Open Access
ARTICLE
Mitochondrial autophagy gene signature predicts prognosis and response to immunity in esophageal cancer
1 Qilu Hospital, Shandong University, Jinan, 250012, China
2 Department of Biochemistry and Molecular Biology, School of Basic Medical Sciences, Cheeloo College of Medicine, Shandong University, Jinan, 250012, China
* Corresponding Author: JIANBO WANG. Email:
BIOCELL 2024, 48(2), 271-281. https://doi.org/10.32604/biocell.2023.029094
Received 01 February 2023; Accepted 24 May 2023; Issue published 23 February 2024
Abstract
Background: Esophageal cancer (ESCA) is a common digestive tract tumor. As a result, optimization of the early diagnosis of ESCA and identifying the contributing prognostic genes is urgently required. Herein, the prognosis of mitochondrial autophagy-related genes was analyzed in different subtypes of ESCA, and prognostic models were constructed to identify the immune cell infiltration with significant differences between subtypes. Methods: The Cancer Genome Atlas database was searched to download 185 ESCA samples, covering gene expression level data and clinical follow-up data, and 179 samples from the Gene Expression Omnibus database for subsequent validation analysis. The consensus Cluster Plus analysis method was employed to identify the best mitochondrial autophagy subtype. Kaplan-Meier curve was used to evaluate the correlation of survival prognosis between different subtype groups and actual survival prognostic information. A chi-square test was performed to analyze the correlation between subtypes and clinical information. Differential genetic analysis between different subtypes was performed using Limma packs (threshold setting: adj. p < 0.05&|log2FC|>1). Univariate Cox regression analysis was applied to identifying genes with significant prognosis, and the LASSO algorithm screened out key genes. The risk scores were constructed by Stepwise Cox regression analysis and divided into high and low-risk groups. Independent prognostic factors were determined using the univariate and nomograms constructed by multivariate Cox analysis. The CIBERSORT method was used to calculate the composition ratio of 22 immune cells; the matrix and immune scores of tumor samples were calculated by the ESTIMATE algorithm. Wilcoxon’s test was performed to compare the expression differences of immune checkpoint genes and human leukocyte antigen family genes between high- and low-risk groups and the difference in IC50 between these risk groups of 138 chemotherapy drugs. Relationships between mitochondrial autophagy subtypes and high- and low-risk groups were assessed using the ggalluvial package in R3.6.1. Results: Seven mitochondrial autophagy genes associated with the of ESCA were identified (PTPN4, ALKBH4, IL6, FN3KRP, HSDL1, B3GNT2, CCT4). High and low risk were significantly correlated with the actual prognosis. Nomograms constructed by factors stage and Risk group showed significant relation with patient prognosis. Eleven immune cells significantly differing in the two subtypes were identified, followed by ten significantly different immune checkpoint genes. Conclusion: Seven mitochondrial autophagy genes associated with the prognosis of ESCA may serve as the key prognostic genes and novel therapeutic targets for esophageal cancer.Keywords
Esophageal Cancer (ESCA), as one of the most prevalent malignant tumors globally, results in a serious threat to human health and life, which lies the seventh most common cancer worldwide and the sixth leading cause of cancer death [1]. The poor prognosis of this tumor poses a severe risk to human health. For over half a century, despite the increasing advances in research on the diagnosis, treatment, and pathogenesis of ESCA, with a five-year survival rate of less than 5% of metastatic ESCA [2], the pathogenesis of ESCA remains unelucidated, in which the morbidity and mortality may be related to various factors. Even after an aggressive surgery-based comprehensive treatment, tumor recurrence and distant metastasis are the remaining most contributing causes of death in patients with ESCA [3]. Targeted immunotherapy has been demonstrated to exhibit feasibility in recent years. Whether the mitochondrial autophagy-related genes can serve as a new target for targeted immunotherapy in patients with advanced ESCA should be explored [4].
As the key organelles for intracellular energy production, mitochondria are essential to maintain tissue homeostasis and the channels for programmed apoptosis and necrotic cell death [5], which requires autophagy, an intracellular degradation process. Generally, autophagy could maintain homeostasis by eliminating old proteins and damaged organelles [6]. In tumor cells, autophagy can inhibit tumorigenesis by damaging tumor cell survival to induce cell death and promote tumorigenesis by activating cancer cell proliferation and tumor growth, displaying a dual role [7]. Mitophagy refers to the targeted phagocytosis and destruction of mitochondria by the autophagy device, which could selectively remove excess or damaged mitochondria, playing a significant role in manipulating the number of mitochondria in cells and maintaining the function of mitochondria, also exerting prominent effects in neurodegenerative diseases, cardiovascular disease, and cancer [8,9]. As a result, identifying the mitochondrial autophagy genes with potential association with ESCA could contribute a lot to the prognosis and treatment.
