Open Access
ARTICLE
M2 macrophages predicted the prognosis of breast cancer by combing a novel immune cell signature and promoted cell migration and invasion of cancer cells in vitro
1 Department of PICC and Day Chemotherapy Center, Sichuan Provincial People’s Hospital, University of Electronic Science and Technology of China, Chengdu, China
2 Department of Nursing, Sichuan Provincial People’s Hospital, University of Electronic Science and Technology of China, Chengdu, 610072, China
3 Sichuan Translational Medicine Research Hospital, Chinese Academy of Sciences, Chengdu, 610072, China
* Corresponding Author: XIANXIU WEN. Email:
(This article belongs to the Special Issue: Bioinformatics Study of Diseases)
BIOCELL 2024, 48(2), 217-228. https://doi.org/10.32604/biocell.2023.027414
Received 28 October 2022; Accepted 06 February 2023; Issue published 23 February 2024
Abstract
Background: Breast cancer (BC) is the most common cancer and the leading cause of cancer death in women. Immune features play an important role in improving the prognosis prediction of BC. However, while previous immune signatures consisted mainly of immune genes, immune cell-based signatures have been rarely reported. Methods: In this study, we report that a novel immune cell signature is effective in improving prognostic prediction by combining M2 macrophages. We identified 17 differentially infiltrating immune cells between cancer and normal groups. Prognostic features of the four immune cells identified by LASSO COX analysis showed good performance for survival risk stratification in both the training and validation datasets. Independent prognostic analysis showed that M2 macrophages were significantly associated with survival in BC patients and both the cells and the risk score were the main prognostic factors independent of survival in BC patients. Results: Therefore, we combined M2 macrophages and risk scores to create a nomogram with good prognostic predictive power. Finally, we attempted to study the effect of M2 on M2 macrophage progression in BC in vitro. BC cells cultured with M2 macrophage-conditioned medium exhibited distinct malignant features, including migration and invasion. Conclusion: The findings suggest that M2 macrophages are associated with poor prognosis in breast cancer patients possibly by promoting tumor invasion and migration. This work may provide a new strategy for prognostic prediction and immunotherapy in BC.Keywords
As the most common malignancy in women, breast cancer (BC) kills more than half a million people worldwide each year and has a serious impact on the health of women [1]. The main cause of death here is the metastasis of tumor cells. High metastatic heterogeneity of breast tumor cells in BC patients reduces the accuracy of prognosis [2]. As a result, the early identification, diagnosis, and treatment of BC have declined in recent decades. Further, multimodal therapies, including surgery, radiotherapy, and chemotherapy, have proved inefficient in BC prognosis in patients with tumor metastasis [3,4]. Therefore, identifying new prognostic biomarkers in BC patients is essential to developing new treatment methods.
The rapid development of genome sequencing technology has led to the development of cancer diagnostic and prognostic capabilities using various transcriptomic molecules. These include the diagnostic and prognostic signatures composed of multiple genes and non-coding RNA, respectively, used for the diagnosis and evaluation of cancer [5]. However, a prognostic signature is urgently needed given the complex interaction between the transcriptomic molecules and tumor cells. Nevertheless, the composition of many biomolecules and immune cells in the tumor and normal environment is significantly different. Thus, it can be used to construct signatures identifying cancer processes [6]. Besides, immune cells are highly linked to cancer [7,8]. Also, the immune signal has been used as a new prognostic signature based on immunotherapy, independent of other clinical factors; thus, superior to the traditional staging methods [9].
Multiple immune parameters have been correlated with the prognostic prediction of BC. In addition, the recurrence score based on the 21-gene model predicts the risk of chemotherapy [10]. Additionally, the 70-gene model divided BC patients into high- or low-risk prognostic models for relapsed populations [11]. Further, another study established a prognostic model of immune-related genes to guide the immunotherapy of BC patients [12]. It is therefore crucial to identify prognostic indicators related to immunity in the BC tumor microenvironment.
The M2 macrophage status also guides patient treatment and helps identify new immunotherapeutic interventions. The M2 macrophage activity is influenced by tumor cell products and the cell cycle. The M2 macrophages cyclically transform into M1 macrophages [13,14]. M2 macrophages are activated to promote tissue repair, while M1 macrophages are activated to kill microorganisms and tumor cells [15]. Further, M2 macrophages secrete the Chitinase 3 Like 1 (CHI3L1) protein and promote the metastasis of dormant BC cells in the bone marrow [16,17]. Therefore, M2 macrophages may be an important biomarker for identifying BC progression.
