Open Access
REVIEW
Computational and bioinformatics tools for understanding disease mechanisms
1 Physics Department, University of Cagliari, Cittadella Universitaria, Monserrato (CA), 09042, Italy
2 Department of Chemistry, School of Energy Technology, Pandit Deendayal Energy University, Gandhinagar, 382426, India
3 Department of Chemistry, College of Science, King Khalid University, Abha, 61413, Saudi Arabia
* Corresponding Author: MOHD ATHAR. Email:
(This article belongs to the Special Issue: Computational Approaches in Molecular and Cellular Biology: Advancements in Disease Research and Therapeutic Design)
BIOCELL 2024, 48(6), 935-944. https://doi.org/10.32604/biocell.2024.049891
Received 22 January 2024; Accepted 03 April 2024; Issue published 10 June 2024
Abstract
Computational methods have significantly transformed biomedical research, offering a comprehensive exploration of disease mechanisms and molecular protein functions. This article reviews a spectrum of computational tools and network analysis databases that play a crucial role in identifying potential interactions and signaling networks contributing to the onset of disease states. The utilization of protein/gene interaction and genetic variation databases, coupled with pathway analysis can facilitate the identification of potential drug targets. By bridging the gap between molecular-level information and disease understanding, this review contributes insights into the impactful utilization of computational methods, paving the way for targeted interventions and therapeutic advancements in biomedical research.Keywords
The field of bioinformatics has experienced significant expansion within systems biology, specifically through the evolution of network databases and tools. These advancements provide essential means for effectively analyzing complex biological processes, genes, and protein networks [1]. Numerous diseases spanning diverse medical domains, such as oncology, cancer immunotherapy, infectious diseases, neurological disorders, heart failure, inflammation, and oxidative stress, have been linked to disruptions in protein-protein interactions (PPIs). In the cellular systems of all living organisms, PPIs and multi-protein complexes play pivotal roles, and deviations from their normal patterns can lead to disease states.
Biomolecular networks, comprising gene and transcription regulatory networks, protein-protein interaction networks, metabolic networks, signaling networks, and hybrid networks, serve as potent resources for uncovering disease-driving genes and genetic modules. The advent of high-throughput measurement techniques like microarray, RNA-seq, chromatin immunoprecipitation with DNA microarray (ChIP-on-chip), and mass spectrometry has led to the generation of extensive biological datasets. These datasets, enriched with detailed information, prove invaluable for comprehending the mechanisms of molecular biological systems, contributing to the diagnosis, treatment, and drug design for complex diseases [2,3].
Despite the existence of numerous tools and databases, there is a lack of systematic comparison and organization of information for understanding disease mechanisms and target identification. This review is dedicated to computational tools and databases that significantly contribute to our understanding of diseases, encompassing protein-protein, protein-gene, and gene-gene interactions, genetic variations, and their annotations in metabolic pathways. By exploring various facets of bioinformatics analysis, including network analysis, gene-gene associations, and disease pathway enrichment analyses, our goal is to offer a comprehensive overview of the computational landscape in biomedical research. Subsequent sections will delve into disease-single nucleotide polymorphism (SNP)/gene associations and genetic variation analyses, dissecting disease mechanisms through protein-protein interaction studies, structural modeling, and dynamics. This comprehensive approach positions our review as a valuable resource for researchers navigating the intricate realm of computational tools and databases in biomedical research.
Bioinformatics Analysis and Functional Insights
Network analysis, integration, protein-protein and gene-gene associations
Protein-protein interaction networks are crucial for understanding disease mechanisms and identifying potential therapeutic targets. Analyzing these interactions provides insights into how a protein interacts within cellular pathways, unveiling key players and vulnerabilities that can be targeted for intervention in diseases. Predicting the protein function of a target protein and its drugability is crucial, especially since it has to be mediated by protein-protein interactions. Using computational tools (a summary given in Table 1), the analysis of interaction networks is increasingly popular, also due to challenges in scaling up interaction experiments [5]. Utilizing data from global mRNA expression profiling studies and curated interaction databases, tools like Cytoscape provide a systematic and accessible approach for network visualization and integration, with numerous plugins available for additional data analysis.
Some of the common databases containing information about protein-protein interaction (PPI) are reported in Fig. 1. The majority of genes and proteins realize resulting phenotype functions as a set of interactions, as has been reviewed by Liu and Chen, for the available PPI databases and methods for predicting PPI networks [6]. Among the PPI database, BioGRID, MIPS, and STRING are tools for analyzing protein functions (Fig. 1) [7–9]. BioGRID, often dubbed the golden sea of PPI drug targets, stands out as a comprehensive repository, excelling in both the number of proteins and exclusive interactions [7]. Boasting a vast database housing 67.6 million proteins and over 20 billion interactions, BioGRID is a vital resource for exhaustive searches in both experimental and predicted PPIs [7]. Positioned at the forefront of network analysis and gene-gene associations, BioGRID distinguishes itself as a pivotal resource with, the highest coverage, curating and integrating data from diverse sources to advance systems and molecular biology [10]. Despite limitations in listing multi-protein complexes larger than dimers, BioGRID’s recent update includes over 1 million non-redundant interactions and stands as a primary source for experimentally derived PPI data and complex cell signaling networks [10]. Completing this ensemble, STRING (Search Tool for the Retrieval of Interacting Genes/Proteins) spans both direct and indirect protein-protein interactions. Integrating experimental and predicted interaction data, STRING offers a panoramic view of the interactome. Researchers across the globe rely on STRING for network analysis, using its insights to explore functional associations between genes and proteins across diverse organisms [11].
Figure 1: Broad classification of some of the common databases containing information about PPI (reprinted with copyright permission) [4].
