells where at least 200 genes are found and expressed in at least 3 cells. Subsequently, low-quality cells are further culled based on sample characteristics, the number of genes detected, mitochondrial ratio, and the total number of molecules detected. The data was then normalized using the “LogNormalize” method of the NormalizeData function with a scaling factor of 10,000. Subsequently, the FindVariableFeatures function identified 4000 highly variable genes for subsequent analysis. The RunPCA function scaled the data to select the top 50 genes. The RunPCA function scaled the data and selected the top 50 PCs for downstream analysis. Finally, the UMAP algorithm downscaled the cells.
We loaded the previously obtained summary data into Monocle objects with default parameters. Monocle2 is an R package for single-cell RNA sequencing data analysis that provides powerful tools to identify and analyze cellular states. Variable genes contributing to the proposed time trajectory were identified by Monocle2 analysis. Cells were aligned to the proposed time trajectory based on the combination of highly variable genes obtained from all cells [20]. This alignment process can help researchers understand how cells develop along a continuous trajectory and which changes in gene expression are significant at different points in time.
Differential genes were defined as genes with a fold change greater than 0.25 compared to other cells and with more than 25% expression. The study performed functional annotation of differential genes using the open web application EnrichR (https://maayanlab.cloud/Enrichr/, accessed on 10 May 2024) to identify potential functions in which the above differential genes may be involved [21]. Gene set enrichment analysis (GSEA) of transition-state cells was performed using the gseGO function in the R package clusterProfiler (v4.2.2) [22] and signaling pathways with NES > 0 and FDR-corrected significance p < 0.05 were defined as activated pathways and inflammation-related pathways were visualized using the R package GseaVis (v0.0.1). In this study, the FindMarkers function (v4.1.1) of the Seurat R software package identified characteristic genes for the indicated cell populations. The differential genes had a logarithmic change of greater than 0.25 compared to other cells and were expressed in more than 25% of the cells.
Analysis of cell-cell interactions
Load expression data for individual cells and corresponding cell type information. Calculate and infer intercellular communication networks and identify biologically meaningful intercellular communications by assigning occurrence probabilities and alignment tests to communicate pairs. Systematically analyze cellular communication networks to determine the extent to which input and output signals contribute in a given cell type. The pre-processed individual cell expression data was loaded into the analysis software to identify the cell type to which each cell belongs. Using algorithms in the software, the likelihood of intercellular communication is calculated based on the expression data. By comparing the expression patterns between different cell types, possible interactions between cells are inferred.
RT-qPCR was implemented according to previous procedures [23]. The 3 samples from the experimental group and 3 healthy samples control group were collected to extract RNA. RNA was extracted using the EZ-10 Total RNA Small Volume Extraction Kit (BBI, B610423-0100, 100PREPS, Shanghai, China). 20 μL of the reaction system was used for one-step qPCR of Ctla4, Ccl5, IL2rb, and GAPDH using the ABScript II One Step SYBR Green RT-qPCR Kit (Abclonal, RK20404, 100RXN, Beijing, China). The primers were demonstrated in Table 1.
Protein levels can be detected by Western blotting [24]. Add 100 μL of cell lysate (Bioleaper, BR2003801, 100 mL, Shanghai, China) to selected CD4+ T cells. centrifuge at 12,000 g for 5 min, aspirate the supernatant and determine the protein concentration by BCA (SALMART, BCA, 1 g, Shenzhen, China). The sample volume was adjusted according to the protein concentration and 15 μg of protein was injected into each lane. Electrophoresis is performed using a 12.5% separator gel at 150 V and a constant voltage of 100 V for 90 min. Transfer the membrane at a constant pressure of 100 V for 90 min. Membranes were blocked with 5% skimmed milk for 60 min and washed three times with TBST (Servicebio, G0004, Wuhan, China) for 5 min each time. Incubate overnight at 4°C with rabbit anti-IL2 receptor beta antibody (1:1000 dilution, bs-1959R, Bioss, Beijing, China), rabbit anti-RANTES antibody (1:1000 dilution, bsm-54125R, Bioss, Beijing, China), CTLA4 rabbit pAb (1:1000 dilution, A13965, Abclonal, Wuhan, China), and beta-actin mouse mAb (1:2000 dilution, AC004, Abclonal, Wuhan, China). After three washes with TBST for 5 min each, HRP goat anti-rabbit IgG (H+L, AS014) or HRP goat anti-mouse IgG (H+L) (1:2000 dilution, AS003, Abclonal, Wuhan, China) was added and incubated for 90 min at room temperature. Rinse three times with TBST for 5 min each time. Automated exposure was performed using the SuperSignal™ West Pico PLUS kit (34577, Thermo Fisher, Waltham, MA, USA).
For data comparisons between two different study groups, we used an unpaired two-sided Student’s t-test, each experiment was repeated 3 times. In this study, all results with p < 0.05 were considered statistically significant. These p-values are indicated in the graphs and legends by symbols at different levels such as *p < 0.05. All statistical analyses were done through GraphPad Prism 8 (GraphPad Software, La Jolla, CA, USA).
Single-cell sequencing of immune cells in C. albicans contaminated tissues
We performed scRNA-seq to analyze immune cells in tissues infected with C. albicans. Cells were classified into 29 clusters (Fig. 1A), which were categorized into 9 cell types, namely B cells (Cd79a, Cd79b), basophil (Fcer1a, Itga2), dendritic cells (Flt3), erythroblast (Car2, Hba-a2), macrophage (Adger1, Cd68, Mrc1), monocyte (Csf1r, Itgam), neutrophil (Csf3r, Cxcr2), natural killer cells (Nkg7, Klrd1), and T cells (Cd3d) (Fig. 1B–D). The proportion of B cells and neutrophils was higher in the infected samples, while the proportion of T cells was lower (Fig. 1E).