This study investigated the prognostic significance of M2 macrophage immune cells in BC based on the The Cancer Genome Atlas (TCGA) dataset. First, we used the CIBERSORT deconvolution algorithm to analyze the infiltration rates of 22 immune cells and identified 17 tumor immune cells with differences between normal and tumor groups. Then four immune cell types that were most associated with BC prognosis were analyzed by LASSO COX. Subsequently, we used univariate and multivariate COX regression to determine the relationship between M2 macrophages and survival in BC patients, and further performed survival analyses. Finally, independent prognostic assessments of M2 macrophages and risk scores were performed, and the differential presentation of M2 macrophages in different clinical features was observed. Nomogram analysis for assessing M2 macrophages and the risk score inprognosis was also done.
Additionally, we used quantitative reverse transcription polymerase chain reaction (qRT-PCR) and western blotting to detect the expression of M2 macrophages. Cell proliferation, colony formation, and transwell assays were used to determine the in vitro function of M2 macrophages. Protein expression levels of ubiquitinated and M2 macrophage markers were analyzed by protein blotting. MDA-MB-231 breast cancer cells were assayed for their migratory and invasive abilities by cellular wound healing assays and transwell assays. Cell invasiveness was enhanced in the presence of the M2-conditioned medium. Finally, the wound healing assay and trans-well assay were performed to evaluate the migratory and invasive abilities of MDA-MB-231 breast cancer cells. This study elucidated the prognostic impact of M2 macrophages on BC and established its value in predicting prognosis and treatment response in BC patients.
The Cancer Genome Atlas (TCGA) was used to collect gene expression profiles and clinical data of BC patients. The gene expression data type is fragments per kilobase of exon per million mapped fragments (FPKM), based on high-throughput sequencing. The clinical information of the patient, including overall survival time (OS), status and tumor, node and metastases (TNM) stage were collected. We performed differential expression analysis on the data by log2 normalization of the expression data, through the limma package in the R language, and obtained differently expressed genes. Then, we extracted the differentially expressed genes in tumor and normal samples for subsequent analysis.
CIBERSORT employs the deconvolution method with three large data sets to determine the absolute immune score and abundance of each immune cell subpopulation. The method appears to be acceptable as the abundance of seven human immune cells can be estimated. CIBERSORT obtains the p-value using Monte Carlo sampling to deconvolve each sample, thereby providing measurement confidence for each estimate. Data values (p < 0.05) with significant differences can be used for further analysis. The median immunological score separated the gene expression data between the upper and lower groups. The “limma” R package was used to probe for immune-related genes (IRGs): (|log2FC| > 1 and FDP < 0.01).
Prognostic risk model construction
Combined with the clinical data downloaded from TCGA, the connection between each IRG of the patients in the training set and the OS was investigated using univariate COX regression. The relationship between patients IRGs and other clinical phenotypes was done by multivariate COX regression. LASSO COX was used to screen for IRGs with high correlation. Such genes were identified as possible survival-related IRGs.
This was applied to the development of immune-related risk models. The formula for assessing risk is as follows:
The median of the risk score (RS) was used as the cutoff value to divide all patients into high-risk and low-risk groups. Further, we examined the difference in the immune infiltration level and the survival status of both groups. ROC curves were drawn to validate their assessment capabilities.
Independent prognostic analysis
We selected M2 macrophages as the prominent factor. The risk score was computed and we examined its prognostic performance. Macrophages M2 and risk scores, as well as numerous clinical characteristics (gender, age, T, N, M, and Stage), were subjected to univariate and multivariate COX regression. Comparative analysis was performed to obtain important factors affecting BC disease.
Cell culture and macrophage polarization induction
The Human THP-1 cell line and breast cancer cell line MDA-MB-231 were purchased from the American Type Culture Collection (ATCC, Manassas, Virginia, USA), and maintained in RMPI 1640 and DMEM medium supplemented with 10% FBS, respectively. THP-1 cells were seed into 6-well plates and treated with phorbol-12-myristate-13-acetate (PMA) at 50 ng/mL for 24 h, then cultured with fresh RPMI 1640 medium for 24 h to induce M0 macrophages. Tumor associated macrophage (TAM) polarization was induced by treating M0 macrophages with MDA-MB-231 conditioned medium at 20% (v/v) for 7 days, and the medium was changed every three days. Polarized TAMs were cultured in serum-free medium for 48 h. The culture supernatant was collected as the TAM-conditioned medium for co-culture with cancer cells.
Quantitative polymerase chain reaction and western blotting
Total RNA was extracted from THP-1 and polarized M2 macrophages by using RNeasy Kit (Qiagen, Dusseldorf, Germany). cDNA was obtained by using the PrimeScript RT reagent kit (TAKARA, Shiga, Japan). Primers used for the quantitative polymerase chain reaction (qPCR) in this study are listed below. CD206-F: Ctcggttcagctattggacgc, CD206-R: Cggaatttctgggattcagcttc, CD163-F: CCAGTCCCAAACACTGTCCT, CD163-R: ATGCCAGTGAGCTTCCCGTTCAGC, CD301-F: GGTGGATGGAACAGACTATGCG, CD301-R: GGATGGAAGTGAGCACAGTCCT, ARG-1-F: TCATCTGGGTGGATGCTCACAC, ARG-1-R: GAGAATCCTGGCACATCGGGAA, Actin-F: CACCATTGGCAATGAGCGGTTC, Actin-R: AGGTCTTTGCGGATGTCCACGT.