In the present study, the mitochondrial metabolism-related genes were investigated. A comprehensive bioinformatics analysis covered the gene expression obtained from The Cancer Genome Atlas (TCGA), mitochondrial autophagy gene isoforms, and clinical data. By identifying the mitochondrial autophagy-associated genes in ESCA, a risk-scoring system was constructed for ESCA and validated in TCGA and Gene Expression Omnibus (GEO) datasets. Our findings suggest the validity of characteristics in seven genes as independent predictors of OS in ESCA patients. This study provided reliable indicators for predicting tumor immune status and reflecting chemotherapy response in ESCA patients, contributing to formulating more personalized and precise treatment strategies.
Data retrieval and preprocessing
ESCA gene expression level data (all normalized log2 (FPKM+1) expression level data) and clinical follow-up data were downloaded from the TCGA database (https://gdc-portal.nci.nih.gov/). After excluding the missing survival time and survival time 0 samples, 185 tumor samples were eventually retained.
Meanwhile, the GSE53625 [10] ESCA dataset with the sequencing platform GPL18109 was downloaded from the National Center for Biotechnology Information GEO [11] (https://www.ncbi.nlm.nih.gov/) database and finally involved 179 samples for subsequent validation analysis.
Identification of differentially expressed mitochondrial autophagy (mitophagy) genes and correlation analysis
Twenty-nine mitochondrial autophagy-associated genes were downloaded from the Pathway Unification database (https://pathcards.genecards.org/), which were then observed separately for differences between ESCA vs. normal and to analyze the direct Pearson correlations for differential mitochondrial autophagy genes.
Identification of mitochondrial autophagic subtypes in esophageal cancer
Based on the differential mitochondrial autophagy genes acquired above, the samples were subjected to tumor subtype analysis by unsupervised hierarchical clustering R 3.6.1 Consensus Cluster Plus (http://www.bioconductor.org/packages/release/bioc/html/ConsensusClusterPlus.html) Version 1.54.0 [12] to obtain the best mitochondrial autophagy subtypes (K values), where the range of K values was set from 2 to 6.
Prognostic and clinical correlation analysis of subtypes
The Kaplan-Meier (KM) curve method in the survival package Version 2.41-1 [13] (http://bioconductor.org/packages/survivalr/) in R3.6.1 language was employed to assess the prognostic correlation of survival between different subtype sample groups. Correlations between subtypes and clinical information (age, gender, stage, etc.) were analyzed by integrating data on clinical data of ESCA by performing the chi-square test.
Identification of inter-subtype specific gene
To observe the possible molecular mechanisms between different mitochondrial autophagy subtypes, the Limma package [14] Version 3.10.3 (http://www.bioconductor.org/packages/2.9/bioc/html/limma.html) was employed to provide the corresponding p-values and log2FC of the genes. I Benjamini & Hochberg method was employed for multiple testing corrections to acquire the corrected p-values, i.e., adj. Value at two differential diversity and significance levels was evaluated, and the differential expression threshold was set as adj. p-value < 0.05&|log2FC|>1. The genes specifically expressed between subtypes were identified as differential.
Identification of prognostic gene
Based on the specific genes’ expression levels, one-way Cox regression analysis in the survival package Version 2.41-1 [13] (http://bioconductor.org/packages/survivalr/) in the R3.6.1 language was employed to screen significant correlations using a p-value less than 0.05 as the threshold. Specific genes significantly associated with survival prognosis at the expression level were enrolled for the subsequent analysis.
Construction and performance validation of prognostic models (risk scores)
Based on the prognosis-related genes retained, survival regression analysis with the LASSO algorithm in the lars package (https://cran.r-project.org/web/packages/lars/index.html) Version 1.2 in the R3.6.1 language was performed to screen out the key genes. Afterward, the Risk score was prepared by performing a stepwise Cox regression analysis using the survminer package (https://cran.r-project.org/web/packages/survival/index.html) Version 0.4.9 in R3.6.1 language. The stepwise Cox regression analysis was employed to construct the Risk score formula by regression coefficients of individual genes and model gene expression levels, as follows:
Risk score = h0(t) * exp (β1X1 + β2X2 + ... + βnXn)
According to the Risk score formula, the Risk score values were calculated for each sample in the TCGA training and GEO validation sets. Then the samples were classified into High (Risk score higher than the median Risk score) and Low (Risk score lower than or equal to the median Risk score) samples, respectively, based on the median Risk score value as the dividing line. Risk score median value) sample groups and the association between the status of the High and Low groups and the actual survival prognosis information was assessed by the KM curve method of the survivor package Version 2.41-1 [13] in the R3.6.1 language.
Prognostic independence analysis and evaluation of the nomogram
The correlation between RiskGroup and clinical information (age, gender, stage, etc.) was evaluated by integrating data on clinical information of ESCA. To further investigate the prognostic independence between clinical prognostic factors and RiskGroup, clinical factors and RiskGroup in TCGA data in univariate and multifactor Cox regression were enrolled to screen out the independent prognostic factors with a p-value < 0.05 as the threshold and drew forest plots.