Figure 1: Single-cell atlas of immune cells in infected tissues. (A, B) Cell populations from the infected and control group. (C) Tissue sections of different cell populations from patients and healthy individuals. (D) Clustering annotated cell markers. (E) Percentage of cell types in the screening dataset.
Single-cell mapping of T cells in C. albicans infected tissues
To understand changes in T cells during the infection event, we annotated the T cell population into 9 subpopulations, including CD4+ memory T cells, CD4+ naive T cells, CD8+ cytotoxic T cells, CD8+ memory T cells, CD8+ naive T cells, MT-related T cells, Th1, Th17 and Treg (Fig. 2A). In the case of systemic infection with C. albicans, the proportions of most of the cell types were significantly altered compared to the control group (Fig. 2B,C). In addition, the proportion of CD4+ memory T cells remained largely unchanged, whereas CD4+ naive T cells were significantly reduced in infected samples (Fig. 2D). CD4+ naive T cells are the starting point of the adaptive immune response, and their recognition of and response to antigens is a critical step in initiating the subsequent immune response. CD4+ naive T cells help maintain the immune response’s balance, preventing excessive inflammatory responses. Therefore, we speculate that CD4+ naive T cells play an important role in resistance to C. albicans infection.
Figure 2: Single-cell profiles of T cells in infected tissues single-cell profiles of T cells in infected tissues with T cells reclustered into subpopulations such as CD4+/CD8+ (A) UMAP plots of annotated T cell subpopulations in the 6 samples. (B) UMAP plots of the distribution of various T cell subpopulations in the control and experimental groups (C) Dot plots of the expression of 9 T cell subpopulation-specific marker genes. mean Exp: average expression; percent of Exp: percentage of cells expressing the gene (D) Proportion of cells in the 9 T cell subpopulations in the 6 samples and the group in which the samples are located.
T-cell subpopulation activation pathways contribute to dynamic immune responses
Due to the significant difference in the proportion of CD4+ naive T cells between the infected and healthy groups (Fig. 3A), we performed GSEA on CD4+ naive T cells, which showed significant down-regulation of the pathways of antigen processing and presentation, chemokine signaling, Mitogen activated protein kinase (MAPK) signaling, and T-cell receptor signaling (Fig. 3B–E). The synergistic role of thymic fibroblasts, B cells, and dendritic cells in antigen presentation, selection, and regulatory T-cell development is critical for maintaining a tightly regulated immune response. It has been found that CD8+ naive T cells can be induced by either specialized antigen-presenting cells or by direct antigen presentation or antigen cross-presentation [25,26]. The downregulation of antigen processing and presentation pathways may lead to increased immune tolerance. This means that the immune system has a reduced ability to recognize and respond to self-antigens or antigens of infected pathogens, which may result in the immune system being unable to clear infections or attacks on tissues effectively. Chemokine signaling is an important regulator of T cell activation and proliferation, and down-regulation of chemokine signaling may result in impaired recognition and transmission of chemokine signals by T cells, which in turn may affect T cell activation and proliferative responses.
Figure 3: CD4+ naive T cell GSEA pathway enrichment analysis (A)Volcanic plot of differentially expressed genes of CD4+ naive T cells between the infected and control group (B) Antigen entry and presentation (C) Chemodynamic signaling pathway (D) MAPK signaling pathway (E) Signalling pathway of T cell receptor.
The MAPK signaling pathway (Table 2) is crucial in the C. albicans infection process. The p38 MAPK signaling pathway has been found to play a crucial regulatory role in the beneficial effects observed after treatment of Candida albicans-infected nematodes with thymol [27]. The peptide toxin candidalysin, secreted by C. albicans, damages epithelial cells and drives innate inflammatory responses mediated by the MAPK pathway. The fungal pathogen Candida albicans secretes the peptide toxin candidalysin, which damages epithelial cells and drives innate inflammatory responses mediated by the epidermal growth factor receptor (EGFR) and MAPK pathways, as well as the transcription factor c-Fos. Down-regulation of the MAPK signaling pathway and the T cell receptor signaling pathway, which play a key role in T cell activation and differentiation, may lead to the down-regulation of T cell responses to external stimuli. Down-regulation of the MAPK signaling pathway and T cell receptor signaling pathway plays a key role in T cell activation and differentiation, and down-regulation of these pathways may lead to a decrease in the ability of T cells to respond to external stimuli, thus affecting T cell activation and differentiation [27,28]. MAPK signaling pathway and T cell receptor signaling pathway play key roles in T cell activation and differentiation, and down-regulation of these pathways may result in reduced T cell responsiveness to external stimuli, affecting T cell activation and differentiation. In summary, infection may inhibit the activation and immune response function of CD4+ T naive cells and affect T cell differentiation. The MAPK signaling pathway is involved in regulating various biological processes such as cell proliferation, differentiation, survival, and inflammation. With the downregulation of the MAPK signaling pathway, the immune cells may produce fewer inflammatory mediators, such as cytokines and chemokines, upon activation. This may lead to reduced activation levels of immune cells, diminishing their ability to fight fungal infections.
EnrichmentScore, NES and p-value of the KEGG MAPK SIGNALING PATHWAY shown in the table can be seen to play a key role in Candida albicans infection.