The protein content was extracted from cells by using RIPA lysis buffer supplemented with a proteinase inhibitor cocktail. Total protein was loaded at 20 μg per well and separated by sodium dodecyl sulfate-polyacrylamide gel electrophoresis (SDS-PAGE) Gel. Proteins were transferred to a polyvinylidene difluoride (PVDF) membrane using a semi-dry transfer machine (Bio-Rad, Hercules, California, USA). After incubating with 5% non-fat milk, the membrane was incubated with primary antibodies overnight at 4°C and then incubated with HRP-conjugated secondary antibody for 1 h at room temperature. Images were captured by using a imaging machine (Bio-Rad, Hercules, California, USA). The antibodies used in this study are listed below. Antibody against β-actin (Santa Cruz Biotechnology, Dallas, Texas, USA), Antibodies against CD206 and Arg-1 (Proteintech, Rosemont, Illinois, USA) were purchased. And Antibodies against CD301 and CD163 from (Invitrogen, Waltham, Massachusetts, USA) were purchased.
Tumor cell migration and invasion assays
Tumor cells were seeded in a 6-well plate at a density of 5 × 105 cells per well. 24 h later, cells were scratched by a 20 μl tip. After washing twice with phosphate buffered saline (PBS), cells were cultured with either DMEM serum-free medium or conditioned medium at a concentration of 20% (v/v) for 24 h. Imaging was performed immediately after scratching and 24 h post-scratching. 3 wells for each group were observed. Cell invasion was performed using the BioCoat Matrigel Invasion Chamber (8 μm, 24 well) (Corning, New York, USA). MDA-MB-231 cells were suspended in serum-free DMEM medium and plated into upper chambers at a density of 2.5 × 104 per well. Simultaneously, DMEM medium alone and 20% (v/v) conditioned medium were added to the lower chamber, respectively. After 20 h, cells inside the upper chamber were carefully removed and cells on the bottom side were fixed with 4% paraformaldehyde and stained with crystal violet. Five images of each well were captured by the microscope (Olympus, Tokyo, Japan).
The nomogram is based on multivariate regression research and incorporates numerous prognostic indicators before being scaled. Line segments are drawn on the same plane according to a certain scale, so the relationship between the various variables in the prediction model can be expressed. We used a nomogram to examine the scores of each factor and judge the 1, 3 and 5-year BC survival rates.
All statistical analysis was done in R Studio software, and survival analysis through “survival” and “survminer” packages. The Kaplan-Meier (K-M) curves were examined using the log-rank test. The hazard ratios (HRs) were calculated using univariate and multivariate COX proportional hazards regression models. Among the 22 immune cell subpopulations, the key prognostic cell was chosen using the LASSO COX regression model with the Least Absolute Shrinkage and Selection Operator (LASSO) penalty. In addition, ten-fold cross-validation was used to narrow the variables. To identify independent predictive markers, we employed a multivariate COX regression model. All statistical tests were two-way, and p < 0.05 was statistically significant. Data were collected from three independent experiments and expressed as mean ± standard deviation (SD). All significance analyses, including one-way analysis of variance (ANOVA), multiple comparisons, and t-tests, were performed using Origin software. p-values of 0.05 were considered significant.
Tumor immune microenvironment alterations
The immune cell infiltration rate was determined using the CIBERSORT deconvolution method. Among the immune cell subtypes, resting memory CD4+ T cells (29.18%), M2 macrophages (25.07%), resting mast cells (8.22%), naïve B cells (6.91%), and plasma cells (6.43%) had the highest infiltration rate in the normal group (Fig. 1A). In the tumor group, the infiltration rate of the resting memory CD4+ T cells, M2 macrophages, M0 macrophages, resting mast cells, and M1 macrophages was 22.62%, 20.01%, 17.19%, 6.94% and 6.51%, respectively (Fig. 1B). Besides, there was a significant difference in memory B cells (p = 0.001), resting natural killer (NK) cells (p = 0.001), and neutrophils (p = 0.007) between the tumor and normal groups. In addition, the infiltration ratios of resting memory CD4+ T cells activated memory CD4+ T cells, T follicular helper cells, regulatory T cells (Tregs), activated NK cells, monocytes, M0 macrophages, M1 macrophages, M2 macrophages, resting dendritic cells, activated dendritic cells, resting mast cells, eosinophils, and plasma cells were significantly different (p < 0.001; Fig. 1C). Therefore, all 17 different infiltrating immune cells were categorized as differentially infiltrating immune cells (DIICs) (*p < 0.05).