Another database, BIND, although its curation ended in 2005, remains a highly cited and valuable resource [12]. This peer-reviewed database contains diversely curated experimental data, including high-throughput datasets and protein complexes from PDB. BIND continues to contribute significantly to the understanding of complex cell signaling networks [13].
UniProt stands as a comprehensive database, providing a vast collection of protein sequences and annotations. Serving as a central hub, it guides researchers through the complexities of gene-gene associations and protein interactions, synthesizing data from diverse sources. With annotated information and links to databases such as InterPro, European Molecular Biology Laboratory (EMBL), Protein ANalysis THrough Evolutionary Relationships (PANTHER), Protein FAMilies (Pfam), Gene3D, Superfamily (SUPFAM), Reference Sequence (RefSeq), AlphaFoldDB, and SWISS-MODEL Repository (SMR), UniProt offers a wealth of knowledge on biological entities. Mentha and IntAct serve as vital repositories for experimentally verified interaction datasets [14]. Mentha facilitates network analysis for 8 interactomes, unraveling molecular pathways and cellular processes, and assigns to each interaction a reliability score that takes into account all the supporting evidence [14]. This database updates the interactions every week so one of the most up-to-date. IntAct, developed at EMBL-EBI, extends beyond binary interactions to include complex molecular associations, enhancing network analysis and functional genomics [15]. As an open database, IntAct sources interactions from literature and direct data depositions by expert curators, utilizing a deep annotation model for detailed information dissemination.
The primary and secondary protein interaction databases along with the interaction coverage of these datasets are illustrated in Fig. 2. Database of Interacting Proteins (DIP) is a comprehensive catalog of experimentally determined protein-protein interactions, that explore protein interaction networks [16]. This database includes intricate associations underlying cellular processes and gene-gene relationships, combining information from various sources to create a unified set of protein-protein interactions.
Figure 2: (a) Schematic representation of data flow (direction is shown by arrow) among primary (red color) and secondary (blue node) protein interaction databases. The arrows indicate the direction of data flow. (b) Protein interaction coverage across databases (reprint with copyright permission) [20].
MINT (Molecular INTeraction Database) takes center stage in the molecular ballet, offering a curated dataset focused on experimentally verified molecular interactions, with a primary emphasis on protein-protein interactions (Table 1). MINT facilitates network analysis and functional genomics, providing researchers with insights into the intricate molecular mechanisms within cells [17]. The database, with more than 12,5464 interactions and 25,530 proteins, primarily focuses on model organisms and assigns confidence scores to experimentally detected protein-protein interactions, reflecting their reliability on a scale from 0 to 1 [17,18]. MINT’s unique approach involves extracting interaction data and experimental details from peer-reviewed literature using a literature mining program, the MINT assistant, and subsequent validation by expert curators [17]. This dual curation enhances the reliability and comprehensiveness of protein-protein interaction information within the DIP database. Additionally, HPRD (Human Protein Reference Database) specializes in providing detailed insights into human proteins and their interactions, enriching the tapestry of gene-gene associations within the human biological context [19]. Even though BioGRID shows the highest coverage (69.5%) of PPIs, HPRD retained the top position in terms of usage frequency [20]. The database covers interaction networks from various organisms, including Homo sapiens, C. elegans, bacteria, and 73 different viruses. As an active partner of the International Molecular Exchange Consortium (IMEx), MINT aligns with IMEx standards and supports the Protein Standard Initiative (PSI) recommendation [17]. Notably, since September 2013, MINT has integrated the IntACT database infrastructure to streamline efforts and enhance software development [17].
Overall, public databases like BioGRID, DIP, MINT, and STRING provide predictive and experimental interaction information. Network representations, crucial for visualizing complex biological activities, describe interactions between entities of interest. Schwikowski et al. used PPI networks to predict novel protein functions in yeast, revealing a single large network of 2,358 interactions out of 2,709 total interactions [21]. Global patterns in large-scale systems can be effectively shown using nodes and edges to represent entities and interactions, respectively.
HumanNet uses a holistic approach by integrating diverse genomic data to infer functional relationships between genes and it covers 99.8% of human protein-coding genes. HumanNet can predict host genes associated with diseases. By leveraging information from multiple sources, including protein-protein interactions, HumanNet aids in constructing a comprehensive functional gene network [22].
In the symphony of databases, FunCoup emerges as a key player, exploring functional coupling between proteins [23]. Integrating various types of functional genomics data, FunCoup becomes a guide for researchers deciphering the intricate web of functional relationships and coordination between genes in biological processes.
Disease pathway enrichment analysis
Pathway enrichment analysis helps reveal the intricate connections between different biological pathways involved in diseases, providing insights into their molecular complexities. Databases such as KEGG (Kyoto Encyclopedia of Genes and Genomes) [24], Reactome [25], and WikiPathways offer comprehensive repositories of curated pathways, serving as foundational resources for pathway enrichment analyses. By employing statistical methods, these analyses identify overrepresented pathways in a set of genes associated with a particular disease, providing a systems-level understanding of the underlying molecular mechanisms. Tools like IPA (Ingenuity Pathway Analysis) can further enhance this exploration, offering interactive visualization and interpretation of complex biological pathways, thus empowering researchers to dissect disease-related networks and formulate targeted interventions based on a holistic understanding of the molecular landscape.
Reactome is a database of pathways and reactions (pathway steps) in human biology that have been curated by expert biologist researchers and is extensively cross-referenced to other resources, e.g., National Center for Biotechnology Information (NCBI), Ensembl, UniProt, University of California, Santa Cruz (UCSC) Genome Browser, HapMap, KEGG (Gene and Compound), ChEBI, PubMed and GO [25,26]. It includes many events in biology that involve changes in state, such as binding, activation, translocation, and degradation, in addition to classical biochemical pathways.