CD4+ naive T cells differentiated towards treg and MT-related T after infection
We performed trajectory analyses to further explore the relationship between dynamic immune response processes and T cell differentiation. We dissected population heterogeneity and reconstructed the reprogramming process using gene expression profiling data. The Monocle 2 algorithm, which is based on transcriptional similarity, was employed for pseudo-temporal cell sorting [20]. For the experimental group, we identified CD4+ memory T cells and CD4+ naive T cells as the intermediate transition state, and finally reached MT-associated T cells, Th1, Th17, and Treg cells terminal differentiation state (Fig. 4A–C), which is also consistent with earlier findings. CD4+ naive differentiated more towards Treg and MT-related T after C. albicans infection. We also examined the gene dynamics of the proposed chronology, with a heatmap highlighting the top 50 genes that underwent dynamic changes (Fig. 4D) and identifying three distinct transformation patterns. Next, we constructed a proposed chronological trajectory for the control group using genes that were highly expressed in the progression of T-cell subpopulations, and the results were consistent with the differentiation status of the experimental group (Fig. 4E–G). To understand the dynamics of gene expression, changes in key genes such as Ccr7, Sell, Ctla4, Cd52, mt-Rnr2 and mt-Cytb were analyzed (Fig. 4H). During Treg differentiation, Sell, a marker gene for CD4+ naive T cells, showed down-regulation, whereas Ctla4, a marker gene for Treg cells, showed up-regulation during the same process. These results suggest CD4+ naive differentiation towards Treg and MT-associated T cells after C. albicans infection.
Figure 4: Differences in marker genes, highly expressed genes and key genes for T cell subpopulation progression in experimental groups in different subpopulations and groups. (A–C) Unsupervised transcriptional tracking of T cell subpopulations based on marker genes for T cell subpopulation progression using cell state and subpopulation colouring by Monocle (3rd ed.). (D) Heatmap showing the expression of typical genes associated with T cell subpopulation differentiation in individual cells. The colour key from blue to red and from low to high shows the relative expression levels. (E–G) Unsupervised transcriptional tracking of T cell subpopulations using Monocle (version 2), with highly expressed genes identified based on the progression of T cell subpopulations, coloured by cell state and subpopulation. (H) Characterisation and expression dynamics of key genes.
The role of intercellular communication of T cell subsets in the dynamic immune response to disease
Cell-cell interactions between immune cells play a crucial role in immune outcomes. We calculated cell-cell interaction scores using CellChatDB (http://www.cellchat.org/, accessed on 10 May 2024) to analyze ligand-receptor interactions between various cell types. We observed interactions between 12 cell types in the control and infected groups (Fig. 5A,B). Notably, the cell-cell junctions in the infected group were significantly different from those in the control group (Fig. 5C,D). Interactions between neutrophils and other cells were reduced in the infected group, and interactions between plasma cells and other cells were increased in the infected group. We also investigated the interaction of specific ligand-receptor pairs between different cell populations. Ligand-receptor pairs of CD4+ Native cells with plasma cells (PTPRC-SEMA4D, PTPRC-MRC1, CD47-SIRB1) were significantly upregulated in the experimental group compared to the control group, suggesting that these pathways are essential for the anti-infection immune response (Fig. 5E,F).
Figure 5: (A) Heatmap of cellular connections induced by a single signalling pathway or ligand receptor in the infected group. (B) Heatmap of cellular interactions mediated by a single signalling pathway or ligand receptor in the control group. (C) Mutual intensity/probability plot of twelve cells in the infected group. (D) Mutual intensity and probability plots for twelve different cell types in the control group. (E) Contribution of all ligand-receptor signaling pathways in the infected group. (F) Contribution of each ligand-receptor to each signaling pathway in the control group.
Compared to the control group, CD4+ naive T cells showed intercellular interactions on the MIF-TNFRSF10D, MIF-TNFRSF14, and HLA-E-KLRK1 ligand receptor pairs. TNFRSF10D, TNFRSF14 are genes belonging to the tumor necrosis factor (TNF) family, which have immune-activating effects, and it has been reported that TNF family genes are involved in the control of HCV infections. KLRK1, also known as the NKG2D gene, once bound to its ligands, activates NK cells and CD4+ T cells, which are motivated to mount an immune response and kill the infected cells. Cellular communication between MT-associated T cells and ICAM1-SPN and ICAM1-ITGAL ligand receptor pairs also emerged (Fig. 5E,F). This suggests that CD4+ naive T cells, MT-related T cells play an important role in the fight against fungal infection link.
Hub gene regulation of T cell differentiation
The protein-protein interaction (PPI) network of differential genes in the CD4+ T naive cell mimetic timing analysis was constructed with the help of the STRING (Search Tool for the Retrieval of Interacting Genes/Proteins, https://cn.string-db.org/, accessed on 10 May 2024) database (Fig. 6A). The interaction score of the PPI network was set to >0.4. To investigate their biological significance, we performed GO and KEGG enrichment analyses of these differential genes (Fig. 6B). Significant enrichment of functions such as T cell receptor signaling pathway, Th1 and Th2 cell differentiation, Th17 cell differentiation, and cytokine-cytokine receptor interaction were observed (Fig. 6B). We found that they were involved in biological processes such as immune response, T cell differentiation, apoptosis, cellular components such as biofilm, and molecular functions such as protein interactions, suggesting that these differential genes regulated T cell differentiation, KEGG pathway including cytokine-cytokine receptor interaction, autoimmune, cell adhesion molecules, and T17 cell differentiation. We selected six genes with high connectivity in the gene expression network as hub genes, including Foxp3, Ctla4, Il2rb, Cd28, Ccl5, and Cd27. Fig. 6C shows that the expression level of hub genes was higher in the infected group than in the control group. mRNA and Western blotting showed that the expression of the 6 hub genes was significantly increased at protein levels after infection (Fig. 6D,E), revealing the infection-relevant properties of hub genes.
