Open Access
ARTICLE
Bioinformatics comprehensive analysis confirmed the potential involvement of SLC22A1 in lower-grade glioma progression and prognosis
1 Guizhou Provincial College-Based Key Lab for Tumor Prevention and Treatment with Distinctive Medicines, Zunyi Medical University, Zunyi, 563000, China
2 College of Basic Medicine, Zunyi Medical University, Zunyi, 563000, China
3 Department of Dermatology, Guizhou Province Cosmetic Plastic Surgery Hospital, Affiliated Hospital of Zunyi Medical University, Zunyi, 563000, China
4 School of Forensic Medicine, Zunyi Medical University, Zunyi, 563000, China
* Corresponding Authors: QINGHONG KONG. Email: ; YUN LIU. Email:
BIOCELL 2024, 48(5), 803-815. https://doi.org/10.32604/biocell.2024.047122
Received 25 October 2023; Accepted 05 March 2024; Issue published 06 May 2024
Abstract
Background: Although it has been established that the human Solute Carrier Family 22 (SLC22) functions as a cationic transporter, influencing cellular biological metabolism by modulating the uptake of various cations, its impact on cancer prognosis remains unclear. Methods: We conducted a comprehensive analysis utilizing data from The Cancer Genome Atlas (TCGA) and other databases to assess the prognostic value and functional implications across various tumors. Silence of SLC22A1 RNA in glioma U251 cells was performed to access the impact of SLC22A1 on lower-grade glioma (LGG) progression. Results: Our findings demonstrated a significant correlation between SLC22A1 expression and the survival time of patients with various cancers (p < 0.05). Importantly, we found the potential involvement of SLC22A1 in occurrence and progress of certain cancers, with a pronounced impact on LGG. Further examination of the SLC22A1-LGG relationship revealed its status as an independent risk factor for LGG, suggesting its potential involvement in regulating diverse immune pathways and metabolic activities. Chinese Glioma Genome Atlas (CGGA) data supported the reliability of the risk score as a prognostic and recurrence indicator, emphasizing the accuracy of the nomograph (1, 3, and 5-year-AUC >0.8). Cell proliferation and clone formation experiment proved that decreased expression of SLC22A1 in glioma U251 cells inhibited glioma cell growth. Conclusion: Our findings suggest SLC22A1 has huge prospects as a promising biomarker and therapeutic target for LGG prognosis. SLC22A1 has only been proved to support cellular function previously. Our findings demonstrated a robust connection between the tumor microenvironment and functional proteins that maintain basal cell metabolism, which gifts unique tumor immune characteristics of gliomas. Additionally, we provide a highly practical prediction model for estimating the survival rate of LGG patients.Keywords
Abbreviations
PD1 | Programmed cell death protein 1 |
CTLA4 | Cytotoxic T-lymphocyte-associated protein 4 |
GO | Gene Ontology |
KEGG | Kyoto Encyclopedia of Genes and Genomes |
GDAC | Geneomics data alta commons |
ACTB | Beta-actin |
MLH1 | MutL homolog 1 |
MSH2 | MutS homolog 2 |
MSH6 | MutS homolog 6 |
PMS2 | Postmeiotic segregation increased 2 |
DNMT1 | DNA (cytosine-5-)- methyltransferase 1 |
DNMT3A | DNA methyltransferase3alpha |
DNMT3B | DNA methyltransferase3beta |
DNMT3L | DNA (cytosine-5-)-methyltransferase 3-like |
ABCG2 | ATP-binding cassette sub-family G member 2 |
ABCB1 | ATP-binding cassette sub-family B member 1 |
ADH1A | Alcohol dehydrogenase 1A |
The human Solute Carrier Family 22 (SLC22) belongs to the membrane transporter family, with the SLC22A subtype collectively known as organic cation transporters (OCTs). Current evidence suggests that OCTs play a crucial role in drug and endogenous compound disposal and clearance, holding significant physiological and pharmacological research value. These transporters exhibit multiple specificity and easy diffusion characteristics, mediating the transport of various positively charged substances and drugs across tissues and organs [1]. In the human brain, common substrates for OCT1-3 include neurotoxin 1-methyl-4-phenylpyridine (MPP+) and endogenous compounds such as 5-hydroxytryptamine (5-HT), norepinephrine (NE), histamine, and guanidine. While all OCTs, except for OCT1, effectively transport epinephrine (EP), histamine and 5-HT are predominantly absorbed by OCT2 and OCT3, with OCT2 and OCT3 displaying the highest uptake efficiency for EP and histamine. Sequence homology and substrate selection specificity among various OCT subtypes significantly impact their transport of endogenous substances and drugs, influencing metabolic kinetics [2,3]. Clinically, SLC22A1 can affect the therapeutic outcomes of diseases, including diabetes, liver cancer, and chronic leukemia, by transporting drugs such as metformin, sorafenib, and imatinib [4–7]. Besides, imatinib usage has been shown to influence the expression of SLC22A1 [8]. The expression of SLC22A1 could affect blood sugar and blood fat concentrations [9–11], suggesting a potential bidirectional influence between SLC22A1 expression and the extracellular environment. However, the relationship between SLC22A1, as a membrane protein, and the tumor microenvironment (TME) remains unclear.
It is now understood that the TME comprises cancer cells, immune cells, and cytokines, playing a pivotal role in the occurrence and progression of certain types of cancer [12,13]. The TME induces changes in the biological activity and metabolic processes of cancer cells, reciprocally influencing the functions of cytokines and immune cells within the microenvironment [12,14]. Tumor immunity, focusing on the interplay between tumor antigens, immune function, and tumor progression, is a critical aspect of cancer treatment. Over the years, researchers have dedicated efforts to understanding the mechanisms of tumor immune response and escape, as well as developing strategies for tumor immune diagnosis and prevention. Immunotherapeutic drugs targeting checkpoints, such as Programmed cell death protein 1 (PD1) and Cytotoxic T-lymphocyte-associated protein 4 (CTLA4) inhibitors, have been utilized in treating various cancers [15–18]. Therefore, identifying new biomarkers is essential to help patients choose more effective immunotherapy methods and drugs.