Disease-SNP/gene association and genetic variation analysis
Information on genetic variations associated with diseases can enable researchers to understand the underlying genetic factors, discover potential biomarkers, and uncover novel pathways for targeted interventions in various medical conditions. Such genetic variations help in dissecting the interplay between diseases and genetic elements. Notable platforms include miRdSNP, which focuses on microRNA-SNP interactions [27]; dbSNP, a comprehensive repository for cataloging SNPs across the human genome; Wellcome Trust Case Control Consortium (WTCCC) and Genome-Wide Association Studies (GWAS) catalog, valuable resources derived from genome-wide association studies [28]; ClinVar, offering insights into the clinical significance of genetic variations [29]; and DisGeNET, an aggregator of gene-disease associations [30]. Furthermore, Online Mendelian Inheritance in Man (OMIM) serves as a definitive resource for inherited diseases, while SIDD and CTD provide unique perspectives on SNPs in diseases [14,16]. Phar-mGKB explores the genetic basis of drug response [31], Genetic Association Database (GAD) compiles information on genetic associations with various diseases and traits [32], and disease-specific databases such as Malacards, Catalogue of Somatic Mutations In Cancer (COSMIC), Human Gene Mutation Database (HGMD), and Psychiatric Genomics Consortium Database (PsyGENET) delve into various medical domains [33–36]. Collectively, these databases, including DISEASE [37,38], contribute to a comprehensive understanding of disease-SNP/gene associations and genetic variation analysis, offering insights that span from the molecular to the clinical level.
In the pursuit of unraveling the complexities of the genetic basis of diseases, these databases collectively empower researchers to navigate the expansive landscape of disease-SNP/gene associations and genetic variation analysis. Each database provides a unique lens, from the intricacies of microRNA-SNP interactions [39] to cataloging SNPs across the human genome, insights from genome-wide association studies [40], and clinical significance of genetic variations [29]. Additionally, these resources aggregate gene-disease associations [41], offer detailed information on inherited diseases and explore the impact of single nucleotide changes in diseases [14,16]. Specialized databases focus on drug-gene interactions [31], genetic associations with diseases [42], and specific medical domains [33–36]. Together with DISEASE [38], these databases form an invaluable repository, contributing to our understanding of genetic variations and their implications in diseases across diverse medical contexts.
Functional annotation and gene set analysis
Gene annotation facilitates the understanding of biological functions, processes, and cellular components associated with diseases, enabling a comprehensive exploration of gene lists and uncovering the functional significance of gene sets in disease-related pathways. Gene Ontology (GO) acts as a foundational resource, providing a structured vocabulary and hierarchical framework to systematically annotate and analyze the functional landscape of genes [43]. DAVID (Database for Annotation, Visualization, and Integrated Discovery) seamlessly integrates functional genomics data with annotation tools, offering a versatile suite to interpret large-scale genomic datasets [44]. Enrichr enhances functional annotation with a user-friendly interface, enabling comprehensive exploration of gene sets and dynamic visualization of enrichment results [45]. g: Profiler, a powerful tool for functional enrichment analysis, employs a diverse set of statistical methods and integrates various organism databases, providing researchers with a versatile resource for uncovering functional signatures associated with gene lists [46]. Together, Gene Ontology, DAVID, Enrichr, and g: Profiler form a formidable quartet, combining fundamental vocabulary with dynamic and user-friendly platforms to guide researchers in decoding the complex functional dimensions of genes and pathways.
Gene Expression Omnibus (GEO) is a public database hosted by the NCBI provides a platform for the storage and retrieval of high-throughput gene expression and molecular abundance data [48]. Researchers worldwide can access and analyze a diverse range of genomic datasets, contributing to the advancement of genomics and biomedical research. Another tool, Search Tool for Interactions of Chemicals (STITCH) is a computational tool designed to explore interactions between chemicals, including small molecules and drugs [49,50].
STITCH integrates extensive data on protein interactions, pathways, and text-mining information, offering insights into the intricate network of chemical interactions, comprising a remarkable 1.6 billion interactions. Research by O’Reilly et al. exemplifies STITCH’s utility in identifying potential drug targets for α1-antitrypsin deficiency [51]. STITCH can also be queried for a set of chemicals to reveal possible targets, as demonstrated in the study by Kumar et al. which screened compounds capable of altering intracellular manganese levels [52].
Among the methods reviewed to determine protein-protein and gene-gene associations, several limitations exist. In databases such as BIOGRID and MENTHA, although the data is derived from experimental evidence, biases may be present towards well-studied genes and interactions. These databases might not capture the entirety of possible interactions and can be limited in their representation of certain proteins or conditions, potentially overlooking the full complexity of dynamic protein-protein interactions. In the case of IntAct, DIP, and HPRD, the coverage might exhibit bias towards specific model organisms or well-studied proteins, leading to a potential lack of representation for diverse interactions occurring in different cellular contexts. Additionally, these databases may not be fully up-to-date and could lack information on less-studied proteins. MINT may suffer from context-dependent interactions that are not fully represented. In the case of HumanNet, FunCoup, and STRING, the accuracy of predicted interactions depends on the underlying algorithms. Predicted interactions may not always align with actual biological interactions, introducing the possibility of false positives.