Figure 6: (A) Differential genes in the proposed time series for constructing the PPI network; (B) HUB gene functions shown in KEGG/GO data. p-values for selected gene ontology concepts are less than 0.05 (one-sided Fisher’s exact test) and corrected by Benjamini-Hochberg. (C) Violin plots of HUB gene expression in two groups of T cells. (D) mRNA expression of hub genes. (E) Protein expression of hub genes. ns, not significant, *p < 0.05, **p < 0.01, ***p < 0.001.
Several previous studies have demonstrated that CD4+ T cells play an important role in the quorum immune response to Candida albicans infection. Hernández-Santos et al. found that the interaction of human CD4+ T cells with C. albicans enolase 1 modulated the response of host CD4+ T cells to the fungus and that the enzyme-mediated immune evasion not only by interfering with complement regulating factors but also by directly modulating the CD4+ T cell response mediates immune evasion [29]. Pandiyan et al. found that in a mouse model of Candida albicans Th17 cell infection, CD4+CD25+Foxp3+ regulatory T cells promote Th17 cells and enhance host resistance in vitro [30]. However, these studies do not provide a comprehensive immunological picture of the mechanisms of Candida albicans infection, and the mechanisms of adaptive immunity in fungal infections have not been thoroughly explored. The heterogeneity of T cell subsets and the presence of immunomodulatory factors in C. albicans infection were demonstrated using scRNA-seq. These findings deepen our understanding of the immune mechanisms of C. albicans infection and have the potential to facilitate the development of new therapeutic technologies and identify new targets for the treatment of C. albicans infection.
We did single-cell sequencing of immune cells in C. albicans-contaminated tissues and further classified T cells into nine subpopulations. We found that the proportion of CD4+ memory T cells remained essentially unchanged in the infected samples, whereas the proportion of CD4+ naive T cells was significantly reduced, and we hypothesized that CD4+ naive T cells play an important role in the resistance to C. albicans infection. We found significant down-regulation of the pathways of antigen processing and presentation, chemokine signaling, MAPK signaling, and T cell receptor signaling by GSEA analysis. Further trajectory analysis of CD4+ naive T cells revealed differentiation towards Treg and MT-associated T after infection. We calculated cell-cell interaction scores using CellChatDB and identified important anti-infection immune response pathways (PTPRC-SEMA4D, PTPRC-MRC1, CD47-SIRB1). Using the STRING database, we constructed a PPI network of differential genes in the simulated time analysis of CD4+ T naive cells, and we identified six hub genes with infection-related properties, including Foxp3, Ctla4, Il2rb, Cd28, Ccl5, and Cd27.
Decoding the immune cell profile in C. albicans infection identified 29 cell clusters, further classified into nine distinct populations. Depletion of T cells mediated antifungal protection in infections [29], which provides clues to understanding the critical role of T cells in the fight against C. albicans. Studies of T cell heterogeneity in infected histopathological samples have included clustering T cells into CD4+ and CD8+ subpopulations under healthy and diseased conditions, documenting changes in immune cell subpopulations. This study emphasized that a pattern of CD4+/CD8+ cell ratios was visible in infected individuals compared to healthy controls. This study also highlights the presence of fungal-reactive T cells in the healthy population, which are regulated during episodes of invasive fungal disease and allergic reactions [30,31], suggesting profound changes in the immune system of certain infected individuals associated with disease progression.
Meanwhile, CD4+ naive T cells were significantly reduced in infected samples. Infection may inhibit the activation and immune response function of CD4+ T naive cells and affect T cell differentiation. We performed GSEA on CD4+ naive T cells, which showed significant down-regulation of antigen processing and presentation pathways, chemokine signaling, MAPK signaling, and T cell receptor signaling. Down-regulation of these pathways reduces the ability of the immune system to respond to antigens from infected pathogens, i.e., after the occurrence of C. albicans infection, the proportion of CD4+ T naive in infected samples is significantly reduced, and the immune function of the CD4+ T naive cells is hampered. Traditionally, CD4+ T cells present antigens to induce cytotoxicity and memory CD8+ T cell responses, which defend against infection. As mentioned in a COVID-19 study, the proportion of cell taxa in an inactivated state was significantly decreased in patients with COVID-19 compared to the healthy group, such as subpopulations of CD4+ naive, CD4+ memory, CD4+ effector memory, Treg [32]. Even during the recovery period, the CD4+ naive, CD8+ naive, and Treg cell subsets did not return to the levels found in healthy individuals, and these studies demonstrate the importance of CD4+ T naive cells for anti-infective immune responses.
CD4+ naive differentiated more towards Treg and Mt-related T after C. albicans infection. Studies of respiratory fungal infections have shown that CD4+ T cells progressively differentiate in lymph nodes. Toll-like receptor (TLR) mediated signaling promotes interferon-γ (IFN-γ) production, whereas MYD88-independent signaling promotes the differentiation of Th1 cells in the lung [33]. Trajectory analyses of T-cell subsets have explored the relationship between immune alterations and T-cell differentiation. CD8+ memory, cytotoxic and naive T cells transition to CD4+ memory and naive T cells shortly after formation. These subpopulations differentiate into MT-associated T cells, Th1, Th17, and Treg cells. While Th2-related immunity may be deleterious, Th-related immune responses protect against fungi [34,35]. Treg cells inhibit overreactivity but may hinder protective immunity, allowing fungi to evade the immune system [36,37]. The development of antifungal Th1 responses has been associated with various fungal infections, which provides new avenues for antifungal therapy.