In recent years, research on establishing disease diagnosis models or analyzing pathogenesis through mathematics based on bioinformatics in the fields of biology and medicine has been common [19–21]. This paper aims to analyze the role of SLC22A1 in various tumors to evaluate its potential as a prognostic marker and therapeutic target. Leveraging numerous public databases, we examined the prognostic value of SLC22A1 and its correlation with several cancer-related genes. Additionally, we investigated the effect of the methylation level of SLC22A1 on the prognosis of cancer patients and analyzed anti-cancer drug sensitivity. Across diverse cancers, SLC22A1 exhibited the strongest correlation with low-grade glioma (LGG). Interestingly, the presence of an intracranial barrier creates a distinctive environment in brain tissue. In neural tumors like glioma, research has demonstrated a close relationship between growth and the tumor microenvironment [22]. Further investigation into LGG revealed that SLC22A1 might influence the clinical survival time of LGG patients through the tumor immunity process, involving extracellular tumor-infiltrating immune cells (TIICs), intracellular tumor immune checkpoints, and immune responses. The research suggests that SLC22A1 could serve as a prognostic biomarker and therapeutic target for LGG. Additionally, we constructed a reliable predictive survival model for LGG patients and introduced a potential new indicator, independent of histology, to assess the development of LGG.
The cancer genome atlas (TCGA, https://www.cancer.gov/about-nci/organization/ccg/research/structural-genomics/tcga) is a project launched by the National Cancer Institute (NCI) and the National Human Genome Research Institute (NHGRI) in 2006. The database contains multiple data of more than 20000 samples of 33 cancers, as the largest cancer gene database at present, it is the base of many bioinformatics researches [23].
Chinese Glioma Genome Atlas (CGGA, http://www.cgga.org.cn/) is the first utilitarian genomics database of glioma in Chinese population. It is a 15 year accumulation of clinical samples constructed by the Beijing Institute of Neurosurgery, which has released functional genomics data of more than 2000 Chinese glioma samples. This acticle used it as a verification set to test the function of SLC22A1 in LGG [24].
The standardized gene expression datasets of TCGA and CGGA databases were downloaded from UCSC website (https://xenabrowser.net/). And the expression data was extracted and processed in R environment. We deleted the missing and duplicate results, and converted the data into log2 (TPM+1) formation. Supplementary prognostic and clinical data came from other literatures [25,26].
COX regression analysis and survival analysis
Based on TCGA database, COX analysis and Kaplan-Meier analysis were performedwith R package “surviver” (0.4.9), and the K-M curves were drawn by “survival” (3.2-10). The cancer patients were divided into two groups on the basis of best cut-off value. The results with p < 0.05 in results represented were considered to significantly affect the survival time of patients, containing overall survival (OS) and disease-specific survival (DSS). Hazard ratio (HR) describes the risk levels of two different groups, used to evaluate the effect of different therapeutic methods and prognostic factors on disease development. HR > 1 represents a factor that is detrimental to patient lifespan, while HR < 1 is the opposite.
Estimating the abundance of TIICs and correlation analysis
Tumor Immune Estimation Resource (TIMER, https://cistrome.shinyapps.io/timer/) database calculates RNA-seq results of pan-cancerin TCGA database based on different algorithms, which is used to infer the composition of immune cells from tumor transcriptome spectrum [27–29]. Using TIMER to evaluate the immune cell infiltration level of various cancers and performed correlation analysis of gene expression by spearman statistical method. The results with p < 0.05 were regarded to have significant correlation.
Protein-protein interaction (PPI) networks and enrichment analysis
PPI network was created to predict gene function by GeneMANIA database (http://genemania.org/). The website collected hundreds of millions of interactions from GEO, BioGRID, IRefIndex and I2D datasets, including protein-protein, protein-DNA and genetic interactions, etc [30]. Enrichment analysis was performed by R package, in which DESeq2 package was used for single gene difference analysis of TCGA data, and enrichment analysis was performed by cluster Profiler package. GSEA used gene set came from MSigDB Collections database (https://www.gsea-msigdb.org/gsea/msigdb/index.jsp). And the top 300 genes co-expressed with SLC22A1 for Gene Ontology (GO) and Kyoto Encyclopedia of Genes and Genomes (KEGG) analysis were found out by cBioportal database (http://www.cbioportal.org/).
Prognostic significance of SLC22A1 methylation
The influence of methylation level of SLC22A1 on the prognosis of cancer patients was completed by Methsurv Tool (https://biit.cs.ut.ee/methsurv/). MethSurv is a network tool for evaluating the relationship between gene methylation and patient prognosis. The data came from TCGA and Geneomics data alta commons (GDAC) Firehose datasets. The results with p < 0.05 were considered to have an appreciable effect on the prognosis of patients.
Processed data sets of RNA-seq and compound activity were obtained from CellMiner database (https://discover.nci.nih.gov/cellminer/home.do) [31]. We extracted the expression of SLC22A1 and pharmacological data (50% inhibiting concentration, IC50) of selected drugs in clinical trial and Parenteral Drug Association (FDA) approved. The missing items were supplemented with mean value and the relevance was analyzed in R package with spearman correlation test. The results with p < 0.05 were deemed to have remarkable relevance.
The Human glioma cell line U251 was obtained from Shanghai Genechem Co. (Shanghai, China). U251 cells were cultured with RPMI 1640 (VivaCell Biosciences, C3001-0500, Shanghai, China) in cell incubator containing 5.0% CO2 and 10% fetal bovine serum (Gibco, 26010074, USA) at 37°C, and logarithmic phase cells were taken for transfection.