Figure 1: CIBERSORT analysis of immune cell infiltration ratios. (A) The infiltration ratio of 22 immune cell types in the normal group. (B) The infiltration rate of 22 immune cell types in the tumor group. (C) Profile of differentially infiltrating immune cells (DIICs).
Construction and verification of signatures of four immune infiltrating cells
The 17 prognostic immune cells were reduced to four (Plasma cells, T cells regulatory (Tregs), M1 macrophages, and M2 macrophages) by LASSO COX analysis (Figs. 2A and 2B). Next, the patient’s risk score (RS) was calculated based on the four immune cell scores multiplied by the LASSO coefficient as follows:
Figure 2: The construction of the prognostic signature. (A) The four immune cell types obtained by the Least Absolute Shrinkage and Selection Operator (LASSO) regression analysis. (B) Partial likelihood deviation of the LASSO coefficient distribution. The two vertical dashed lines indicate the Lambda.min and lambda.1se. (C) Survival rates and immune cell distribution in high-risk and low-risk groups.
RS = 0.2637* plasma cells + 1.3418* regulatory T cells (Tregs) − (−0.2662)* M1 macrophages + 1.8123* M2 macrophages, using the median as the critical value. All the samples were categorized as either high or low-risk. The TCGA, patient status, and pattern of 4-cell signatures are presented in Fig. 2C.
Of the 1069 samples assayed, 534 were categorized as low-risk and 535 as high-risk. The high-risk samples had a good prognosis compared to the low-risk (p = 0.0048; Fig. 3A) with a predicted RS area under the curve (AUC) of 1, 3 and 5 year, AUC1 = 0.660, AUC3 = 0.602, and AUC5 = 0.618 (Fig. 3B). Besides, the high-risk group significantly outperformed the low-risk group in terms of prognosis (p = 0.0075; Fig. 3C) with the RS AUC of AUC1 = 0.590, AUC3 = 0.680, and AUC5 = 0.693 (Fig. 3D).
Figure 3: Establishment and verification of the 4-cell prognostic signature. (A) Survival analysis based on 4-cell prognostic signature risk score in the The Cancer Genome Atlas (TCGA). (B) The predictive power of risk scores for patients with 1-year, 3-year, and 5-year survival rates in the TCGA. (C) Survival analysis based on the risk score of the 4-cell signature in the validation cohort. (D) Verification of the predictive power of the risk scores for the 1-year, 3-year, and 5-year survival rates in the cohort of patients.
Correlating clinical manifestations and M2 macrophages
Univariate and multivariate COX regression analysis on the four immune cells revealed that M2 macrophages were closely related to OS (Table 1). Based on the survival analysis, there was a low survival rate of patients, closely associated with the increased infiltration rate of M2 macrophages (p < 2.22e-16; p = 0.00083; Figs. 4A and 4B). The predicted OS AUC were AUC1 = 0.660, AUC3 = 0.590, and AUC5 = 0.610 (Fig. 4C).
Figure 4: Clinical associationsof M2 macrophages in the The Cancer Genome Atlas (TCGA) cohort. (A) The M2 macrophage infiltration patterns in the high- and low-risk groups. (B) Survival analysis with M2 macrophages. (C) Applying the M2 macrophage predictions for BC patient survival rates.
In the validation cohort, the M2 macrophages had similar clinical features. Their infiltration rate was higher in the high-risk group than the low-risk group (p < 2.22e-16; Fig. 5A), which was closely associated with the poor survival rate of patients (p = 0.00075; Fig. 5B). Their predicted OS AUC were AUC1 = 0.660, AUC3 = 0.680, and AUC5 = 0.700 (Fig. 5C).
Figure 5: Clinical manifestations of M2 macrophages in the validation cohort. (A) The infiltration pattern of M2 macrophages in the high-and low-risk groups. (B) Survival analysis with M2 macrophages. (C) The predictive capacity of M2 macrophages for BC patients with 1-year, 3-year, and 5-year survival rates.
Correlation analysis between the M2 macrophages and pathological features revealed that the M2 macrophage infiltration rate in patients aged ≥67 was significantly higher than those <67 years (p = 7.31e-06; Fig. 6A). Interestingly, the M2 macrophage infiltration rate was significantly correlated with the degree of size (T) (p = 0.049), spread to lymph nodes (N) (p = 0.041), and stage of lesions (p = 0.015; Figs. 6B–6D).