Based on the independent prognostic factors above, the column line graph (Nomogram) was constructed using the rms package Version 5.1-2 (https://cran.r-project.org/web/packages/rms/index.html) [15] in the R3.6.1 language.
Comparison of immune microenvironment between subtypes
The immune microenvironment status of ESCA samples was assessed following two algorithms. The Wilcoxon test examined the differences in their infiltration among different subtypes.
CIBERSORT (https://cibersortx.stanford.edu/index.php [16]) was employed to calculate 22 immune cell composition ratios based on the expression levels of ESCA samples.
The ESTIMATE algorithm [17] was employed to estimate the stromal scores and immune scores of tumor samples based on expression data to represent the presence of stromal and immune cells.
Comparison of immune checkpoints and human leukocyte antigen (HLA) family gene differences between risk groups
Immune checkpoint genes (e.g., PD1 (PDCD1), PD-L1 (CD274); CTLA-4 (CTLA4); CD278 (ICOS); TIM3 (HAVCR2); LAG3; CD47; BTLA; TIGIT; MYD1 (SIRPA); OX40 (TNFRSF4)) and HLA family genes were extracted from ESCA expression data, 4-1BB (TNFRSF9); B7-H4 (VTCN1)) and HLA family gene expression data. The Wilcoxon test was performed to compare the expression differences of immune checkpoint genes and HLA family genes between high and low-risk groups.
The sensitivity of each patient to chemotherapeutic agents was estimated by employing the Genomics of Drug Sensitivity in Cancer (GDSC; https://www.cancerrxgene.org/) database. The half-maximal inhibitory concentration (IC50) was quantified using the pRRophetic package (https://github.com/paulgeeleher/pRRophetic) [18] in R. The Wilcoxon test was performed to compare the differences in IC50 between RiskGroup for 138 chemotherapeutic agents.
Association between risk group and subtypes
Combining the subtype grouping (Cluster) and high and low-risk grouping (RiskGroup) of the samples, we drew the Sankey diagram using the ggalluvial package (version 0.12.3, https://CRAN.R-project.org/package=ggalluvial) [19] in the R3.6.1 language to observe the relationship between mitochondrial autophagic subtypes and high and low-risk groups.
Expression pattern analysis of mitochondrial autophagy genes
A set of 29 recognized mitochondrial autophagy-associated genes were analyzed to identify different mitochondrial autophagy-associated gene expression patterns (Fig. 1A), where 14 mitochondrial autophagy-related genes significantly differed between ESCA and normal samples (p < 0.05). The correlation pattern between 14 differential mitochondrial autophagy-related genes was analyzed to explore the association between the differential mitochondrial autophagy-related genes (Fig. 1B).
Figure 1: Mitochondrial autophagy gene expression patterns and identification of mitochondrial autophagy subtypes in esophageal cancer (ESCA). (A) Transcriptome expression box plot of 29 mitochondrial autophagy-related genes between healthy and ESCA samples. *p < 0.05, **p < 0.01, ****p < 0.0001, (B) Expression level correlation heatmap of 14 differential mitochondrial autophagy-related genes. Here, X stands for no biological significance, (C) Consensus clustering cumulative distribution function (CDF) for k = 2–6, (D) Consensus clustering matrix for optimal k = 2, (E) The corresponding relative change in area under the cumulative distribution function (CDF) curves when the clustering number changes from k to k + 1; the range of k changed from 2 to 6; and the optimal k = 2.
Identification of mitochondrial autophagic subtypes in esophageal cancer
Based on the 14 identified differential mitophagy-related genes, a sample unsupervised clustering analysis was performed on ESCA tumor samples (Fig. 1C). The best k = 2 was selected by setting the range of k values from 2 to 6 (Fig. 1D), and two different subtypes, C1 and C2, were obtained, covering 101 and 84 ESCA samples, respectively. The stability of the clustering results was further verified by PAC, and the minimum value of PAC, which was also the optimal k remaining 2, as shown in Fig. 1E.
Analysis of survival and clinical correlation of subtypes
The survival KM curve method in R3.6.1 language was employed to assess the survival prognostic correlation between different subtypes (Fig. 2A), revealing the significantly different survival prognostic information between subtypes, with a poorer prognosis in C1. The distribution of 14 differential mitochondrial autophagy gene expression levels in the subtype samples was depicted in a heat map (Fig. 2B). The clinical information of the samples for all ESCA samples (age, gender, stage, etc.) was collected to analyze the correlation between subtypes and clinical factors, and the cardinality test results are shown in Fig. 2C, which revealed Pathologic_M (Pathological metastasis), Pathologic_N (Pathological node), and Pathologic_Stage as the significantly different subtypes.