Transfection experiments with small interfering RNAs (siRNAs)
Seeding U251 cells into 6-well plates at density of 2 × 104 cells in each well, and transfecting cells on the basis of transfection reagent’ protocol (Genephrma, G04008, Suzhou, China). The cells were treated with 8 × 10−2 nmol specific siRNAs (Genephrma, A10001, Suzhou, China) and 4 µL GP-transfect-Mate reagent (Genephrma, G04008, Suzhou, China) in per mL growth medium. The sequences of human SLC22A1 siRNA were as follows: sense 5′-CCAUUGCAAUACAAAUGAUTT-3′, antisense 5′-AUCAUUUGUAUUGCAAUGGTT-3′.
After 48 h, the efficiency of gene knockout was examined by reverse transcription-qPCR (RT-qPCR). Total RNA was isolated according to the protocol of RNA extraction reagent (Servicebio, G3013, Wuhan, China), then it was reversely transcribed into complementary DNA (cDNA) by HiScript II Q RT SuperMix for qPCR (Vazyme Biotech, R223-01, Nanjing, China) in a PCR instrument (Jena, Biometra Tone 96G, German). According to the standard procedure of ChamQ Universal SYBR qPCR Mater Mix (Vazyme Biotech, Q711, Nanjing, China), RT-qPCR was performed by qPCR instrument (Roche, LightCycler®96, German). ACTB was chosen as the reference gene. The primer sequences of genes were as follows: forward primer of human ACTB 5′-CATGTACGTTGCTATCCAGGC-3′, reverse primer of human ACTB 5′-CTCCTTAATGTCACGCACGAT-3′, forward primer of human SLC22A1 5′-ACGGTGGCGATCATGTACC-3′, reverse primer of human SLC22A1 5′-CCCATTCTTTTGAGCGATGTGG-3′. The gene silencing efficiency was counted by 2−∆∆Ct method.
Sulforhodamine B (SRB) proliferation detection
Seeding U251 cells into 96-well plates at density of 1500 cells per well, and transfecting cells according to section Transfection Experiments (100 µL incubation growth medium). After 48 h, the growth medium was replaced with fresh complete culture medium. Five replicates were made for each siRNA and measured every 24 h.
The supernate was abandoned and 100 µL of precooled 10% trichloroacetic acid (Sigma Aldrich, T0699, Shanghai, China) was added in each well. After washing plates three times with ddH2O, staining cells with 4 mg/mL SRB (Sigma Aldrich, 230162, Shanghai, China) for 15 min and washing plates again with 1% acetic acid five times. We added 100 µL 10 mM Tris-HCl (Sigma Aldrich, 108315, Shanghai, China) per well and tested the absorbance (530 nm).
Inoculating U251 cells into a 6-well plate at a density of 500 cells per well and transfecting cells as described in section Transfection Experiments (600 µL incubation growth medium). The cells were cultured for 8 days and fixed with formaldehyde. Washing plates by ice-cold PBS, the number of colony was counted after Giemsa staining (Sigma Aldrich, G5637, Shanghai, China). Each group had three parallel wells.
In this paper, statistical analysis and visualization were accomplished in R package (Version 3.6.3). “Glmnet” (4.1-2) and “survival” (3.2-10) were used for LASSO analysis, “rms” was applied for nomogram and calibration, and ROC analysis was run by “timeROC”. Furthermore, the results with p < 0.05 were considered to be of statistical significance and ggplot2 package was used for graph plotting. The area under the curve (AUC) is used to measure the model’s discriminative ability, AUC always falls between 0 and 1, AUC > 0.8 indicates high accuracy of the model.
Prognostic analysis of SLC22A1 in multiple cancers
Utilizing TCGA data, Cox analysis was conducted to assess the correlation between SLC22A1 expression and the survival rates of pan-cancer patients. The results demonstrated that SLC22A1 RNA level was related to both poor and improved prognosis (in terms of OS and DSS) in specific cancers. Table 1 outlines the outcomes of the OS analysis, revealing that elevated SLC22A1 expression correlated with poor prognoses in ACC, HNSC, KICH, KIRC, KIRP, LAML, LGG, MESO, and SARC (HR > 1, p < 0.05). Conversely, higher SLC22A1 expression in BLCA, LIHC, and PCPG was associated with better clinical outcomes (HR < 1, p < 0.05). DSS analysis further indicated that increased SLC22A1 levels were significantly correlated with longer disease-specific survival time in LIHC patients (HR < 1, p < 0.05), while in ACC, HNSC, KICH, KIRC, LGG, MESO, and SARC, higher SLC22A1 expression was associated with shorter DSS (HR > 1, p < 0.05).
The relationship between tumor immunity and SLC22A1 level
Next, we intended to assess the influence of SLC22A1 on immune cell recruitment. Using the TIMER tool, we evaluated the relation between SLC22A1 RNA and immune cell infiltration levels, including neutrophils, helper T cells (T cell CD4+), macrophages, myeloid dendritic cells, B cells, and cytotoxic T cells (T cell CD8+) in various cancers. Fig. 1A illustrates the most significant correlations observed in LGG and LIHC. In LIHC, SLC22A1 expression positively correlated with the infiltration of five immune cells, while in LGG, the mRNA level of SLC22A1 was remarkable negatively correlated with infiltration levels of six immune cells. Fig. 1B indicates that higher immune infiltration levels significantly correlated with worse prognosis for LGG patients (p < 0.01), while the survival time of LIHC patients was not affected by immune infiltration levels.
Figure 1: The relations between SLC22A1 expression and immune cells infiltration in various cancers (A). Kaplan-Meier analysis (OS) of immune cells infiltration in LGG and LIHC (B). The relation between SLC22A1 level and gene expression of immune checkpoints evaluated by TIMER database (C). The correlation between SLC22A1 level and expression of mismatch repair (MMR) genes (D). The connection between mRNA levels of SLC22A1 and expression of DNA methyltransferase (E). Protein-Protein Internet (PPI) network of SLC22A1 based on GeneMANIA website (F). All abbreviations of cancers were shown in Table 1.