Figure 6: The clinical relevance of M2 macrophages in BC. (A) The correlation between infiltration level differences of M2 macrophages in different age groups. (B) The correlation between the infiltration level of M2 macrophages and the T stage (the size of the tumor and any spread of cancer into nearby tissue). (C) The correlation between the infiltration level of M2 macrophages and the N stage (spread to nearby lymph nodes). (D) The correlation between the infiltration level of M2 macrophages and the stage.
Independent prognostic analysis identified both M2 macrophages and risk scores were crucial prognostic risk factors
An independent prognostic analysis of M2 macrophages, risk scores and multiple clinical characteristics (gender, age, T, N, M, and Stage) was performed through the univariate and multivariate COX regression. The risk score (HR = 4.6447; p < 0.001; Fig. 7A) and M2 macrophages (HR = 11.722, p < 0.001; Fig. 7B) were BC progression risk variables based on the univariate COX regression analysis on the TCGA cohort. Multivariate analysis further revealed that the risk score (HR = 2.761, p = 0.0215; Fig. 8A) and M2 macrophages (HR = 5.555, p = 0.0246; Fig. 8B) were the main crucial survival predictors of BC patients, with other clinical parameters having a minimal effect.
Figure 7: Univariate COX regression analysis. (A) COX analysis of the risk score. (B) COX analysis of M2 macrophages.
Figure 8: Multivariate COX regression analysis. (A) The risk score and the multivariate COX model. (B) The M2 macrophages and the multivariate COX model.
Nomogram of M2 macrophage and risk score and its prediction
The risk score and M2 macrophages were suitable indicators for nomogram construction since they had high prognostic capabilities. In addition, RS (C-index = 0.734) and M2 macrophages (C-index = 0.712) had good predictive potentials. Thus, the nomogram composed of M2 macrophage and risk score had a good predictive capability (C-index = 0.766; Fig. 9A). Besides, the 1-, 3-, and 5-year OS prediction rates revealed that the nomogram was capable of accurate predictions (Fig. 9B).
Figure 9: Nomogram-predicted survival rate. (A) Nomogram constructed with RS and M2 macrophages as factors. (B) The Nomogram survival probabilities vs. the actual 1-year, 3-year, and 5-year survival probabilities.
Tumor associated macrophage-conditioned medium promoted in vitro breast cancer cell migration and invasion
TPH-1 cells were polarized to TAMs by incubating with the cultured medium from MDA-MB-231 culture (Fig. 10A). Cell markers were examined by qRT-PCR (Fig. 10B) and western blotting (Fig. 10C). The expression of M2 macrophage markers CD163, CD206, CD 301, and Arg-1 were significantly lowered after polarization. The migration and invasion abilities of MDA-MB-231 cells were evaluated by the wound-healing assay and trans-well assay, respectively. The migration distance in the M2-conditioned medium was significantly expanded compared to the control group (Figs. 10D and 10E). The cell invasion was also enhanced in the presence of M2-conditioned medium (Fig. 10F). These results suggested that in the presence of TAMs, tumor cells exhibited more malignant characteristics, explaining the extremely strong relationship between M2-like TAMs and poor prognosis.
Figure 10: M2 supernatant promoted breast cancer cell migration and invasion. (A) Representative images of TAM polarization. Scale bar: 100 μm. (B) Quantitative polymerase chain reaction (qPCR) for TAMs polarization. (C) Western blotting for TAMs polarization. (D) Representative images of the wound healing assay, and evaluation of the migration ability of MDA-MB-231 cells, scale bar: 100 μm. (E) Statistical analysis for the cell migration distance (n = 6). (F) Representative images of the trans-well invasion assay, evaluating the invasive ability of MDA-MB-231 cancer cells. Statistical analysis for the number of cells (n = 5). *p < 0.05, **p < 0.01, ***p < 0.001.
The present study identified four immune cells most relevant to the BC prognosis through differential analysis of 22 immune cell types and LASSO regression analysis. Their expression levels in the tumor microenvironment were predicated by constructing a prognostic signature, scoring the BC samples against the signature, and then assigning them to high-risk or low-risk groups. The novel immune cell signature had an outstanding predictive ability.
COX regression analysis was performed on these four types of immune cells. Next, the expression and clinical manifestations of M2 macrophages in BC patients were analyzed to determine the predictive ability of M2 macrophages on BC progression. Subsequently, the independent prognosis of M2 macrophages and a risk score analysis based on the M2 macrophage infiltration level and various clinical indicators were performed. The risk score was highly correlated with the patient’s OS. The area under the ROC curve indicated very good prognostic ability. The specific qRT-PCR and protein blotting assays in this study showed that the M2 culture supernatant promoted breast cancer cell line migration and invasion. Protein blotting analysis showed the protein levels in control and M2 macrophages. Representative images for the wound healing assay were used to evaluate the migratory capacity of MDA-MB-231 cells. Similarly, representative images were used for the transwell invasion assay to assess the invasive ability of MDA-MB-231 cancer cells. The results indicate that MDA-MB-231 cells had pro-metastatic and invasive functions. The above evidence suggests that M2 macrophages can regulate the tumor immune microenvironment and promote breast cancer metastasis.