In the context of Genetic Variation Analysis methods, such as miRdSNP, there may be limited coverage for specific microRNAs and SNPs, potentially resulting in the omission of certain associations. Considering the diverse functional effects of SNPs, the dbSNP database may lack detailed annotations for some variants, and it might not comprehensively capture rare or novel variants. The significance of databases like WTCCC and GWAS, emphasizing large-scale genome-wide association studies, may inadvertently overlook rare variants within certain populations, impacting the replicability of findings across diverse populations. In the case of ClinVar, detailed clinical annotations for some variants may be lacking, and the database might not encompass the full spectrum of genetic variation. PheGenI may face limitations due to the restricted availability of phenotypic data for certain traits, potentially impacting the analyses. The COSMIC database, with its exclusive focus on somatic mutations in cancer, may not provide comprehensive coverage of germline mutations and is particularly tailored to cancer-related research. Regarding HGMD, the database predominantly covers germline mutations associated with inherited diseases, potentially overlooking somatic mutations. Additionally, accessibility to HGMD may be restricted, requiring a subscription for full access.
Enrichment case studies of human disease
Predicting disease-related genes involves leveraging various features and patterns. The rapid identification of the genome-wide human PPIs network provided us with new avenues for elucidating the disease gene directly from the PPIs network [53].
Zheng et al. utilized computational approaches, specifically conducting functional enrichment analyses, to unveil 1,170 differentially expressed genes (DEGs) in glioblastoma multiforme (GBM) samples and identify the top 20 hub genes (Fig. 3) [47]. A similar analysis of enrichment and protein-pathway correlation was performed by Armendáriz-Castillo et al., which identified potential molecular markers for Alternative Lengthening of Telomeres (ALT) in 411 telomere maintenance gene sets across 31 Pan-Cancer Atlas studies. They employed the STRING database to construct a protein-protein interaction network and utilized GO, KEGG, and REACTOME for enrichment analysis. In doing so, they identified primary pathways and their involvement in ALT-related processes, such as homologous recombination and homology-directed repair. Researchers have explored sequence features [54] and expression patterns [55] to identify disease-causing genes or gene prioritization have recently been reviewed by Kaushal et al. [56] and the computational techniques have been classified into filtering-based techniques (work based on the properties of genes), similarity-based techniques (perform prioritization by calculating the similarity between candidate genes and seed genes), and network-based techniques (uses the topology of the network for ranking the genes) [57].
Figure 3: Functional enrichment analyses of differentially expressed genes (DEGs) and hub genes in expression data of glioma samples from Henry Ford Hospital (GSE4290). The volcano plot in (a) illustrates up-regulated (red dots) and down-regulated (blue dots) DEGs. GO function analysis (b) revealed statistically enriched biological processes, molecular functions, and cellular components among the DEGs. KEGG pathway analysis (c) identified the top 30 enriched pathways associated with DEGs. Additionally, (d) showcases the top 20 hub genes predicted from the DEGs using the Cytoscape and STRING, crucial in the occurrence and progression of human glioblastoma multiforme [47].
Topological features are more important and popular in PPI networks owing to the basis that disease-associated genes exhibit non-random positioning in the network. They often display high connectivity, clustering, and central network locations [58]. Researchers have discovered additional topological features; for instance, Tu et al. [59] found that the degrees of disease genes in PPI networks are significantly higher than other genes. Oti et al. [60] observed that genes neighboring disease-related genes are more likely to be disease-related. Xu et al. [53] developed a classifier employing five quantities to measure different topological features. Despite their success, local topology-based methods face limitations when predicting disease-related genes within a single disease-gene family, especially for genes not in proximity to known disease genes. To address this, a new method has been proposed based on topological similarity [61] that considers the entire graph, allowing two vertices to be similar without sharing neighbors [38].
Protein-protein interaction and the genes susceptible in rare diseases have been found important and a database integration called ODCs (Orphan Disease Connections) establishes shared susceptibility genes and protein interactions of the corresponding gene products. the diseases connected to one of interest, to explore in detail the connections between two rare diseases, or to search for rare diseases associated with a given gene [38].
In the exploration of Disease Biomarker Discovery, tools for discerning interactions among disease-related proteins emerge as potential biomarkers. Investigating these interactions holds the promise of uncovering diagnostic markers for diseases. A gene interaction network was built using 98 shared Differentially Expressed Genes (DEGs) in dysplastic and cancer cells. The analysis identified common modules, hubs, and significant motifs. Notably, ZWINT, CDC7, MCM4, MCM2, and MCM6 were identified as influential genes in neoplasia, playing a crucial role in disease progression [62]. Metabolic signatures were employed to investigate the pathogenesis of Hypersensitivity Pneumonitis (HP), allowing the identification of enriched biological processes, altered pathways, and the protein-protein interaction (PPI) network associated with differentially expressed genes. The findings reveal impaired glycolysis and phosphatidylinositol-3-kinase (PI3K/AKT) pathways in patients with HP [63].
Conclusion and Future Perspective
The comprehensive review underscores the importance of integrating various databases, providing a holistic understanding of protein interactions, disease pathways, and genetic variations. Access to genetic variation tools and databases offers insights into genetic contributions to pathologies. The identification of hub genes, enriched pathways, and potential molecular markers sets the stage for targeted interventions in complex diseases.
Themed projects dedicated to interaction curation have recently emerged, focusing on pivotal biological processes relevant to diseases [64]. BIOGRID’s project-based curation approach for human protein interactions enables the creation of focused, impactful datasets. These projects also explore specific diseases, such as glioblastoma (51,613 interactions), Fanconi Anemia (32,016 interactions), COVID-19 Coronavirus (42,526 interactions), the Ubiquitin-Proteasome System (409,395 interactions), Autophagy (53,334 interactions), and S. cerevisiae Kinome (106,059 interactions) [65] (updated on 18th Feb 2024). New themed curation projects underway include Alzheimer’s disease [66] as well as new viral, bacterial, and protozoan pathogens, all of which will be supported by dedicated themed project pages.