It is widely thought that the interaction of immune checkpoints can hinder immune cell recognition and the killing of tumor cells, enabling cancer cells to evade immune surveillance [32–34]. Screening 49 immune checkpoints expressed in tumor cells [35], we found that the number of checkpoints positively and negatively related to SLC22A1 expression was highest in LGG and LIHC, respectively (Fig. 1C). Notably, cancers of immune organs (DLBC and TGCT) exhibited the highest coefficients. These findings suggest that SLC22A1 might influence LGG tumor cell development by enhancing immune cell recruitment and immune escape through immune checkpoints.
Relationship between genome stability and expression of SLC22A1
Genetic and epigenetic alterations are common features of cancer cells, and abnormal gene expression can impact the immune landscape within tumors. To investigate the role of SLC22A1 expression in carcinogenesis, we utilized the TIMER database to evaluate the relation between SLC22A1 RNA and the transcription levels of mismatch repair (MMR) enzymes (MutL homolog 1(MLH1), MutS homolog 2 (MSH2), MutS homolog 6 (MSH6), and Postmeiotic segregation increased 2 (PMS2)) as well as methyltransferase enzymes (DNA (cytosine-5-)- methyltransferase 1(DNMT1), DNA methyltransferase3alpha (DNMT3A), DNA methyltransferase3beta (DNMT3B), and DNA (cytosine-5-)-methyltransferase 3-like (DNMT3L)). As shown in Fig. 1D, SLC22A1 expression was associated with at least one MMR gene in many cancers. Notably, in LIHC, the transcription level of all four MMR genes was negatively related with SLC22A1 levels. Fig. 1E indicates a relationship between the expression of methyltransferase enzymes and SLC22A1 expression across various cancers. Especially in KIRC and LGG, SLC22A1 and four methyltransferases exhibited a significantly positive co-expression, while an inverse relationship was observed with PRAD. In summary, SLC22A1 was associated with the genomic stability of various tumors. Given the strongest correlation observed in LGG between SLC22A1 and tumor immunity, it is highly conceivable that SLC22A1 influences the tumor immune environment by affecting DNA methylation levels in LGG.
Protein-protein interaction (PPI) network
To explore the potential mechanism by which SLC22A1 affects the tumor microenvironment, a PPI network involving SLC22A1 was created using the GeneMANIA website. As depicted in Fig. 1F, SLC22A1 exhibited significant physical interactions with ATP-binding cassette sub-family G member 2 (ABCG2), ATP-binding cassette sub-family B member 1 (ABCB1), and Alcohol dehydrogenase 1A (ADH1A). Both ABCG2 and ABCB1 are members of the ATP-binding cassette (ABC) transporter family, crucial for drug transport in the human body. These proteins use ATP hydrolysis to expel various drug molecules from cells, influencing tumor drug resistance. ABCG2 is also a biomarker for various tumor stem cells, impacting the proliferation, invasion, and prognosis of many cancers. ADH1A plays an important role in the redox detoxification metabolic function of the liver and holds potential clinical prognostic value for LIHC patients. Accordingly, our findings suggest that SLC22A1 may interact with drug transporters and tumor stem cell markers, especially ABCG2, affecting cell metabolism and altering the relation between cancer cells and TME.
Prognostic significance of methylation level of SLC22A1
The aforementioned analyses suggested that SLC22A1 might promote development of LGG by affecting the TME. To explore whether changes in SLC22A1 expression affect the prognosis of cancer patients, we assessed the prognostic value of the methylation levels of 12 CpG island sites of SLC22A1 across various cancers using the Methsurv tool. The top 20 most significantly correlated sites, based on p values, are presented in Fig. 2A. The results indicated a significant correlation between SLC22A1 methylation and a favorable prognosis in LGG patients. It is well-documented that DNA methylation, as an epigenetic modification affecting gene expression, predominantly reduces gene expression. Therefore, reducing SLC22A1 expression may potentially prolong the survival of LGG patients.
Figure 2: The top 20 relationships between methylation of SLC22A1 and prognosis of various cancer patients with minimal p value (A). The pathways and targets of 17 anti-tumor drugs which sensitive to SLC22A1 levels (B). COX regression analysis on clinical factors of LGG patients (C & D). The expression of SLC22A1 in different subgroups (E–I). The survival analysis for SLC22A1 expression and prognosis in LGG using data from CGGA database (OS) (J). Asterisks indicate statistical significance based on Wilcoxon rank sum tests. The p values were shown as *p < 0.05, **p < 0.01, ***p < 0.001, ns for not significant.
To further elucidate the impact of SLC22A1 on the tumor microenvironment, we investigated whether it influences the sensitivity of tumor cells to chemotherapy drugs and explored its underlying mechanisms. Analyzing data from the Parenteral Drug Association (PDA) approved and clinical trial anticancer drugs in the CellMiner database, we identified 17 drugs whose IC50 were correlated with SLC22A1 expression in NCI-60 cell lines. The action pathways and targets of these drugs are illustrated in Fig. 2B. Notably, SGI-1027 and CEP-9722, exhibiting the strongest correlations, were effective in inhibiting cancer through epigenetic modification. However, they demonstrated opposite sensitivities to SLC22A1 expression levels, possibly due to their corresponding targets (DNMT and PARP1) playing opposing roles in genome stability. The analysis revealed that the mRNA level of SLC22A1 in cancer patients could impact the sensitivity of anticancer drugs, thereby influencing clinical outcomes, suggesting that SLC22A1 could affect the sensitivity of tumor cells to anticancer drugs through various pathways, especially via epigenetic modification, specifically methylation (DNMT), which has a notable effect on inhibiting tumor cell proliferation, consistent with the findings in Fig. 1E.