Recently, TAMs have attracted a great deal of attention because they are frequently documented as abundant immune cells in the tumor stroma of a wide range of cancers. The high abundance of these cells is usually associated with poorer clinical outcomes [18]. M2 macrophages, on the other hand, are important functional cells that promote immunosuppression and prolong drug efficacy in the tumor microenvironment (TME) [19,20]. Moreover, macrophages are abundantly present in the TME of most cancers, where they promote invasion and angiogenesis, metastasis, and increase immunosuppression [21]. The importance of M2 macrophages in the TME has generated interest in therapeutic approaches that aim at reducing the immunosuppression of TAMs or increasing macrophage phagocytosis [22,23]. Current macrophage-based immunotherapeutic approaches are mechanistically dependent on the TME and frequently exhibit an immunosuppressive M2 phenotype, which promotes tumor growth and facilitates resistance to therapy [24]. Combined treatment with the tumor environment and M2 macrophages often accelerates the development of untreated tumors [25,26] and compromises the efficacy of anti-cancer drugs [27].
The TME covers the tumor cells and the surrounding cells, such as the normal epithelial and stromal cells, immune cells, and vascular cells [28]. Further, abnormal immune functions in the TME is a hallmark of cancer [29]. In addition, immune cells impact cancer patient prognosis due to the heterogeneity of tumors and immune cell infiltration [30,31]. The infiltration of Treg cells suppresses tumors and is associated with prognosis [28,32,33), while the infiltration of dendritic cells (DC) is usually associated with positive clinical results [34]. However, dendritic cells favor the infiltration of Tregs in breast tumors, leading to a poor prognosis [35]. On the other hand, plasma cells inhibit interferon (IFN)-α production [36].
The rapid development of genome sequencing technology has enabled more studies on BC prognostic signatures. For example, independent prognostic biological target genes such as p53 (p < 0.008) and EZH2 (p < 0.0045) were shown to have a good prognostic ability within 5 years [37]. In the present study, the four immune cell prognostic signatures developed had significant prognostic capacities (p < 0.0075), with M2 macrophages having a better independent prognostic capacity (p < 0.00075). While immune cells did not necessarily undergo transcriptomic regulation in tumor cells, they improved the reliability and highlighted the clinical significance of cell signatures.
We performed univariate independent prognostic analyses to compare the clinical survival time and survival status. In the true sense, only multiple factors can be used to verify whether a subject can be a factor that is related to OS. The multivariate analysis amalgamated these clinical traits and our risk value together to compare with the obtained survival time and survival state. This confirmed that the risk score can be employed as a predictive indicator independent of other clinical characteristics.
Macrophages are key immune cells highly related to inflammatory responses [29]. Based on their activation status and function, macrophages can be classified as M1 and M2 types. The M1 macrophages play key roles in phagocytosis and microbicial activities, presentation of antigens, and initiation of adaptive immune responses, while the M2 macrophages are activated to kill microorganisms and tumor cells. Therefore, the activation of M2 macrophages is highly correlated with their infiltration into tumor cells, which serves as an indicator of cancer progression. The M2 macrophages in the TEM also secrete chemokines to recruit T cells and dendritic cells [30]. Therefore, M2 macrophages as a new immune target offer significant advantages in BC clinical prognosis.
In this study, the immune signature of tumor-infiltrating immune cells (TICs) could predict the prognostic outcome of BC patients. However, our study also has inevitable limitations. Firstly, the datasets we used are public datasets with biases and limitations. These results may require large-scale, multi-center, evidence-based medical studies for verification. Secondly, this is a retrospective study, and data bias may affect survival and immune cell characteristics. Our results require prospective studies for validation. Thirdly, although the specific mechanism of the M2 macrophage immune cells in the CIBERSORT-based study has been reported, the data still needs to be experimentally verified.
We constructed a novel immune cell signature that was combined with M2 macrophages to enable an effective prognostic prediction of BC patients. The M2 macrophages served as an independent prognostic factor for BC and promoted cell migration and invasion of breast cancer cells in vitro. The findings suggest that M2 macrophages are associated with poor prognosis in breast cancer patients possibly by promoting tumor invasion and migration. This work may provide a new strategy for BC prognosis prediction and immunotherapy.
Acknowledgement: None.
Funding Statement: This study was funded by the Construction and Practice of Management Cloud Platform for Intravenous Therapy Specialist Alliance, Sichuan Provincial Health Commission of Sichuan Provincial (l9pj120).