Recent advances in artificial intelligence approaches, particularly in secondary structure prediction [67], as demonstrated by tools like AlphaFold [68], NeuralPLexer [69], or RoseTTAfold [70] have unraveled extensive information related to the primary structure. This includes mutations and interfacial protein-protein interactions (or multimers), not only in exploring metabolic pathways but also in rare diseases such as motor neuron diseases [71,72]. Despite this progress, challenges like predicting post-translational modifications and navigating the complexities of DNA, RNA, and their complexes still exist.
While combining various methods and tools, addressing challenges such as data heterogeneity, enhancing interoperability between databases, and incorporating cutting-edge technologies like deep learning for more accurate predictions is essential. Collaborative efforts, as demonstrated by databases like Mentha and IntAct, highlight the benefits of data sharing, standardization, and mutual support within the scientific community. Encouraging more collaborations is crucial for further advancements. Continuous progress and the integration of multi-omics data are anticipated to reshape the biomedical research landscape, opening new avenues for transformative discoveries.
Acknowledgement: None.
Funding Statement: This work was supported by EU funding within the NextGenerationEU-MUR PNRR Extended Partnership Initiative on Emerging Infectious Diseases (Project No. PE00000007, INF-ACT). A. Irfan extends his appreciation to the Deanship of Scientific Research at King Khalid University Saudi Arabia for funding through the Small Research Group Project under Grant Number RGP1/89/44.
Author Contributions: The authors confirm their contribution to the paper as follows: study conception and design: MA; data collection: MA, AM; analysis and interpretation of results: MA, NR, AM; draft manuscript preparation: MA, AI, NR, AM. All authors reviewed the results and approved the final version of the manuscript.
Availability of Data and Materials: Data sharing is not applicable to this article as no datasets were generated or analyzed during the current study.
Ethics Approval: Not applicable.
Conflicts of Interest: The authors declare that they have no conflicts of interest to report regarding the present study.
References
1. Priyamvada P, Debroy R, Anbarasu A, Ramaiah S. A comprehensive review on genomics, systems biology, and structural biology approaches for combating antimicrobial resistance in ESKAPE pathogens: computational tools and recent advancements. World J Microbiol Biotechnol. 2022;38(9):153. doi:10.1007/s11274-022-03343-z [Google Scholar] [PubMed] [CrossRef]
2. Santos MVC, Feltrin AS, Costa-Amaral IC, Teixeira LR, Perini JA, Martins DCJr., et al. Network analysis of biomarkers associated with occupational exposure to benzene and malathion. Int J Mol Sci. 2023;24(11):9415. doi:10.3390/ijms24119415 [Google Scholar] [PubMed] [CrossRef]
3. Chen L, Wang RS, Zhang XS. Biomolecular networks: methods and applications in systems biology. USA: John Wiley & Sons; 2009. [Google Scholar]
4. Poluri KM, Gulati K, Sarkar S, Poluri KM, Gulati K, Sarkar S. Prediction, analysis, visualization, and storage of protein–protein interactions using computational approaches. In: Protein-protein interactions: Principles techniques, vol. I. Singapore: Springer; 2021. p. 265–346. [Google Scholar]
5. Kuzmanov U, Emili A. Protein-protein interaction networks: probing disease mechanisms using model systems. Genome Med. 2013;5(4):37. doi:10.1186/gm441 [Google Scholar] [PubMed] [CrossRef]
6. Liu ZP, Chen L. Proteome-wide prediction of protein-protein interactions from high-throughput data. Protein Cell. 2012;3(7):508–20. doi:10.1007/s13238-012-2945-1 [Google Scholar] [PubMed] [CrossRef]
7. Stark C, Breitkreutz BJ, Reguly T, Boucher L, Breitkreutz A, Tyers M. BioGRID: a general repository for interaction datasets. Nucleic Acids Res. 2006;34:D535–9. doi:10.1093/nar/gkj109 [Google Scholar] [PubMed] [CrossRef]
8. Mewes HW, Frishman D, Gruber C, Geier B, Haase D, Kaps A, et al. MIPS: a database for genomes and protein sequences. Nucleic Acids Res. 2000;28(1):37–40. doi:10.1093/nar/28.1.37 [Google Scholar] [PubMed] [CrossRef]
9. Franceschini A, Szklarczyk D, Frankild S, Kuhn M, Simonovic M, Roth A, et al. STRING v9.1: protein-protein interaction networks, with increased coverage and integration. Nucleic Acids Res. 2013;41:D808–15. doi:10.1093/nar/gks1094 [Google Scholar] [PubMed] [CrossRef]
10. Breitkreutz BJ, Stark C, Reguly T, Boucher L, Breitkreutz A, Livstone M, et al. The BioGRID interaction database: 2008 update. Nucleic Acids Res. 2008;36:D637–40. doi:10.1093/nar/gkm1001 [Google Scholar] [PubMed] [CrossRef]