Figure 2: Survival and clinical relevance of subtypes. (A) Kaplan Meier survival curves for different subtypes, (B) Clinically relevant heatmap of 29 mitochondrial autophagy gene expression. Red, high expression; blue, low expression, (C) Distribution of clinical features under different subtypes.
Differential gene identification between subtypes and identification of prognostically significantly associated genes
Differential genes between the two subtypes were analyzed, identifying 914 differentially expressed genes (Figs. 3A and 3B). One-way Cox regression analysis in the R3.6.1 language survival package was performed to screen out 92 genes with significantly associated prognoses (p < 0.05).
Figure 3: Differential gene identification between subtypes. (A) Differential gene volcano map, blue, downregulated genes; red, upregulated genes, (B) Differential gene expression heatmap (top 100, 50 genes up and down).
Prognostic model construction and performance validation
Based on the expression data of 92 prognostic genes, the LASSO algorithm was employed to screen out the optimized gene results (Fig. 4A), which identified 36 key genes (Fig. 4B). Afterwards, the optimal gene combinations were screened out by the stepwise Cox regression algorithm, to eventually identify seven model genes (Fig. 4C). Subsequently, we constructed a Risk score model using the regression coefficients of the seven model genes and their expression levels in the TCGA training dataset.
Figure 4: Prognostic model construction and performance validation. (A) The distribution of the least absolute shrinkage and selection operator (LASSO) coefficient, (B) Likelihood bias of the LASSO coefficient distribution, with two vertical dashed lines representing lambda.min (red line on the left) and lambda.1se (black line on the right), (C) Multivariate Cox regression forest plot for seven model genes, (D) Risk score distribution (top) and time-to-live state (bottom) in TCGA training set, (E) Kaplan-Meier (KM) curve plot related to prognosis based on Riskscore prediction model, (F) 1-, 3-, and 5-year receiver operating characteristic (ROC) curves based on prognostic genetic characteristics, (G) Risks core distribution (top) and time-to-live state (bottom) in Gene Expression Omnibus validation set, (H) KM curve related to prognosis based on Risk score prediction model, (I) ROC curves based on prognostic genetic characteristics at 1, 3 and 5 years.
The Risk score for each patient was calculated, based on which the samples in the TCGA training set and GEO validation set were divided into High (Risk score above the median Risk score value) and Low (Risk score equal to below the median Risk score value) sample groups. The distribution of Risks core values and survival time distribution are depicted in Fig. 4D. The KM curve method of the survivor package in R3.6.1 language was employed to assess the correlation between the grouping status of High and Low and the actual patient prognostic information; Fig. 4E described the KM curves for each data set. The ROC curves at 1, 3, and 5 years based on genetic prognostic features are depicted in Fig. 4F. Moreover, in the validation set, Kaplan–Meier analysis demonstrated a significant variation in patient survival status between the studied groups (Figs. 4G and 4H). The ROC curve demonstrated that the risk score had a good performance in predicting the patient’s survival status at 1-, 3-, and 5-year; the AUC measures were 0.718, 0.658, and 0.669, respectively (Fig. 4I). A significant correlation was revealed between the different risk groups and the actual prognosis obtained from the classification of the samples based on the prediction of the Risk score model in the TCGA training set and the GEO validation set.
Prognostic independence analysis and the nomogram
For all ESCA samples in TCGA, we collected the clinical data from the samples, and one-way Cox regression analysis was performed on the clinical factors and Risk Group of the samples using the R3.6.1 language survival package, where the factors with p < 0.05 were selected for the further multifactor Cox regression to identify the significant independent prognostic factors (Figs. 5A and 5B).
Figure 5: Prognostic independence analysis and nomogram survival analysis. (A) Clinical information univariate, (B) Multivariate Cox regression forest plot, (C) Independent prognostic factor survival prediction model nomogram plot, (D) Kaplan-Meier (KM)-related curve based on nomogram prediction model and prognosis, (E) Nomogram for 1-, 3-, and 5-year survival rate prediction and actual survival rate consistency line chart. The horizontal axis represents the predicted survival rate, and the vertical axis represents the actual survival rate, (F) Nomogram plots for 1-, 3-, and 5-year receiver operating characteristic (ROC) curves.
To further assess the correlation between the prognostic factors stage and Risk group and survival prognosis (Fig. 5C), the Stage and Risk Group were involved in the construction of the column line graph survival model. The clinical indicators through the “Total points” axis were combined in the first row with a C-index = 0.715, and the column line graph could predict the survival of the sample. The consistency of the predicted 1-, 3-, and 5-year survival rates with the actual 1-, 3-, and 5-year survival rates of the column line graph survival model was compared and validated (Fig. 5D). A significant association of nomogram with patient prognosis was revealed (Fig. 5E), as depicted in Fig. 5F.