Author Contributions: The authors confirm their contribution to the paper as follows: QX collected the data and wrote the manuscript. XC and QM analyzed the data. XW proposed the idea. All authors contributed to the paper and approved the submitted version.
Availability of Data and Materials: All data generated or analyzed during this study are included in this published article.
Ethics Approval: Not applicable.
Conflicts of Interest: The authors declare that they have no conflicts of interest to report regarding the present study.
References
1. Mavingire N, Campbell P, Wooten J, Aja J, Davis MB, Loaiza-Perez A, et al. Cancer stem cells: culprits in endocrine resistance and racial disparities in breast cancer outcomes. Cancer Lett. 2021;500:64–74. [Google Scholar]
2. Schwartz RS, Erban JK. Timing of metastasis in breast cancer. N Engl J Med. 2017;376:2486–8. [Google Scholar] [PubMed]
3. Weigel MT, Dowsett M. Current and emerging biomarkers in breast cancer: prognosis and prediction. Endocr-Relat Cancer. 2010;17:R245–62. [Google Scholar] [PubMed]
4. Emens LA. Breast cancer immunotherapy: facts and hopes. Clin Cancer Res. 2018;24:511–20. [Google Scholar] [PubMed]
5. Deng X, Bi Q, Chen S, Chen X, Li S, Zhong Z, et al. Identification of a five-autophagy-related-lncRNA signature as a novel prognostic biomarker for hepatocellular carcinoma. Front Mol Biosci. 2020;7:611626. [Google Scholar] [PubMed]
6. Hu X, Schwarz JK, Lewis JJ, Huettner PC, Rader JS, Deasy JO, et al. A microRNA expression signature for cervical cancer prognosis. Cancer Res. 2010;70(4):1441–8. [Google Scholar] [PubMed]
7. Joshi NS, Akama-Garren EH, Lu Y, Lee DY, Chang GP, Li A, et al. Regulatory T cells in tumor-associated tertiary lymphoid structures suppress anti-tumor T cell responses. Immun. 2015;43(3):579–90. [Google Scholar]
8. Saxena M, Bhardwaj N. Re-emergence of dendritic cell vaccines for cancer treatment. Trends Cancer. 2018;4(2):119–37. [Google Scholar] [PubMed]
9. Meng Y, Yang Y, Zhang Y, Yang X, Li X, Hu C. The role of an immune signature for prognosis and immunotherapy response in endometrial cancer. Am J Transl Res. 2021;13:532–48. [Google Scholar] [PubMed]
10. Kumar S, Meena R, Paulraj R. Role of macrophage (M1 and M2) in titanium-dioxide nanoparticle-induced oxidative stress and inflammatory response in rat. Appl Biochem Biotech. 2016;180(7):1257–75. [Google Scholar]
11. Chen Y, Zhang S, Cao J, Zhang X. Shrimp antiviral mja-miR-35 targets CHI3L1 in human M2 macrophages and suppresses breast cancer metastasis. Front Immunol. 2018;9. [Google Scholar]
12. Zhao Y, Pu C, Liu Z. Exploration the significance of a novel immune-related gene signature in prognosis and immune microenvironment of breast cancer. Front Oncol. 2020;10:1211. [Google Scholar] [PubMed]
13. Kishore A, Petrek M. Roles of macrophage polarization and macrophage-derived miRNAs in pulmonary fibrosis. Front Immunol. 2021;12:678457. [Google Scholar] [PubMed]
14. Ribeiro A, Silva E, Araujo P, Souza ST, Fonseca E, Barreto E. Application of raman spectroscopy for characterization of the functional polarization of macrophages into M1 and M2 cells. Spectrochim Acta Part A: Mol Biomol Spectrosc. 2021;265(1):120328. [Google Scholar]
15. Radzun HJ, Parwaresch MR. Differential immunohisto-chemical resolution of the human mononuclear phagocyte system. Cell Immunol. 1983;82(1):174–83. [Google Scholar] [PubMed]
16. Chen Y, Zhang S, Wang Q, Zhang X. Tumor-recruited M2 macrophages promote gastric and breast cancer metastasis via M2 macrophage-secreted CHI3L1 protein. J Hematol Oncol. 2017;10:36. [Google Scholar] [PubMed]
17. Kwak EJ, Hong JY, Kim MN, Kim SY, Kim SH, et al. Chitinase 3-like 1 drives allergic skin inflammation via Th2 immunity and M2 macrophage activation. Clin Exp Allerg. 2019;49:1464–74. [Google Scholar] [PubMed]
18. Steidl C, Lee T, Shah SP, Farinha P, Han G, et al. Tumor- associated macrophages and survival in classic Hodgkin’s lymphoma. N Engl J Med. 2010;362:875–85. [Google Scholar] [PubMed]