11. Szklarczyk D, Gable AL, Lyon D, Junge A, Wyder S, Huerta-Cepas J, et al. STRING v11: protein-protein association networks with increased coverage, supporting functional discovery in genome-wide experimental datasets. Nucleic Acids Res. 2019;47(D1):D607–13. doi:10.1093/nar/gky1131 [Google Scholar] [PubMed] [CrossRef]
12. Bader GD, Betel D, Hogue CW. BIND: the biomolecular interaction network database. Nucleic Acids Res. 2003;31(1):248–50. doi:10.1093/nar/gkg056 [Google Scholar] [PubMed] [CrossRef]
13. Shoemaker BA, Panchenko AR. Deciphering protein–protein interactions. Part I. Experimental techniques and databases. PLoS Comput Biol. 2007;3(3):e42. doi:10.1371/journal.pcbi.0030042 [Google Scholar] [PubMed] [CrossRef]
14. Calderone A, Castagnoli L, Cesareni G. Mentha: a resource for browsing integrated protein-interaction networks. Nat Methods. 2013;10(8):690–1. doi:10.1038/nmeth.2561 [Google Scholar] [PubMed] [CrossRef]
15. Hermjakob H, Montecchi-Palazzi L, Lewington C, Mudali S, Kerrien S, Orchard S, et al. IntAct: an open source molecular interaction database. Nucleic Acids Res. 2004;32:D452–5. doi:10.1093/nar/gkh052 [Google Scholar] [PubMed] [CrossRef]
16. Salwinski L, Miller CS, Smith AJ, Pettit FK, Bowie JU, Eisenberg D. The database of interacting proteins: 2004 update. Nucleic Acids Res. 2004;32:D449–51. doi:10.1093/nar/gkh086 [Google Scholar] [PubMed] [CrossRef]
17. Chatr-aryamontri A, Ceol A, Palazzi LM, Nardelli G, Schneider MV, Castagnoli L, et al. MINT: the molecular INTeraction database. Nucleic Acids Res. 2007;35:D572–4. doi:10.1093/nar/gkl950 [Google Scholar] [PubMed] [CrossRef]
18. Licata L, Briganti L, Peluso D, Perfetto L, Iannuccelli M, Galeota E, et al. MINT, the molecular interaction database: 2012 update. Nucleic Acids Res. 2012;40:D857–61. doi:10.1093/nar/gkr930 [Google Scholar] [PubMed] [CrossRef]
19. Keshava Prasad T, Goel R, Kandasamy K, Keerthikumar S, Kumar S, Mathivanan S, et al. Human protein reference database—2009 update. Nucleic Acids Res. 2009;37(suppl_1):D767–D72. doi:10.1093/nar/gkn892 [Google Scholar] [PubMed] [CrossRef]
20. Bajpai AK, Davuluri S, Tiwary K, Narayanan S, Oguru S, Basavaraju K, et al. Systematic comparison of the protein-protein interaction databases from a user’s perspective. J Biomed Inform. 2020;103:103380. doi:10.1016/j.jbi.2020.103380 [Google Scholar] [PubMed] [CrossRef]
21. Schwikowski B, Uetz P, Fields S. A network of protein-protein interactions in yeast. Nat Biotechnol. 2000;18(12):1257–61. doi:10.1038/82360 [Google Scholar] [PubMed] [CrossRef]
22. Kim CY, Baek S, Cha J, Yang S, Kim E, Marcotte EM, et al. HumanNet v3: an improved database of human gene networks for disease research. Nucleic Acids Res. 2022;50(D1):D632–9. doi:10.1093/nar/gkab1048 [Google Scholar] [PubMed] [CrossRef]
23. Persson E, Castresana-Aguirre M, Buzzao D, Guala D, Sonnhammer EL. FunCoup 5: functional association networks in all domains of life, supporting directed links and tissue-specificity. J Mol Biol. 2021;433(11):166835. doi:10.1016/j.jmb.2021.166835 [Google Scholar] [PubMed] [CrossRef]
24. Kanehisa M, Goto S. KEGG: kyoto encyclopedia of genes and genomes. Nucleic Acids Res. 2000;28(1):27–30. doi:10.1093/nar/28.1.27 [Google Scholar] [PubMed] [CrossRef]
25. Joshi-Tope G, Gillespie M, Vastrik I, D’Eustachio P, Schmidt E, de Bono B, et al. Reactome: a knowledgebase of biological pathways. Nucleic Acids Res. 2005;33(suppl_1):D428–32. doi:10.1093/nar/gki072 [Google Scholar] [PubMed] [CrossRef]
26. Fabregat A, Jupe S, Matthews L, Sidiropoulos K, Gillespie M, Garapati P, et al. The reactome pathway knowledgebase. Nucleic Acids Res. 2018;46(D1):D649–55. doi:10.1093/nar/gkx1132 [Google Scholar] [PubMed] [CrossRef]
27. Glinsky G. Disease phenocode analysis identifies SNP-guided microRNA maps (MirMaps) associated with human “master” disease genes. Cell Cycle. 2008;7:3680–94. doi:10.4161/cc.7.23.7153 [Google Scholar] [PubMed] [CrossRef]
28. Saccone SF, Saccone NL, Swan GE, Madden PA, Goate AM, Rice JP, et al. Systematic biological prioritization after a genome-wide association study: an application to nicotine dependence. Bioinformatics. 2008;24(16):1805–11 [Google Scholar] [PubMed]
29. Landrum MJ, Chitipiralla S, Brown GR, Chen C, Gu B, Hart J, et al. ClinVar: improvements to accessing data. Nucleic Acids Res. 2020;48(D1):D835–44. doi:10.1093/nar/gkz972 [Google Scholar] [PubMed] [CrossRef]
30. Piñero J, Bravo À., Queralt-Rosinach N, Gutiérrez-Sacristán A, Deu-Pons J, Centeno E, et al. DisGeNET: a comprehensive platform integrating information on human disease-associated genes and variants. Nucleic Acids Res. 2017;45(D1):D833–9. [Google Scholar]