Ligand receptor pairs (PTPRC-SEMA4D, PTPRC-MRC1, CD47-SIRB1) of CD4+ Native cells with plasma cells were significantly upregulated in the experimental group compared to controls, suggesting that these pathways are critical for the anti-infection immune response. Intercellular interactions are dynamic under different pathological conditions and are key in shaping the immune response. Antifungal immune responses involve neutrophil pattern recognition receptors (PRRs), signal transduction pathways, and cytotoxic mechanisms [19,38,39]. Cytokine production and T-cell polarisation are essential for IL17/IL17 receptor-dependent antifungal immune responses. Dectin-1 on monocytes and Dendritic cells (DCs) is essential [40,41]. Our study found that intercellular interactions were altered in the infected group, with a decrease in neutrophil association. Expression of ICAM1-ITGAL and ICAM1-SPN, which mediate neutrophil interactions, was reduced. Bone marrow-produced neutrophils are released for in vivo patrolling [42,43]. Neutrophils detect activated endothelium and inflammatory tissue, while local sentinel cells release pro-inflammatory chemicals upon recognizing danger signals [44]. These signals stimulate endothelial cells, upregulate chemokines, and enhance the expression of adhesion molecules [21,43]. During C. albicans infection, host immune cells enhance cell-cell interactions against pathogens through ligand-receptor pairing.
This study identifies key genes associated with cell differentiation. Previous studies have shown that Candida-driven dendritic cell maturation is associated with reduced expression of CCR5 (CC chemokine receptor 5) and increased expression of CXCR4 (C-X-C chemokine receptor type 4) and CCR7, suggesting that dendritic cells co-localize with T-cells when they encounter Candida in lymphoid organs [22,45]. The co-stimulatory signals mediating the transition from Th0 to Th17 are different from those mediating the transition from Th1. Previous studies, consistent with our findings, have reported that CTLA-4 inhibits Th17 differentiation [46]. Our data suggest that Ccr7, Sell, and Ctla4 contribute to immune regulation and may influence infection-related changes in the local tissue environment.
A study related to sepsis immunity proposed that IL2RB ameliorates sepsis-induced immune dysfunction and that IL2RB also balances the Th1/Th2 response and blocks Th17 activation [47]. Cytotoxic T-lymphocyte antigen 4 (CTLA4) is upregulated in immune cells during acute infections and may act as an immune checkpoint. During chronic hepatitis C virus (HCV) infection, CCL5 promotes the induction of immune cell chemotaxis, and, significantly, CCL5 is involved in establishing the T-helper 1 response critical for the control of liver disease and the outcome of HCV infection. Previous studies are consistent with our findings that Il2rb, Ctla4, and Ccl5 contribute to immune regulation and may influence infection-related changes in the local tissue environment and that these genes are precisely the key genes identified in our study as being associated with cellular differentiation [47–49].
From a clinical perspective, the study of CD4+ Naive T cells and their related hub genes can help deepen the understanding of the mechanisms of immune response regulation and provide a basis for developing new therapeutic strategies. For example, by intervening in the activation and differentiation process of CD4+ naive T cells, the strength and direction of the immune response can be regulated, thereby improving the ability to resist infection. In addition, by identifying and utilizing specific hub genes of CD4+ naive T cells, more precise diagnostic methods and individualized treatment plans can be developed, providing more effective means for clinical treatment. Moreover, understanding the differentiation of CD4+ naive T cells during infection can help design more effective vaccines. By targeting specific hub genes in these cells for vaccine design, the immune system can be better triggered to generate a protective immune response against the infection, increasing the effectiveness and coverage of the vaccine.
In conclusion, this study reveals the immune landscape of C. albicans infection, providing a detailed characterization of T cells, including clustering, dynamics, developmental trajectories, and unique features. We found an increased proportion of B cells and neutrophils in the infected group and a decreased proportion of T cells, especially CD4+ naive T cells. Further analysis showed that CD4+ naive T cells differentiated toward Treg and MT-associated T cells after infection, whereas the proportion of CD4+ memory T cells remained unchanged. GSEA analysis revealed that down-regulation of the pathways of antigen processing and presentation, chemokine signaling, MAPK signaling, and T-cell receptor signaling during infection may lead to increased immune tolerance and decreased T-cell activation capacity. In addition, analysis of cell-cell interactions suggests that communication between different cell types plays an important role in the immune response to infection and that the interaction of specific ligand-receptor pairs may regulate immune cell function. Finally, we identified hub genes regulated during T cell differentiation, including Il2rb, Ctla4, Ccl5, Foxp3, Cd28, and Cd27, whose expression correlates with the immune response after infection. Despite the limitations of the small sample size of the single-cell design, our findings are a valuable resource for understanding the immune response to C. albicans infection and hold promise for developing novel immunotherapeutic strategies against C. albicans infection.
Acknowledgement: None.
Funding Statement: This work was supported by National Key Research and Development Program of China (2021YFC2301405), Chongqing Talent Program (No. CQYC202003220).
Author Contributions: Study conception and design: Quanming Zou and Hao Zeng; data collection: Keran Jia, Huihai Zhao, Yanhao Zhang, Mengyu Jiang and Mengge Cui; analysis and interpretation of results: Keran Jia, Jia Wang, Jiajia Zhang, Huihai Zhao and Mengyan Li; draft manuscript preparation: Keran Jia, Mengyu Jiang, Quanming Zou and Hao Zeng; reviewed and edited the manuscript: Quanming Zou and Hao Zeng. All authors reviewed the results and approved the final version of the manuscript.