19. Cassetta L, Kitamura T. Macrophage targeting: opening new possibilities for cancer immunotherapy. Immunol. 2018;155:285–93. [Google Scholar]
20. Saeed M, Gao J, Shi Y, Lammers T, Yu H. Engineering nanoparticles to reprogram the tumor immune microenvironment for improved cancer immunotherapy. Theranostics. 2019;9:7981–8000. [Google Scholar] [PubMed]
21. Noy R, Pollard JW. Tumor-associated macrophages: from mechanisms to therapy. Immunity. 2014;41:49–61. [Google Scholar] [PubMed]
22. Morrison C. Immuno-oncologists eye up macrophage targets. Nat Rev Drug Discov. 2016;15:373–4. [Google Scholar] [PubMed]
23. Weiskopf K. Cancer immunotherapy targeting the CD47/SIRPα axis. Eur J Cancer. 2017;76:100–9. [Google Scholar] [PubMed]
24. Rodell CB, Arlauckas SP, Cuccarese MF, Garris CS, Li R, Ahmed MS, et al. TLR7/8-agonist-loaded nanoparticles promote the polarization of tumour-associated macrophages to enhance cancer immunotherapy. Nat Biomed Eng. 2018;2:578–88. [Google Scholar] [PubMed]
25. Wynn TA, Chawla A, Pollard JW. Macrophage biology in development, homeostasis and disease. Nat. 2013;496:445–55. [Google Scholar]
26. Pittet MJ, Nahrendorf M, Swirski FK. The journey from stem cell to macrophage. Ann N Y Acad Sci. 2014;1319(1):1–18. [Google Scholar] [PubMed]
27. de Palma M, Lewis CE. Macrophage regulation of tumor responses to anticancer therapies. Cancer Cell. 2013;23(3):277–86. [Google Scholar] [PubMed]
28. Li Z, Li Y, Wang X, Yang Q. Identification of a six-immune- related long non-coding RNA signature for predicting survival and immune infiltrating status in breast cancer. Front Genet. 2020;11:680. [Google Scholar] [PubMed]
29. Wang J, Shi W, Miao Y, Gan J, Guan Q, Ran J. Evaluation of tumor microenvironmental immune regulation and prognostic in lung adenocarcinoma from the perspective of purinergic receptor P2Y13. Bioeng. 2021;12(1):6286–304. [Google Scholar]
30. Fletcher M, Goldstein AL. Recent advances in the understanding of the biochemistry and clinical pharmacology of interleukin-2. Lymphokine Res. 1987;6:45–57. [Google Scholar] [PubMed]
31. Ciavarra RP, Brown RR, Holterman DA, Garrett M, Glass WN, Wright GJ, et al. Impact of the tumor microenvironment on host infiltrating cells and the efficacy of flt3-ligand combination immunotherapy evaluated in a treatment model of mouse prostate cancer. Cancer Immunol Immun. 2003;52:535–45. [Google Scholar]
32. Elsheshtawy NM, Saad M, El-Ghamrini YM, Ziada KW. Determination of a CD4+CD25+ Foxp3+ T cells subset in Egyptian colorectal cancer patients. Egypt J Pediatr Allerg Immunol. 2021;28:145–56. [Google Scholar]
33. Gamez-Diaz L, Grimbacher B. Immune checkpoint deficiencies and autoimmune lymphoproliferative syndromes. Biomed J. 2021;44:400–11. [Google Scholar] [PubMed]
34. Peng R. Distribution of S-100 protein-positive dendritic cells in transitional cell carcinoma of human bladder and its relation to clinical prognosis. Zhonghua Yi Xue Za Zhi. 1991;71:579–80+40 (In Chinese). [Google Scholar]
35. Yan H, Qu J, Cao W, Liu Y, Zheng G, Zhang E, et al. Identification of prognostic genes in the acute myeloid leukemia immune microenvironment based on TCGA data analysis. Cancer Immunol Immun. 2019;68:1971–8. [Google Scholar]
36. Dinarello CA, Cannon JG, Wolff SM, Bernheim HA, Beutler B, Cerami A, et al. Tumor necrosis factor (cachectin) is an endogenous pyrogen and induces production of interleukin 1. J Exp Med. 1986;163:1433–50. [Google Scholar] [PubMed]
37. Tang L, Mei Y, Shen Y, He S, Xiao Q, Yin Y, et al. Nanoparticle-mediated targeted drug delivery to remodel tumor microenvironment for cancer therapy. Int J Nanomed. 2021;16:5811–29. [Google Scholar]
Cite This Article
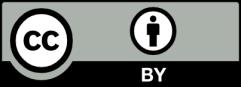
This work is licensed under a Creative Commons Attribution 4.0 International License , which permits unrestricted use, distribution, and reproduction in any medium, provided the original work is properly cited.