Functional analysis of SLC22A1 in LGG
Given the comprehensive impact of SLC22A1 on various tumor-related indicators in pan-cancer, with the strongest association observed in LGG, we sought to validate the function of SLC22A1 in LGG using data from TCGA and CGGA databases. Gliomas were classified into LGG and glioblastoma multiforme (GBM) in both databases (Table 2).
Univariate COX analysis revealed that tumor grade, age, isocitrate dehydrogenase (IDH) status, 1p/19q codeletion, biological type, and SLC22A1 expression were associated with OS in LGG patients (Fig. 2C). Multivariate analysis identified grade, age, IDH status, and SLC22A1 as independent risk factors for LGG (p < 0.05, HR > 1, Fig. 2D). SLC22A1 expression varied across clinical subgroups, including grade, 1p/19q codeletion, and biological type (Figs. 2E–2I). Kaplan-Meier analysis of CGGA data confirmed a significant association between increased SLC22A1 expression and worse prognosis in LGG patients (p = 0.009, Fig. 2J). Taken together, these results underscored the potential of SLC22A1 as a prognostic marker for LGG.
Functional enrichment analysis
To understand the role of SLC22A1 in LGG, pathway enrichment analysis was conducted. As shown in Fig. 3A, the top 20 pathways from Gene Set Enrichment Analysis (GSEA) demonstrated that the high-expression SLC22A1 group was significantly associated with enhanced immune reactions, extracellular material recognition, and cell proliferation. Pathways included neutrophil degranulation, signaling by interleukins, interferon signaling, IL18 signaling pathway, extracellular matrix organization, cell surface interaction at the vascular, cytokine receptor interaction, extracellular matrix (ECM) regulators, mitotic metaphase and anaphase, mitotic prometaphase, cell cycle checkpoints, and separation of sister chromatids, among others.
Figure 3: The top 20 results of Gene set enrichment analysis (GSEA) (A). GO analysis of the top 500 genes co-related with SLC22A1 (B). KEGG analysis of the top 500 genes co-related with SLC22A1 (C).
The top 500 genes associated with SLC22A1 were annotated in biological processes and pathways related to immunoregulation and immune response. These included regulation of innate immune response, nuclear factor (NF-kappaB) signaling, response to interferon-gamma, alpha-beta T cell activation, positive regulation of response to cytokine stimulus, positive regulation of cytokine-mediated signaling pathway, NOD-like receptor signaling pathway, and TNF signaling pathway (Figs. 3B, 3C). Collectively, these results indicated that SLC22A1 might influence the prognosis of LGG through immunization routes.
Evaluation of the prognostic value of SLC22A1-related immune checkpoints and development of a risk score prognosis model
Univariate COX regression analysis identified 26 genes among 33 immune checkpoints associated with survival time (Table 3). Utilizing LASSO and the COX proportional hazards model, we identified three immune regulatory factors with independent predictive value and established a risk score prognosis model (Figs. 4A–4D, with CD276 included due to its p value being close to 0.05). The risk score was calculated by summing the product of the coefficient and gene expression, resulting in the scoring formula: risk score = 0.5960 * TNFRSF14 + 0.7947 * CEACAM1 + 0.4087 * CD276. A higher number of LGG patients died in the high-risk score group, with the three risk factors showing increased expression in the high-risk group (Fig. 4E). Kaplan-Meier curves further validated that LGG patients with a low risk score had significantly longer survival times than those with a high risk score (p < 0.001, Fig. 4F), consistent with overall survival curves based on the CGGA database (p < 0.001, Fig. 4G). Additionally, the risk score of recurrent LGG patients in the CGGA database was significantly higher than that of primary LGG patients (Fig. 4H, p < 0.001), suggesting that a high risk score might indicate a higher probability of recurrence. Hence, the risk score holds clinical significance in evaluating the likelihood of relapse in LGG patients. Consequently, we established an immune checkpoint risk score model related to SLC22A1, and the survival outcomes and curves demonstrated that a higher risk score implied less favorable survival of LGG patients.
Figure 4: Lasso analysis of 26 immune checkpoints with prognosis value (A and B). Using COX regression to analyze whether 5 genes have independent prognostic function (C). A risk score prognosis model based on COX Proportional Hazards Model (D). Risk factor graph on account of the risk score model (E). The survival analysis for risk score in LGG using data from TCGA database (F). Kaplan-Meier analysis for risk score in LGG using data from CGGA database (G). Comparison of risk score in primary LGG patients and recurrent LGG patients (H). All results were expressed as the mean ± SD. Asterisks indicate statistical significance based on Wilcoxon rank sum tests. Wilcoxon test was used for data analysis. The p values were shown as ***p < 0.001.
Development and validation of nomogram
The hazard ratios of different patient features indicated that age, grade, and risk score were independent risk factors for the prognosis of LGG patients (Fig. 5A). We next exploited a nomogram model to predict the survival time of LGG patients using risk score and age, as illustrated in Fig. 5B. Calibration curves verified the reliability of the nomogram in predicting the 1-year, 3-year, and 5-year survival rates of LGG patients (Figs. 5C–5E). ROC curves were employed to assess the prediction accuracy of the nomogram, revealing AUC values of 0.890, 0.878, and 0.808 for 1 year, 3 years, and 5 years, respectively (Fig. 5G). In comparison to the AUC values of the risk score alone (Fig. 5F), this demonstrated that the nomogram had better accuracy. Furthermore, the prognostic model was tested with CGGA data, yielding AUC values of 0.807, 0.813, and 0.811 for 1 year, 3 years, and 5 years, respectively (Fig. 5H), indicating good accuracy in practical application. The results substantiated that the nomogram, established based on risk scores and age, exhibited a strong ability to predict the survival rate of LGG patients.