Availability of Data and Materials: The data that support the findings of this study are available from China National Center for Bioinformation (CNCB) but restrictions apply to the availability of these data, which were used under license for the current study, and so are not publicly available. Data are however available from the authors upon reasonable request and with permission of CNCB.
Ethics Approval: The study protocol was approved by the Ethics Committee of the 980th Hospital of the Joint Logistics Support Force of the Chinese People’s Liberation Army (2022-KY-129) and followed the principles of the Declaration of Helsinki.
Conflicts of Interest: The authors declare that they have no conflicts of interest.
References
1. Kawamoto H, Masuda K, Nagano S. Regeneration of antigen-specific T cells by using induced pluripotent stem cell (iPSC) technology. Int Immunol. 2021;33(12):827–33. doi:10.1093/intimm/dxab091. [Google Scholar] [PubMed] [CrossRef]
2. Christophersen A, Dahal-Koirala S, Chlubnová M, Jahnsen J, Lundin KEA, Sollid LM. Phenotype-based isolation of antigen-specific CD4+ T cells in autoimmunity: a study of celiac disease. Adv Sci. 2022;9(10):e2104766. doi:10.1002/advs.202104766. [Google Scholar] [PubMed] [CrossRef]
3. Laphanuwat P, Gomes DCO, Akbar AN. Senescent T cells: beneficial and detrimental roles. Immunol Rev. 2023;316(1):160–75. doi:10.1111/imr.13206. [Google Scholar] [PubMed] [CrossRef]
4. Kojima H, Kashiwakura Y, Kanno Y, Hashiguchi M, Kobata T. Fine-tuning of antigen-specific immune responses by regulatory T cells activated via antigen recognition-independent and humoral factor-dependent mechanisms. Scand J Immunol. 2021;93(6):e13020. doi:10.1111/sji.13020. [Google Scholar] [PubMed] [CrossRef]
5. Barnaba V. T cell memory in infection, cancer, and autoimmunity. Front Immunol. 2021;12:811968. doi:10.3389/fimmu.2021.811968. [Google Scholar] [PubMed] [CrossRef]
6. Wang X, Xu T, Wu S, Li L, Cai X, Chen F, et al. Candida albicans-myeloid cells-T lymphocytes axis in the tumor microenvironment of oral tumor-bearing mice. Cancer Lett. 2024;588(1):216814. doi:10.1016/j.canlet.2024.216814. [Google Scholar] [PubMed] [CrossRef]
7. Gow NA, van de Veerdonk FL, Brown AJ, Netea MG. Candida albicans morphogenesis and host defence: discriminating invasion from colonization. Nat Rev Microbiol. 2011;10(2):112–22. doi:10.1038/nrmicro2711. [Google Scholar] [PubMed] [CrossRef]
8. Li D, Cheng J, Calderone R, Bellanti JA. Measurements of treg cell induction by Candida albicans DNA Using flow cytometry. Methods Mol Biol. 2022;2542:301–6. doi:10.1007/978-1-0716-2549-1. [Google Scholar] [CrossRef]
9. Langenhorst D, Fürst AL, Alberter K, Vilhena C, Dasari P, Daud M, et al. Soluble enolase 1 of Candida albicans and Aspergillus fumigatus stimulates human and mouse B cells and monocytes. J Immunol. 2023;211(5):804–15. doi:10.4049/jimmunol.2200318. [Google Scholar] [PubMed] [CrossRef]
10. Richardson JP, Moyes DL. Adaptive immune responses to Candida albicans infection. Virulence. 2015;6(4):327–37. doi:10.1080/21505594.2015.1004977. [Google Scholar] [PubMed] [CrossRef]
11. Qin Y, Zhang L, Xu Z, Zhang J, Jiang YY, Cao Y, et al. Innate immune cell response upon Candida albicans infection. Virulence. 2016;7(5):512–26. doi:10.1080/21505594.2016.1138201. [Google Scholar] [PubMed] [CrossRef]
12. Laidlaw BJ, Craft JE, Kaech SM. The multifaceted role of CD4+ T cells in CD8+ T cell memory. Nat Rev Immunol. 2016;16(2):102–11. doi:10.1038/nri.2015.10. [Google Scholar] [PubMed] [CrossRef]
13. Xu L, Liu Y, Song X, Li Y, Han Y, Zhu T, et al. Naïve CD4+ cell counts significantly decay and high HIV RNA levels contribute to immunological progression in long-term non-progressors infected with HIV by blood products: a cohort study. BMC Immunol. 2021;22(1):36. doi:10.1186/s12865-021-00426-8. [Google Scholar] [PubMed] [CrossRef]
14. Deng W, Su Z, Liang P, Ma Y, Liu Y, Zhang K, et al. Single-cell immune checkpoint landscape of PBMCs stimulated with Candida albicans. Emerg Microbes Infect. 2021;10(1):1272–83. doi:10.1080/22221751.2021.1942228. [Google Scholar] [PubMed] [CrossRef]
15. Zhang K, Hu Y, Li R, Li T. Single-cell atlas of murine adrenal glands reveals immune-adrenal crosstalk during systemic Candida albicans infection. Front Immunol. 2022;13:966814. doi:10.3389/fimmu.2022.966814. [Google Scholar] [PubMed] [CrossRef]