Comparison of immune microenvironment among mitochondrial autophagic subtypes
The expression profile data of ESCA samples were employed to calculate the immune cell types using the CIBERSORT algorithm, which revealed 22 immune cell type proportions. Then the various immune cell proportions among different subtypes were compared, and 11 significantly different immune cells (DICs) were identified (Fig. 6A). The estimate algorithm was utilized to calculate the immune score and stromal score. Then the differences in the infiltration levels of the immune score and stromal score between different subtypes were analyzed (Fig. 6B).
Figure 6: Immune microenvironment among mitochondrial autophagic subtypes, inter-risk group immune checkpoint and human leukocyte antigen (HLA) family gene. (A) Comparison plot of immune cell types with significant differences between different subtypes in the CIBERSORT algorithm, (B) Immunological score and matrix score vs. violin plot in different subtypes, (C) Comparison chart of immune checkpoint genes with significant differences between different risk groups, (D) HLA family gene comparison chart with significant differences between different risk groups. *p < 0.05, **p < 0.01, ***p < 0.001, ****p < 0.0001.
Inter-risk group immune checkpoint and human leukocyte antigen family gene analysis
The expression data of immune checkpoint genes were extracted from TCGA. By comparing the differences in immune checkpoint gene expression between subtypes, 10 significantly different immune checkpoint genes were identified (Fig. 6C). The Wilcoxon test was performed to compare the differences in the expression of HLA family genes among subtypes (Fig. 6D).
The Genomics of Drug Sensitivity in Cancer (GDSC) database was employed to estimate the sensitivity of each patient to chemotherapeutic drugs, with the IC50 quantified by the pRRophetic package in R. The differences in IC50 levels of 138 chemotherapeutic drugs were compared. The results are depicted in Fig. 7 (demonstrating the four significantly common chemotherapeutic agents).
Figure 7: Drug sensitivity analysis. (A–D) Comparison of IC50 levels of four chemotherapy drugs in different risk groups.
The association analysis between TEX-subtype and high- and low-risk groups
The relationship between mitochondrial autophagy subtypes (Cluster) grouping and high and low-risk groups (Risk Group) was assessed, with the results of the chi-square test shown in Fig. 8, where C1 subtypes account for the most in the high-risk group.
Figure 8: The distribution between cluster and risk groups.
Mitochondria are recognized as the “cellular energy factories” that convert energy produced by the oxidation of organic matter into ATP [20], also as the main source of reactive oxygen species (ROS). Studies have pointed out changes in gene expression as a result of excess ROS, causing damage to proteins and lipids, which then promotes the development of cancer [21]. Mitochondrial DNA (mtDNA) is susceptible to damage from mitochondrial reactive oxygen species (mtROS) [22]. Autophagy refers to a testament depending on lysosomes to degrade its own cytoplasmic proteins and damaged organelles in mammals [23], whereas mitophagy is a process to selectively clear the damaged mitochondria, mainly mediated by PINK1-Parkin, which serves as a mitochondrial quality control mechanism [23]. Autophagy also plays a prominent role in developing ESCA by clearing damaged mitochondria to relieve oxidative stress within cells. Predicting the mitochondrial autophagy genes associated with ESCA contributes to the prognosis and treatment of ESCA patients.
Through bioinformatic analyses, we analyzed 29 mitochondrial autophagy-related genes, and significant differences were found in 14 of these genes between ESCA and normal samples (p < 0.05). Accordingly, we performed the sample unsupervised clustering analysis on ESCA tumor samples, obtaining two different subtypes C1 and C2 [24,25]. The prognostic survival correlation among subtypes was assessed by the KM survival curve, with poor prognosis of C1 in tumor, M, and N stages [26]. We further obtained 914 differentially expressed genes using the differential gene analysis on the two subtypes [27], and 92 prognostically significant genes were screened out using the univariate Cox regression analysis [28]. Subsequently, 36 key genes were obtained from the optimized gene results using the LASSO algorithm, then seven model genes (PTPN4, ALKBH4, IL6, FH3KRP, HSDL1, B3GNT2, and CCT4) were obtained using the stepwise Cox regression algorithm. Of these model genes, members of the PTPN family are mostly associated with gastrointestinal cancers. Studies have demonstrated the highly expressed PTPN1, PTPN4, and PTPN12 in ESCA cell lines [29]. B3GNT2 encodes polyN-acetolactosamine synthase, which reduces T cell activation and disrupts tumor-T cell interactions [30]. The dysregulation of the CCT4 gene leads to inhibited migration and invasion capacity of ESCC cells [31]. We then constructed a risk-scoring model using the seven model genes based on the regression coefficients and expression levels [32], which were also validated in the TCGA training and GEO validation sets. The clinical information of TCGA ESCA samples was collected to perform the univariate Cox regression analysis; factors with p < 0.05 were selected for multivariate Cox regression analysis, and the significant independent prognostic factors were identified [33]. Prognostic significant correlator Stage and risk groups were used to construct the survival model, and the nomogram showed a significant correlation with the patient’s prognosis, using which the 1-, 3-, and 5-year ROC curves were constructed [34,35]. Nomograms can serve to diagnose or predict the onset or progression of a disease in combination with multiple indicators, exerting a superior performance to TNM staging systems for multiple cancers, and have been proposed as alternatives or even as new criteria [36].