31. Thorn CF, Klein TE, Altman RB. PharmGKB: the pharmacogenomics knowledge base. Methods Mol Biol. 2013;1015:311–20. doi:10.1007/978-1-62703-435-7. [Google Scholar] [CrossRef]
32. Becker KG, Barnes KC, Bright TJ, Wang SA. The genetic association database. Nat Genet. 2004;36(5):431–2. doi:10.1038/ng0504-431 [Google Scholar] [PubMed] [CrossRef]
33. Espe S. MalaCards: the human disease database. J Med Libr Assoc. 2018;106(1):140–1. doi:10.5195/jmla.2018.253. [Google Scholar] [CrossRef]
34. Bamford S, Dawson E, Forbes S, Clements J, Pettett R, Dogan A, et al. The COSMIC (Catalogue of Somatic Mutations in Cancer) database and website. Br J Cancer. 2004;91(2):355–8. doi:10.1038/sj.bjc.6601894 [Google Scholar] [PubMed] [CrossRef]
35. Stenson PD, Mort M, Ball EV, Chapman M, Evans K, Azevedo L, et al. The human gene mutation database (HGMD®optimizing its use in a clinical diagnostic or research setting. Hum Genet. 2020;139(10):1197–207. doi:10.1007/s00439-020-02199-3 [Google Scholar] [PubMed] [CrossRef]
36. Gutiérrez-Sacristán A, Grosdidier S, Valverde O, Torrens M, Bravo A, Pinero J, et al. PsyGeNET: a knowledge platform on psychiatric disorders and their genes. Bioinform. 2015;31(18):3075–7. doi:10.1093/bioinformatics/btv301 [Google Scholar] [PubMed] [CrossRef]
37. Grissa D, Junge A, Oprea TI, Jensen LJ. Diseases 2.0: a weekly updated database of disease-gene associations from text mining and data integration. Database. 2022;2022:baac019. doi:10.1093/database/baac019 [Google Scholar] [PubMed] [CrossRef]
38. Zhang L, Hu K, Tang Y. Predicting disease-related genes by topological similarity in human protein-protein interaction network. Cent Eur J Phys. 2010;8:672–82. [Google Scholar]
39. Bruno AE, Li L, Kalabus JL, Pan Y, Yu A, Hu Z. miRdSNP: a database of disease-associated SNPs and microRNA target sites on 3’UTRs of human genes. BMC Genomics. 2012;13:1–7. doi:10.1186/1471-2164-13-44 [Google Scholar] [PubMed] [CrossRef]
40. Wellcome Trust Case Control Consortium. Genome-wide association study of 14,000 cases of seven common diseases and 3,000 shared controls. Nat. 2007;447(7145):661–78. doi:10.1038/nature05911 [Google Scholar] [PubMed] [CrossRef]
41. Pinero J, Bravo A, Queralt-Rosinach N, Gutierrez-Sacristan A, Deu-Pons J, Centeno E, et al. DisGeNET: a comprehensive platform integrating information on human disease-associated genes and variants. Nucleic Acids Res. 2017;45(D1):D833–D9 [Google Scholar] [PubMed]
42. Becker KG, Barnes KC, Bright TJ, Wang SA. The genetic association database. Nat Genetics. 2004;36(5):431–2 [Google Scholar] [PubMed]
43. Consortium GO. The gene ontology (GO) database and informatics resource. Nucleic Acids Res. 2004;32(suppl_1):D258–61. [Google Scholar]
44. Dennis GJr., Sherman BT, Hosack DA, Yang J, Gao W, Lane HC, et al. DAVID: database for annotation, visualization, and integrated discovery. Genome Biol. 2003;4(5):R60. [Google Scholar]
45. Chen EY, Tan CM, Kou Y, Duan Q, Wang Z, Meirelles GV, et al. Enrichr: interactive and collaborative HTML5 gene list enrichment analysis tool. BMC Bioinform. 2013;14(1):128. [Google Scholar]
46. Raudvere U, Kolberg L, Kuzmin I, Arak T, Adler P, Peterson H, et al. G: profiler: a web server for functional enrichment analysis and conversions of gene lists (2019 update). Nucleic Acids Res. 2019;47(W1):W191–8 [Google Scholar] [PubMed]
47. Zheng J, Su Z, Kong Y, Lin Q, Liu H, Wang Y, et al. LncRNAs predicted to interfere with the gene regulation activity of miR-637 and miR-196a-5p in GBM. Front Oncol. 2020;10:303 [Google Scholar] [PubMed]
48. Clough E, Barrett T. The gene expression omnibus database. Statistical Genomics: Methods and Protocols. 2016;93–110. [Google Scholar]
49. Kuhn M, von Mering C, Campillos M, Jensen LJ, Bork P. STITCH: interaction networks of chemicals and proteins. Nucleic Acids Research. 2007;36(suppl_1):D684–8 [Google Scholar] [PubMed]
50. Szklarczyk D, Santos A, von Mering C, Jensen LJ, Bork P, Kuhn M. STITCH 5: augmenting protein-chemical interaction networks with tissue and affinity data. Nucleic Acids Res. 2016;44(D1):D380–4. doi:10.1093/nar/gkv1277 [Google Scholar] [PubMed] [CrossRef]
51. O’reilly LP, Long OS, Cobanoglu MC, Benson JA, Luke CJ, Miedel MT, et al. A genome-wide RNAi screen identifies potential drug targets in a C. elegans model of α1-antitrypsin deficiency. Hum Mol Genet. 2014;23(19):5123–32. doi:10.1093/hmg/ddu236 [Google Scholar] [PubMed] [CrossRef]
52. Kumar KK, Lowe EWJr, Aboud AA, Neely MD, Redha R, Bauer JA, et al. Cellular manganese content is developmentally regulated in human dopaminergic neurons. Sci Rep. 2014;4(1):1–8. doi:10.1038/srep06801 [Google Scholar] [PubMed] [CrossRef]