16. Yao Q, He Y, Deng L, Chen D, Zhang Y, Luo H, et al. Rapid detection of pathogenic fungi from coastal population with respiratory infections using microfluidic chip technology. BMC Infect Dis. 2024;24(1):326. doi:10.1186/s12879-024-09212-4. [Google Scholar] [PubMed] [CrossRef]
17. Hameed MRP, Makris M, Weger-Lucarelli J. Optimized protocol for mouse footpad immune cell isolation for single-cell RNA sequencing and flow cytometry. STAR Protoc. 2023;4(3):102409. doi:10.1016/j.xpro.2023.102409. [Google Scholar] [PubMed] [CrossRef]
18. O’Leary K, Zheng D. Metacell-based differential expression analysis identifies cell type specific temporal gene response programs in COVID-19 patient PBMCs. NPJ Syst Biol Appl. 2024;10(1):36. doi:10.1038/s41540-024-00364-2. [Google Scholar] [PubMed] [CrossRef]
19. Mangiola S, Doyle MA, Papenfuss AT, Mathelier A. Interfacing seurat with the R tidy universe. Bioinformatics. 2021;37(22):4100–7. doi:10.1093/bioinformatics/btab404. [Google Scholar] [PubMed] [CrossRef]
20. Qiu X, Mao Q, Tang Y, Wang L, Chawla R, Pliner HA, et al. Reversed graph embedding resolves complex single-cell trajectories. Nat Methods. 2017;14(10):979–82. doi:10.1038/nmeth.4402. [Google Scholar] [PubMed] [CrossRef]
21. Kuleshov MV, Jones MR, Rouillard AD, Fernandez NF, Duan Q, Wang Z. Enrichr: a comprehensive gene set enrichment analysis web server 2016 update. Nucleic Acids Res. 2016;44(W1):W90–7. doi:10.1093/nar/gkw377. [Google Scholar] [PubMed] [CrossRef]
22. Xie K, Li C, Wang M, Fu S, Cai Y. miR-135a-5p overexpression in peripheral blood-derived exosomes mediates vascular injury in type 2 diabetes patients. Front Endocrinol. 2023;14:1035029. doi:10.3389/fendo.2023.1035029. [Google Scholar] [PubMed] [CrossRef]
23. Su W, Hu S, Zhou L, Bi H, Li Z. FOXP2 inhibits the aggressiveness of lung cancer cells by blocking TGFβ signaling. Oncol Lett. 2024;27(5). doi:10.3892/ol.2024.14361. [Google Scholar] [PubMed] [CrossRef]
24. Zhao L, Huang J, Liu W, Su X, Zhao B, Wang X, et al. Long non-coding RNA RAD51-AS1 promotes the tumorigenesis of ovarian cancer by elevating EIF5A2 expression. J Cancer Res Clin Oncol. 2024;150(4). doi:10.1007/s00432-024-05671-z. [Google Scholar] [PubMed] [CrossRef]
25. Shirafkan F, Hensel L, Rattay K. Immune tolerance and the prevention of autoimmune diseases essentially depend on thymic tissue homeostasis. Front Immunol. 2024;15:1339714. doi:10.3389/fimmu.2024.1339714. [Google Scholar] [PubMed] [CrossRef]
26. Büttner JK, Becker S, Fink A, Brinkmann MM, Holtappels R, Reddehase MJ, et al. Direct antigen presentation is the canonical pathway of cytomegalovirus CD8 T-cell priming regulated by balanced immune evasion ensuring a strong antiviral response. Front Immunol. 2023;14:1272166. doi:10.3389/fimmu.2023.1272166. [Google Scholar] [PubMed] [CrossRef]
27. Shu C, Sun L, Zhang W. Thymol has antifungal activity against Candida albicans during infection and maintains the innate immune response required for function of the p38 MAPK signaling pathway in Caenorhabditis elegans. Immunol Res. 2016;64(4):1013–24. doi:10.1007/s12026-016-8785-y. [Google Scholar] [PubMed] [CrossRef]
28. Nikou S-A, Zhou C, Griffiths JS, Kotowicz NK, Coleman BM, Green MJ, et al. The Candida albicans toxin candidalysin mediates distinct epithelial inflammatory responses through p38 and EGFR-ERK pathways. Sci Signal. 2022;15(728):eabj6915. doi:10.1126/scisignal.abj6915. [Google Scholar] [PubMed] [CrossRef]
29. Hernández-Santos N, Huppler AR, Peterson AC, Khader SA, McKenna KC, Gaffen SL. Th17 cells confer long-term adaptive immunity to oral mucosal Candida albicans infections. Mucosal Immunol. 2013;6(5):900–10. doi:10.1038/mi.2012.128. [Google Scholar] [PubMed] [CrossRef]
30. Pandiyan P, Conti HR, Zheng L, Peterson AC, Mathern DR, Hernández-Santos N, et al. CD4+CD25+Foxp3+ regulatory T cells promote Th17 cells in vitro and enhance host resistance in mouse Candida albicans Th17 cell infection model. Immunity. 2011;34(3):422–34. doi:10.1016/j.immuni.2011.03.002. [Google Scholar] [PubMed] [CrossRef]
31. Bacher P, Steinbach A, Kniemeyer O, Hamprecht A, Assenmacher M, Vehreschild MJ, et al. Fungus-specific CD4+ T cells for rapid identification of invasive pulmonary mold infection. Am J Respir Crit Care Med. 2015;191(3):348–52. doi:10.1164/rccm.201407-1235LE. [Google Scholar] [PubMed] [CrossRef]