In the tumor immune microenvironment, a large number of immune cells accumulate in and around the tumor, where these immune cells are inextricably linked to each other, tumor cells, and immune cells. The so-called immune microenvironment or immune infiltration analysis is essential for calculating the composition ratio of immune cells in tumor tissues [37]. We compared 22 intertype immune cells and obtained 11 significantly different immune cells. Studies have shown the anti-tumor immunity of TFH in a CD8+-dependent manner [38]. Based on the differences in immune checkpoint gene expression between subtypes, 10 checkpoint genes were obtained (p < 0.001). We also compared the differences in HLA family gene expression between subtypes. The tumor immune microenvironment can influence the response of the body to immune checkpoint inhibitors (ICIs) [39]. For example, patients with ESCA are associated with a poor prognosis after conventional therapy, for whom chemotherapy combined with ICIs to treat the anti-tumor immune response could serve as a new treatment strategy [40]. As a result, ICIs can be treated as “broad-spectrum antineoplastic agents” with clinical efficacy in a wide range of cancers [41]. One study noted the optimized patient response rates resulting from combining CTLA-4 and PD-1 blockers [42,43]. Further research is required in combination with these ICIs for ESCA [44]. We compared the differences in IC50 levels of 138 chemotherapy drugs in different risk groups, revealing higher sensitivity with the commonly used chemotherapy drugs cisplatin and docetaxel in the low-risk group. The relationship between the mitochondrial autophagy subtype grouping and the high- and low-risk groups were analyzed; the chi-square test indicated the involvement of mainly the C1 subtype in the high-risk group.
In summary, the present study identified seven mitochondrial autophagy-related characteristic genes and established a risk model and nomogram with accurate predictive capability on the prognosis of ESCA patients. These results provide promising therapeutic targets for the treatment of patients with ESCA. Up to now, substances that can induce mitochondrial autophagy response under pathological conditions, molecules that specifically mediate the activation of the mitochondrial autophagy pathway, and the role they play in the occurrence and development of ESCA are remaining issues that require further studies. The limitations of this study refer to the involvement of genes restricted to those being important for building prognostic models identified by laminar screening, while other excluded genes may also have an impact on ESCA. Second, as a public data mining-based study, our results should be validated in prospective multicenter clinical trials.
Acknowledgement: All authors are grateful to each participant involved in this study.
Funding Statement: This research was not supported by any foundation.
Author Contributions: Daixin Zhao designed the study, performed experiments, analyzed data, wrote the manuscript, and edited the figures. Qingyu Wang and Jianbo Wang reviewed the manuscript, provided funding, and revised the manuscript.
Availability of Data and Materials: The data used to support the findings of this study are presented in this article. Further inquiries can be directed to the corresponding author.
Ethics Approval: Not applicable.
Conflicts of Interest: All authors state no conflict of interest.
References
1. Yamamoto S, Kato K. Immuno-oncology for esophageal cancer. Future Oncol. 2020;16:2673–81. [Google Scholar] [PubMed]
2. Ghazy HF, El-Hadaad HA, Wahba HA, Abbas R, Abbas OA. Metastatic esophageal carcinoma: prognostic factors and survival. J Gastrointest Cancer. 2022;53(2):446–50. [Google Scholar] [PubMed]
3. Huang FL, Yu SJ. Esophageal cancer: risk factors, genetic association, and treatment. Asian J Surg. 2018;41(3):210–5. [Google Scholar] [PubMed]
4. Park R, Williamson S, Kasi A, Saeed A. Immune therapeutics in the treatment of advanced gastric and esophageal cancer. Anticancer Res. 2018;38(10):5569–80. [Google Scholar] [PubMed]