53. Xu J, Li YJB. Discovering disease-genes by topological features in human protein-protein interaction network. Bioinform. 2006;22(22):2800–5. doi:10.1093/bioinformatics/btl467 [Google Scholar] [PubMed] [CrossRef]
54. López-Bigas N, Ouzounis C. Genome-wide identification of genes likely to be involved in human genetic disease. Nucleic Acids Res. 2004;32(10):3108–14. doi:10.1093/nar/gkh605 [Google Scholar] [PubMed] [CrossRef]
55. Van Driel MA, Cuelenaere K, Kemmeren PP, Leunissen JA, Brunner HG. A new web-based data mining tool for the identification of candidate genes for human genetic disorders. Eur J Hum Genet. 2003;11(1):57–63. doi:10.1038/sj.ejhg.5200918 [Google Scholar] [PubMed] [CrossRef]
56. Kaushal P, Singh S. Network-based disease gene prioritization based on protein-protein interaction networks. Netw Model Anal Hlth. 2020;9:1–16. doi:10.1007/s13721-020-00260-9. [Google Scholar] [CrossRef]
57. Kim Y, Park JH, Cho YR. Network-based approaches for disease-gene association prediction using protein-protein interaction networks. Int J Mol Sci. 2022;23(13):7411. doi:10.3390/ijms23137411 [Google Scholar] [PubMed] [CrossRef]
58. Ideker T, Sharan R. Protein networks in disease. Genome Res. 2008;18(4):644–52. doi:10.1101/gr.071852.107 [Google Scholar] [PubMed] [CrossRef]
59. Tu Z, Wang L, Xu M, Zhou X, Chen T, Sun F. Further understanding human disease genes by comparing with housekeeping genes and other genes. BMC Genomics. 2006;7(1):1–13. doi:10.1186/1471-2164-7-31 [Google Scholar] [PubMed] [CrossRef]
60. Oti M, Snel B, Huynen MA, Brunner HG. Predicting disease genes using protein-protein interactions. J Med Genet. 2006;43(8):691–8 [Google Scholar] [PubMed]
61. Leicht EA, Holme P, Newman ME. Vertex similarity in networks. Phys Rev E Stat Nonlin Soft Matter Phys. 2006;73(2 Pt 2):26120. [Google Scholar]
62. Suman S, Mishra A. An interaction network driven approach for identifying biomarkers for progressing cervical intraepithelial neoplasia. Sci Rep. 2018;8(1):12927 [Google Scholar] [PubMed]
63. Dasgupta S, Das SS, Kar A, Choudhury P, Mitra I, Mukherjee G, et al. Transcriptome analysis for the screening of hub genes and potential drugs in hypersensitivity pneumonitis. Human Gene. 2023;37:201208. [Google Scholar]
64. Oughtred R, Rust J, Chang C, Breitkreutz BJ, Stark C, Willems A, et al. The BioGRID database: a comprehensive biomedical resource of curated protein, genetic, and chemical interactions. Protein Sci. 2021;30(1):187–200 [Google Scholar] [PubMed]
65. Oughtred R, Stark C, Breitkreutz BJ, Rust J, Boucher L et al. The BioGRID interaction database: 2019 update. Nucleic Acids Res. 2019;47(D1):D529–41 [Google Scholar] [PubMed]
66. Roussarie JP, Yao V, Rodriguez-Rodriguez P, Oughtred R, Rust J, Plautz Z, et al. Selective neuronal vulnerability in Alzheimer’s disease: a network-based analysis. J Neuron. 2020;107(5):821–35.E12. doi:10.1016/j.neuron.2020.06.010 [Google Scholar] [PubMed] [CrossRef]
67. Parmar M, Thumar R, Patel B, Athar M, Jha PC, Patel D. Structural differences in 3C-like protease (Mpro) from SARS-CoV and SARS-CoV-2: molecular insights revealed by molecular dynamics simulations. Struct Chem. 2023;34(4):1309–26. doi:10.1007/s11224-022-02089-6 [Google Scholar] [PubMed] [CrossRef]
68. Jumper J, Evans R, Pritzel A, Green T, Figurnov M, Ronneberger O, et al. Highly accurate protein structure prediction with AlphaFold. Nat. 2021;596(7873):583–9. doi:10.1038/s41586-021-03819-2 [Google Scholar] [PubMed] [CrossRef]
69. Qiao Z, Nie W, Vahdat A, Miller TF, Anandkumar A. State-specific protein-ligand complex structure prediction with a multiscale deep generative model. Nat Mach Intell. 2024;6(2):195–208. doi:10.1038/s42256-024-00792-z. [Google Scholar] [CrossRef]
70. Baek M, DiMaio F, Anishchenko I, Dauparas J, Ovchinnikov S, Lee GR, et al. Accurate prediction of protein structures and interactions using a three-track neural network. Sci. 2021;373(6557):871–6. doi:10.1126/science.abj8754 [Google Scholar] [PubMed] [CrossRef]
71. Buel GR, Walters KJ. Can AlphaFold2 predict the impact of missense mutations on structure? Nat Struct Mol Biol. 2022;29(1):1–2. doi:10.1038/s41594-021-00714-2 [Google Scholar] [PubMed] [CrossRef]
72. Sebastiano MR, Ermondi G, Hadano S, Caron GJDDT. AI-based protein structure databases have the potential to accelerate rare diseases research: AlphaFoldDB and the case of IAHSP/Alsin. Drug Discovery Today. 2022;27(6):1652–60. [Google Scholar]
Cite This Article
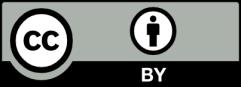
This work is licensed under a Creative Commons Attribution 4.0 International License , which permits unrestricted use, distribution, and reproduction in any medium, provided the original work is properly cited.