Figure 5: COX regression analysis of clinical characters (A). Nomogram to predicted survival probability of LGG patients (B). Calibration curves to evaluate the predictive value ofnomogram (C–E). AUC values of risk score in 1 year, 3 year and 5 year (F). AUC analysis of nomogram in 1 year, 3 year and 5 year (G). ROC curves to test the precision of nomogram based on CGGA database (H).
Effect of silencing SLC22A1 on proliferation of glioma U251 cells
To verify the effect of silencing SLC22A1 on proliferation gliomas U251 cells, we reduced the expression of SLC22A1 using siRNA and detected the cell proliferation. In Fig. 6A, fluorescent labeled siRNA showed that 48 h incubation time was considered to exhibited high transfection efficiency. And RT-qPCR assay confirmed that mRNA level of SLC22A1 in gliomas U251 cells was successfully silenced by SLC22A1 siRNA (Fig. 6B). As shown in Fig. 6C, the proliferation rate of U251 cells treated with SLC22A1 siRNA was significantly lower than that treated with NC siRNA. And equally the cloning efficiency of U251 cells decreased after inhibition of SLC22A1 expression (Figs. 6D and 6E). As our prediction, the decrease of SLC22A1 RNA levels depressed the growth of gliomas U251 cells.
Figure 6: The photos of U251 cells treated by FAM-NC siRNA for 48 h (A). The silencing efficiency of SLC22A1 displayed by RT-qPCR (B). The proliferation efficiency of U251 cells transfected with NC siRNA and SLC22A1 siRNA (C). Clone formation experiments shed light on the effects of SLC22A1 down-regulation on proliferation (D and E). These results are mean ± SD of three independent experiments performed in triplicate. *p < or **p < 0.01 vs. control (one-way ANOVA followed by a Student-Newman-Keuls test).
SLC22A1, a cation transporter, has been recognized for its influence on the therapeutic outcomes of various diseases. However, its role in tumor development has remained unclear. This study aimed to elucidate the correlation between SLC22A1 expression and the prognosis of patients across different cancers and to investigate potential underlying mechanisms. Our findings revealed that elevated SLC22A1 expression was associated with prolonged survival in LIHC patients but correlated with poor prognosis in ACC, HNSC, KICH, KIRC, LGG, MESO, and SARC (both OS and DSS). Correlation analyses demonstrated that SLC22A1 levels were closely linked to various indicators of the tumor immune environment and the stability of intracellular genes, including immune infiltration levels, immune checkpoints, DNA mismatch repair enzymes, and DNA methylase. These findings position SLC22A1 as a potential prognostic biomarker for a range of cancers, including LGG and LIHC. Additionally, PPI analysis indicated that SLC22A1 had significant physical interactions with proteins closely associated with tumor development. Methylation of SLC22A1 was shown to impact the survival rate of many cancer patients, and drug sensitivity analysis revealed correlations between SLC22A1 expression and the expected efficacy of 18 cancer drugs. Consequently, SLC22A1 may influence the tumor immune environment through its effects on immune checkpoints, epigenetic modifications, and protein interactions, thereby affecting the clinical outcomes of cancer patients and making it a potential target for cancer treatment.
Given that the most significant correlations were observed in LGG, further analysis was conducted to explore the relationship between SLC22A1 and LGG. COX analysis confirmed that SLC22A1 was an independent risk factor for LGG patients. Pathway enrichment analysis highlighted the potential impact of SLC22A1 on various biological processes and pathways related to immune response and tumor development in LGG cells. These included processes such as neutrophil degranulation, signaling by interleukins, interferon signaling, extracellular matrix organization, cell surface interaction at the vascular level, cytokine receptor interaction, cell cycle checkpoints, and regulation of innate immune response. The level of immune cell infiltration was found to significantly affect the OS of LGG patients. Collectively, these findings suggest that SLC22A1 may serve as a biomarker for poor prognosis in LGG patients, influencing their survival rates through the recruitment of immune cells in the TME and intracellular immune responses. This comprehensive analysis sheds light on the potential multifaceted role of SLC22A1 in cancer progression and provides valuable insights into its clinical implications.
Gliomas are often considered “cold phenotype” tumors from an immunity standpoint due to their close association with immune cells and factors in the TME. They can establish “immune privilege” through various mechanisms [36,37], rendering traditional immunotherapy less effective. The relationship between the unique microenvironment of gliomas and its anti-tumor immune activity is very complex. Immune cells function in a more complex way, not only related to immune factors, but also to other biological factors. SLC22A1, as a protein that maintains cellular cation exchange, has not been reported to be associated with tumor immunity. This article demonstrated from multiple perspectives that its high correlation with the formation of the tumor microenvironment in gliomas, suggesting that studying gliomas from a functional mechanism perspective may benefit more. Our research highlights SLC22A1 as a promising and effective biomarker and target for LGG, this result needs to be confirmed in the future. In vitro studies observing the cell viability of overexpressing or knockout cells can provide insights into their effects on immune cells, particularly macrophages, and their tumorigenicity in vivo. Clinically, evaluating the effectiveness of immunotherapy by detecting SLC22A1 levels or enhancing the treatment outcome for cancer patients by targeting SLC22A1 in combination with traditional immunotherapy represents potential avenues for exploration. In this paper, we demonstrated that downregulating the expression SLC22A1 in glioma U251 cells had a good anti-tumor effect. Furthermore, the risk score and survival model developed in this study were based on the mRNA levels of three immune checkpoints and the patient’s age. This approach circumvents the challenges posed by the complexity of brain structure and the diversity of glioma types in clinical practice, allowing for a more accurate assessment. Similar to established indicators like IDH mutation and 1p/19q deletion, which are independent of histology [38,39], the risk score and nomogram serve as new indicators that empower clinicians to comprehend the status of LGG patients and their survival rates. These indicators demonstrate strong practical application potential, with an area under the curve (AUC) exceeding 0.8. Although we have demonstrated that the decrease of SLC22A1 expression inhibited the growth of glioma U251 cells, the underlying mechanism was not yet clear, and more experiments were needed to prove its correlation with immune regulation.