5. Abate M, Festa A, Falco M, Lombardi A, Luce A, Grimaldi A, et al. Mitochondria as playmakers of apoptosis, autophagy and senescence. Semin Cell Dev Biol. 2020;98:139–53. [Google Scholar] [PubMed]
6. Li W, He P, Huang Y, Li YF, Lu J, Li M, et al. Selective autophagy of intracellular organelles: recent research advances. Theranostics. 2021;11(1):222–56. [Google Scholar] [PubMed]
7. Li X, He S, Ma B. Autophagy and autophagy-related proteins in cancer. Mol Cancer. 2020;19(1):12. [Google Scholar] [PubMed]
8. Bravo-San Pedro JM, Kroemer G, Galluzzi L. Autophagy and mitophagy in cardiovascular disease. Circ Res. 2017;120(11):1812–24. [Google Scholar] [PubMed]
9. Cai Q, Jeong YY. Mitophagy in Alzheimer’s disease and other age-related neurodegenerative diseases. Cells. 2020;9(1):150. [Google Scholar] [PubMed]
10. Li Y, Lu Z, Che Y, Wang J, Sun S, Huang J, et al. Immune signature profiling identified predictive and prognostic factors for esophageal squamous cell carcinoma. Oncoimmunol. 2017;6(11):e1356147. [Google Scholar]
11. Barrett T, Wilhite SE, Ledoux P, Evangelista C, Kim IF, Tomashevsky M, et al. NCBI GEO: archive for functional genomics data sets—update. Nucleic Acids Res. 2013;41(D1):D991–5. [Google Scholar] [PubMed]
12. Wilkerson MD, Hayes DN. ConsensusClusterPlus: a class discovery tool with confidence assessments and item tracking. Bioinformatics. 2010;26:1572–3. [Google Scholar] [PubMed]
13. Rizvi AA, Karaesmen E, Morgan M, Preus L, Wang J, Sovic M, et al. gwasurvivr: an R package for genome-wide survival analysis. Bioinformatics. 2019;35(11):1968–70. [Google Scholar] [PubMed]
14. Ritchie ME, Phipson B, Wu D, Hu Y, Law CW, Shi W, et al. Limma powers differential expression analyses for RNA-sequencing and microarray studies. Nucleic Acids Res. 2015;43(7):e47. [Google Scholar] [PubMed]
15. Zhang S, Tong YX, Zhang XH, Zhang YJ, Xu XS, Xiao AT, et al. A novel and validated nomogram to predict overall survival for gastric neuroendocrine neoplasms. J Cancer. 2019;10(24):5944–54. [Google Scholar] [PubMed]
16. Chen B, Khodadoust MS, Liu CL, Newman AM, Alizadeh AA. Profiling tumor infiltrating immune cells with CIBERSORT. Cancer Syst Biol: Methods Protoc. 2018;1711:243–59. [Google Scholar]
17. Hu D, Zhou M, Zhu X. Deciphering immune-associated genes to predict survival in clear cell renal cell cancer. Biomed Res Int. 2019;2019:2506843. [Google Scholar] [PubMed]
18. Geeleher P, Cox N, Huang RS. pRRophetic: an R package for prediction of clinical chemotherapeutic response from tumor gene expression levels. PLoS One. 2014;9(9):e107468. [Google Scholar] [PubMed]
19. Jagla B, Libri V, Chica C, Rouilly V, Mella S, Puceat M, et al. SCHNAPPs—single cell sHiNy APPlication(s). J Immunol Methods. 2021;499:113176. [Google Scholar] [PubMed]
20. Chinopoulos C, Adam-Vizi V. Mitochondria as ATP consumers in cellular pathology. Biochim Biophys Acta (BBA)–Mol Basis Dis. 2010;1802:221–7. [Google Scholar]
21. Yang Y, Karakhanova S, Hartwig W, D’Haese JG, Philippov PP, Werner J, et al. Mitochondria and mitochondrial ROS in cancer: novel targets for anticancer therapy. J Cell Physiol. 2016;231:2570–81. [Google Scholar] [PubMed]
22. Quan Y, Xin Y, Tian G, Zhou J, Liu X. Mitochondrial ROS-modulated mtDNA: a potential target for cardiac aging. Oxid Med Cell Longev. 2020;2020:9423593. [Google Scholar] [PubMed]
23. Aventaggiato M, Vernucci E, Barreca F, Russo MA, Tafani M. Sirtuins’ control of autophagy and mitophagy in cancer. Pharmacol Therapeut. 2021;221:107748. [Google Scholar]
24. Dapas M, Lin FTJ, Nadkarni GN, Sisk R, Legro RS, Urbanek M, et al. Distinct subtypes of polycystic ovary syndrome with novel genetic associations: an unsupervised, phenotypic clustering analysis. PLoS Med. 2020;17(6):e1003132. [Google Scholar] [PubMed]
25. Wang J, Lou J, Fu L, Jin Q. An independent poor-prognosis subtype of hepatocellular carcinoma based on the tumor microenvironment. J Int Med Res. 2021;49(2):300060520980646. [Google Scholar] [PubMed]