32. Tavukcuoglu E, Horzum U, Cagkan Inkaya A, Unal S, Esendagli G. Functional responsiveness of memory T cells from COVID-19 patients. Cell Immunol. 2021;365:104363. doi:10.1016/j.cellimm.2021.104363. [Google Scholar] [PubMed] [CrossRef]
33. Rivera A, Ro G, Van Epps HL, Simpson T, Leiner I, Sant’Angelo DB, et al. Innate immune activation and CD4+ T cell priming during respiratory fungal infection. Immunity. 2006;25(4):665–75. doi:10.1016/j.immuni.2006.08.016. [Google Scholar] [PubMed] [CrossRef]
34. Pathakumari B, Liang G, Liu W. Immune defence to invasive fungal infections: a comprehensive review. Biomed Pharmacother. 2020;130(165):110550. doi:10.1016/j.biopha.2020.110550. [Google Scholar] [PubMed] [CrossRef]
35. Huffnagle GB. Role of cytokines in T cell immunity to a pulmonary Cryptococcus neoformans infection. Biol Signals. 1996;5(4):215–22. doi:10.1159/000109193. [Google Scholar] [PubMed] [CrossRef]
36. Belkaid Y, Rouse BT. Natural regulatory T cells in infectious disease. Nat Immunol. 2005;6(4):353–60. doi:10.1038/ni1181. [Google Scholar] [PubMed] [CrossRef]
37. Mills KH. Regulatory T cells: friend or foe in immunity to infection? Nat Rev Immunol. 2004;4(11):841–55. 2004/11/02. doi:10.1038/nri1485. [Google Scholar] [PubMed] [CrossRef]
38. Li DD, Jawale CV, Zhou C, Lin L, Trevejo-Nunez GJ, Rahman SA, et al. Fungal sensing enhances neutrophil metabolic fitness by regulating antifungal Glut1 activity. Cell Host Microbe. 2022;30(4):530–44.e536. doi:10.1016/j.chom.2022.02.017. [Google Scholar] [PubMed] [CrossRef]
39. Gazendam RP, van de Geer A, Roos D, van den Berg TK, Kuijpers TW. How neutrophils kill fungi. Immunol Rev. 2016;273(1):299–311. doi:10.1111/imr.12454. [Google Scholar] [PubMed] [CrossRef]
40. Seeling M, Pöhnl M, Kara S, Horstmann N, Riemer C, Wöhner M, et al. Immunoglobulin G-dependent inhibition of inflammatory bone remodeling requires pattern recognition receptor Dectin-1. Immunity. 2023;56(5):1046–63.e7. doi:10.1016/j.immuni.2023.02.019. [Google Scholar] [PubMed] [CrossRef]
41. Kalia N, Singh J, Kaur M. The role of dectin-1 in health and disease. Immunobiology. 2021;226(2):152071. doi:10.1016/j.imbio.2021.152071. [Google Scholar] [PubMed] [CrossRef]
42. Nauseef WM, Borregaard N. Neutrophils at work. Nat Immunol. 2014;15(7):602–11. doi:10.1038/ni.2921. [Google Scholar] [PubMed] [CrossRef]
43. Ozturk A, Kara MM. Diagnostic and prognostic significance of the lymphocyte/C-reactive protein ratio, neutrophil/lymphocyte ratio, and D-dimer values in patients with COVID-19. BIOCELL. 2022;46(12):2625–35. doi:10.32604/biocell.2022.023124. [Google Scholar] [CrossRef]
44. Zheng C, Cao T, Ye C, Zou Y. Neutrophil recruitment by CD4 tissue-resident memory T cells induces chronic recurrent inflammation in atopic dermatitis. Clin Immunol. 2023;256:109805. doi:10.1016/j.clim.2023.109805. [Google Scholar] [PubMed] [CrossRef]
45. Dieu MC, Vanbervliet B, Vicari A, Bridon JM, Oldham E, Aït-Yahia S, et al. Selective recruitment of immature and mature dendritic cells by distinct chemokines expressed in different anatomic sites. J Exp Med. 1998;188(2):373–86. doi:10.1084/jem.188.2.373. [Google Scholar] [PubMed] [CrossRef]
46. Ying H, Yang L, Qiao G, Li Z, Zhang L, Yin F, et al. Cutting edge: CTLA-4--B7 interaction suppresses Th17 cell differentiation. J Immunol. 2010;185(3):1375–8. doi:10.4049/jimmunol.0903369. [Google Scholar] [PubMed] [CrossRef]
47. Liang P, Wu Y, Qu S, Younis M, Wang W, Wu Z, et al. Exploring the biomarkers and potential therapeutic drugs for sepsis via integrated bioinformatic analysis. BMC Infect Dis. 2024;24(1):32. doi:10.1186/s12879-023-08883-9. [Google Scholar] [PubMed] [CrossRef]
48. Ji G, Yang Q, Wang S, Yan X, Ou Q, Gong L, et al. Single-cell profiling of response to neoadjuvant chemo-immunotherapy in surgically resectable esophageal squamous cell carcinoma. Genome Med. 2024;16(1):49. doi:10.1186/s13073-024-01320-9. [Google Scholar] [PubMed] [CrossRef]
49. Galvez-Cancino F, Simpson AP, Costoya C, Matos I, Qian D, Peggs KS, et al. Fcγ receptors and immunomodulatory antibodies in cancer. Nat Rev Cancer. 2024;24(1):51–71. doi:10.1038/s41568-023-00637-8. [Google Scholar] [PubMed] [CrossRef]
Cite This Article
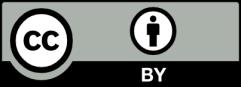
This work is licensed under a Creative Commons Attribution 4.0 International License , which permits unrestricted use, distribution, and reproduction in any medium, provided the original work is properly cited.