SLC22A1 is a common cation transporter that participates in ion exchange of cells. This study identified SLC22A1 as an independent risk factor and potential prognostic biomarker for LGG. SLC22A1 emerges as a treatment target for LGG patients, with its negative impact on prognosis potentially associated with tumor immunity. Additionally, the established survival model for LGG patients exhibits robust predictive performance, opening avenues for improved clinical management and personalized treatment strategies.
Acknowledgement: None.
Funding Statement: This work was supported by National Natural Science Foundation of China (82160639), the Guizhou Provincial Science & Technology Program (QKHJC[2021]571), the Science & Technology Plan of Zunyi (ZSKHHZ[2020]87), the Xin Miao Foundation of Zunyi Medical University (QKPTRC[2019]-026), the PhD Start-Up Foundation of Zunyi Medical University (F-948).
Author Contributions: The authors confirm contribution to the paper as follows: study conception and design: Jing HUI, Qinghong KONG, Yun LIU; analysis and interpretation of results: Nana SUN, Yong LIU, Chunbo YU, Yong KE, Yong CAO, Anxiao YU; manuscript preparation: Jing HUI, Yun LIU. All authors reviewed the results and approved the final version of the manuscript.
Availability of Data and Materials: The datasets generated during and/or analyzed during the current study are available from the corresponding author on reasonable request.
Ethics Approval: Not applicable.
Conflicts of Interest: The authors declare that they have no conflicts of interest to report regarding the present study.
References
1. Lozano E, Briz O, Macias RIR, Serrano MA, Marin JJG, Herraez E. Genetic heterogeneity of SLC22 family of transporters in drug disposition. J Pers Med. 2018;8(2):14. [Google Scholar] [PubMed]
2. Furihata T, Anzai N. Functional expression of organic ion transporters in astrocytes and their potential as a drug target in the treatment of central nervous system diseases. Biol Pharm Bull. 2017;40(8):1153–60. [Google Scholar] [PubMed]
3. Yoshikawa T, Yanai K. Histamine clearance through polyspecific transporters in the brain. Handb Exp Pharmacol. 2017;241:173–87. [Google Scholar] [PubMed]
4. Chen P, Cao Y, Guo Y, Xu Q, Wang X, Zhang L, et al. Association of SLC22A1 rs622342 and ATM rs11212617 polymorphisms with metformin efficacy in patients with type 2 diabetes. Pharmacogenet Genomics. 2022;32(2):67–71. [Google Scholar] [PubMed]
5. Degaga A, Sirgu S, Huri HZ, Sim MS, Kebede T, Tegene B, et al. Association of Met420del variant of metformin transporter gene SLC22A1 with Metformin treatment response in ethiopian patients with type 2 diabetes. Diabetes Metab Syndr Obes. 2023;16:2523–35. [Google Scholar] [PubMed]
6. Mohammadi F, Rostami G, Assad D, Shafiei M, Hamid M, Jalaeikhoo H. Association of SLC22A1, SLCO1B3 drug transporter polymorphisms and smoking with disease risk and cytogenetic response to imatinib in patients with chronic myeloid leukemia. Lab Med. 2021;52(6):584–96. [Google Scholar] [PubMed]
7. Herraez E, Lozano E, Macias RI, Vaquero J, Bujanda L, Banales JM, et al. Expression of SLC22A1 variants may affect the response of hepatocellular carcinoma and cholangiocarcinoma to sorafenib. Hepatology. 2013;58(3):1065–73. [Google Scholar] [PubMed]
8. Sreenivasan Tantuan S, Viljoen CD. Imatinib affects the expression of SLC22A1 in a non-linear concentration-dependent manner within 24 hours. Med Sci Monit Basic Res. 2018;24:59–62. [Google Scholar] [PubMed]
9. Cahua-Pablo J, Gómez-Zamudio JH, Reséndiz-Abarca CA, Tello-Flores VA, Eulogio-Metodio Y, Ramírez-Vargas MA, et al. Genetic variants in SLC22A1 are related to serum lipid levels in Mexican women. Lipids. 2022;57(2):105–14. [Google Scholar] [PubMed]
10. Ningrum VDA, Sadewa AH, Ikawati Z, Yuliwulandari R, Ikhsan MR, Fajriyah R. The influence of metformin transporter gene SLC22A1 and SLC47A1 variants on steady-state pharmacokinetics and glycemic response. PLoS One. 2022;17(7):e0271410. [Google Scholar] [PubMed]
11. Al-Eitan LN, Almomani BA, Nassar AM, Elsaqa BZ, Saadeh NA. Metformin pharmacogenetics: effects of SLC22A1, SLC22A2, and SLC22A3 polymorphisms on glycemic control and HbA1c levels. J Pers Med. 2019;9(1):17. [Google Scholar] [PubMed]
12. Jia Y, Liu L, Shan B. Future of immune checkpoint inhibitors: focus on tumor immune microenvironment. Ann Transl Med. 2020;8(17):1095. [Google Scholar] [PubMed]
13. Hernández-Camarero P, López-Ruiz E, Marchal JA, Perán M. Cancer: a mirrored room between tumor bulk and tumor microenvironment. J Exp Clin Cancer Res. 2021;40(1):217. [Google Scholar]
14. Wan M, Ding Y, Li Z, Wang X, Xu M. Metabolic manipulation of the tumour immune microenvironment. Immunol. 2022;165(3):290–300. [Google Scholar]
15. Liao X, Li G, Cai R, Chen R. A review of emerging biomarkers for immune checkpoint inhibitors in tumors of the gastrointestinal tract. Med Sci Monit. 2022;28:e935348. [Google Scholar] [PubMed]