26. Lin D, Fan W, Zhang R, Zhao E, Li P, Zhou W, et al. Molecular subtype identification and prognosis stratification by a metabolism-related gene expression signature in colorectal cancer. J Transl Med. 2021;19(1):279. [Google Scholar] [PubMed]
27. Qi L, Ye C, Zhang D, Bai R, Zheng S, Hu W, et al. The effects of differentially-expressed homeobox family genes on the prognosis and HOXC6 on immune microenvironment orchestration in colorectal cancer. Front Immunol. 2021;12:781221. [Google Scholar] [PubMed]
28. Liu Z, Zhang K, Zhao Z, Qin Z, Tang H. Prognosis-related autophagy genes in female lung adenocarcinoma. Medicine. 2022;101(1):e28500. [Google Scholar] [PubMed]
29. Chen J, Zhao X, Yuan Y, Jing JJ. The expression patterns and the diagnostic/prognostic roles of PTPN family members in digestive tract cancers. Cancer Cell Int. 2020;20(1):238. [Google Scholar] [PubMed]
30. Joung J, Kirchgatterer PC, Singh A, Cho JH, Nety SP, Larson RC, et al. CRISPR activation screen identifies BCL-2 proteins and B3GNT2 as drivers of cancer resistance to T cell-mediated cytotoxicity. Nat Commun. 2022;13(1):1606. [Google Scholar] [PubMed]
31. Wang X, Li M, Wang Z, Han S, Tang X, Ge Y, et al. Silencing of long noncoding RNA MALAT1 by miR-101 and miR-217 inhibits proliferation, migration, and invasion of esophageal squamous cell carcinoma cells. J Biol Chem. 2015;290(7):3925–35. [Google Scholar] [PubMed]
32. Tang X, Pang T, Yan WF, Qian WL, Gong YL, Yang ZG. A novel prognostic model predicting the long-term cancer-specific survival for patients with hypopharyngeal squamous cell carcinoma. BMC Cancer. 2020;20(1):1095. [Google Scholar] [PubMed]
33. Gao R, Wang Z, Liu Q, Yang C. MicroRNA-105 plays an independent prognostic role in esophageal cancer and acts as an oncogene. Cancer Biomark. 2020;27(2):173–80. [Google Scholar] [PubMed]
34. Qiu MJ, Yang SL, Wang MM, Li YN, Jiang X, Huang ZZ, et al. Prognostic evaluation of esophageal cancer patients with stages I–III. Aging. 2020;12(14):14736–53. [Google Scholar] [PubMed]
35. Semenkovich TR, Yan Y, Subramanian M, Meyers BF, Kozower BD, Nava R, et al. A clinical nomogram for predicting node-positive disease in esophageal cancer. Ann Surg. 2021;273(6):e214–21. [Google Scholar] [PubMed]
36. Lei Z, Li J, Wu D, Xia Y, Wang Q, Si A, et al. Nomogram for preoperative estimation of microvascular invasion risk in hepatitis B virus-related hepatocellular carcinoma within the Milan criteria. JAMA Surg. 2016;151(4):356–63. [Google Scholar] [PubMed]
37. Huang TX, Fu L. The immune landscape of esophageal cancer. Cancer Commun. 2019;39(1):79. [Google Scholar]
38. Zander R, Kasmani MY, Chen Y, Topchyan P, Shen J, Zheng S, et al. Tfh-cell-derived interleukin 21 sustains effector CD8+ T cell responses during chronic viral infection. Immunity. 2022;55:475–93. [Google Scholar] [PubMed]
39. Baba Y, Nomoto D, Okadome K, Ishimoto T, Iwatsuki M, Miyamoto Y, et al. Tumor immune microenvironment and immune checkpoint inhibitors in esophageal squamous cell carcinoma. Cancer Sci. 2020;111(9):3132–41. [Google Scholar] [PubMed]
40. Zou LQ, Yang X, Li YD, Zhu ZF. Immune checkpoint inhibitors: a new era for esophageal cancer. Expert Rev Anticancer Ther. 2019;19(8):731–8. [Google Scholar] [PubMed]
41. Havel JJ, Chowell D, Chan TA. The evolving landscape of biomarkers for checkpoint inhibitor immunotherapy. Nat Rev Cancer. 2019;19(3):133–50. [Google Scholar] [PubMed]
42. Rotte A. Combination of CTLA-4 and PD-1 blockers for treatment of cancer. J Exp Clin Canc Res. 2019;38(1):255. [Google Scholar]
43. Zhang H, Dai Z, Wu W, Wang Z, Zhang N, Zhang L, et al. Regulatory mechanisms of immune checkpoints PD-L1 and CTLA-4 in cancer. J Exp Clin Canc Res. 2021;40(1):184. [Google Scholar]
44. Zayac A, Almhanna K. Esophageal, gastric cancer and immunotherapy: small steps in the right direction? Transl Gastroenterol Hepatol. 2020;5:9. [Google Scholar] [PubMed]
Cite This Article
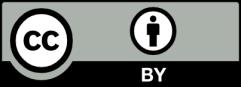
This work is licensed under a Creative Commons Attribution 4.0 International License , which permits unrestricted use, distribution, and reproduction in any medium, provided the original work is properly cited.