16. Lupu J, Hamann P, Routier É., Robert C. Treatment of melanoma with immune checkpoints inhibitors. Rev Prat. 2021;71(4):380–3. [Google Scholar] [PubMed]
17. Grobet-Jeandin É., Pinar U, Loriot Y, Roupret M. Treatment of bladder cancer with immune checkpoints inhibitors. Rev Prat. 2021;71(4):391–5. [Google Scholar] [PubMed]
18. Venkatachalam S, McFarland TR, Agarwal N, Swami U. Immune checkpoint inhibitors in prostate cancer. Cancers. 2021;13(9):2187. [Google Scholar] [PubMed]
19. Mahdy AMS. Stability, existence, and uniqueness for solving fractional glioblastoma multiforme using a Caputo-Fabrizio derivative. Math Meth Appl Sci. 2023;42(3):1–18. [Google Scholar]
20. Mahdy AMS, Mohamed MS, Lotfy K, Alhazmi M, El-Bary AA, Raddadi MH. Numerical solution and dynamical behaviors for solving fractional nonlinear Rubella ailment disease model. Results Phys. 2021;24(2):104091. [Google Scholar]
21. Mahdy AMS, Higazy M, Mohamed MS. Optimal and memristor-based control of a nonlinear fractional tumor-immune model. Comput Mater Contin. 2021;67(3):3463–86. [Google Scholar]
22. Haddad AF, Young JS, Oh JY, Okada H, Aghi MK. The immunology of low-grade gliomas. Neurosurg Focus. 2022;52(2):E2. [Google Scholar] [PubMed]
23. Ryan M, Wong WC, Brown R, Akbani R, Su X, Broom B, et al. TCGASpliceSeq a compendium of alternative mRNA splicing in cancer. Nucleic Acids Res. 2016;44(D1):D1018–22. [Google Scholar] [PubMed]
24. Zhao Z, Zhang KN, Wang Q, Li G, Zeng F, Zhang Y, et al. Chinese glioma genome atlas (CGGAa comprehensive resource with functional genomic data from chinese glioma patients. Genom Proteomics Bioinform. 2021;19(1):1–12. [Google Scholar]
25. Ceccarelli M, Barthel FP, Malta TM, Sabedot TS, Salama SR, Murray BA, et al. Molecular profiling reveals biologically discrete subsets and pathways of progression in diffuse glioma. Cell. 2016;164(3):550–63. [Google Scholar] [PubMed]
26. Liu J, Lichtenberg T, Hoadley KA, Poisson LM, Lazar AJ, Cherniack AD, et al. An integrated TCGA pan-cancer clinical data resource to drive high-quality survival outcome analytics. Cell. 2018;173(2):400–16. [Google Scholar] [PubMed]
27. Li T, Fan J, Wang B, Traugh N, Chen Q, Liu JS, et al. TIMER: a web server for comprehensive analysis of tumor-infiltrating immune cells. Cancer Res. 2017;77(21):e108–10. [Google Scholar] [PubMed]
28. Li T, Fu J, Zeng Z, Cohen D, Li J, Chen Q, et al. TIMER2.0 for analysis of tumor-infiltrating immune cells. Nucleic Acids Res. 2020;48(W1):W509–14. [Google Scholar] [PubMed]
29. Li B, Severson E, Pignon JC, Zhao H, Li T, Novak J, et al. Comprehensive analyses of tumor immunity: implications for cancer immunotherapy. Genome Biol. 2016;17(1):174. [Google Scholar] [PubMed]
30. Yu G, Wang LG, Han Y, He QY. clusterProfiler: an R package for comparing biological themes among gene clusters. OMICS. 2012;16(5):284–7. [Google Scholar] [PubMed]
31. Reinhold WC, Sunshine M, Liu H, Varma S, Kohn KW, Morris J, et al. CellMiner: a web-based suite of genomic and pharmacologic tools to explore transcript and drug patterns in the NCI-60 cell line set. Cancer Res. 2012;72(14):3499–511. [Google Scholar] [PubMed]
32. Jia X, Yan B, Tian X, Liu Q, Jin J, Shi J, et al. CD47/SIRPα pathway mediates cancer immune escape and immunotherapy. Int J Biol Sci. 2021;17(13):3281–7. [Google Scholar] [PubMed]
33. Zhang Y, Zheng J. Functions of immune checkpoint molecules beyond immune evasion. Adv Exp Med Biol. 2020;1248:201–26. [Google Scholar] [PubMed]
34. Jiang N, Yu Y, Wu D, Wang S, Fang Y, Miao H, et al. HLA and tumour immunology: immune escape, immunotherapy and immune-related adverse events. J Cancer Res Clin Oncol. 2023;149(2):737–47. [Google Scholar] [PubMed]
35. Hu FF, Liu CJ, Liu LL, Zhang Q, Guo AY. Expression profile of immune checkpoint genes and their roles in predicting immunotherapy response. Brief Bioinform. 2021;22(3):bbaa176. [Google Scholar] [PubMed]
36. Abels ER, Broekman MLD, Breakefield XO, Maas SLN. Glioma EVs contribute to immune privilege in the brain. Trends Cancer. 2019;5(7):393–6. [Google Scholar] [PubMed]
37. Qi Y, Liu B, Sun Q, Xiong X, Chen Q. Immune checkpoint targeted therapy in glioma: status and hopes. Front Immunol. 2020;11:578877. [Google Scholar] [PubMed]
38. Spallone A. Editorial: modern neurosurgical management of gliomas, including local therapies. Front Oncol. 2023;13:1217180. doi:10.3389/fonc.2023.1217180. [Google Scholar] [CrossRef]
39. Norman S, Juthani RG, Magge R. Foundations of the diagnosis and management of low-grade gliomas. World Neurosurg. 2022;166:306–12. [Google Scholar] [PubMed]
Cite This Article
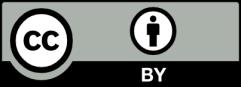
This work is licensed under a Creative Commons Attribution 4.0 International License , which permits unrestricted use, distribution, and reproduction in any medium, provided the original work is properly cited.