Open Access
ARTICLE
Identification of EML4 as a key hub gene for endometriosis and its molecular mechanism and potential drug prediction based on the GEO database
1 Reproductive Medicine Center, The Second Affiliated Hospital of Soochow University, Suzhou, 215002, China
2 Imaging Diagnosis Department, The Second Affiliated Hospital of Soochow University, Suzhou, 215002, China
* Corresponding Author: Hong Zhang,
# Equal contribution
(This article belongs to the Special Issue: Bioinformatics Study of Diseases)
BIOCELL 2023, 47(9), 2059-2068. https://doi.org/10.32604/biocell.2023.030565
Received 13 April 2023; Accepted 29 May 2023; Issue published 28 September 2023
Abstract
Objective: Key genes were screened to analyze molecular mechanisms and their drug targets of endometriosis by applying a bioinformatics approach. Methods: Gene expression profiles of endometriosis and healthy controls were obtained from the Gene Expression Omnibus database. Significant differentially expressed genes were screened using the limma package. Correlation pathways were screened by Spearman correlation analysis on the echinoderm microtubule-associated protein-like 4 (EML4) and enrichment in endometriosis pathways and estimated by the GSVA package. Immune characteristics were assessed by the “ESTIMATE” R package. Potential regulatory pathways were determined by enrichment analysis. The SWISS-MODE website was used in homology modeling with EML4 and EML4 protein activity was predicted. VarElect was employed in molecular docking for screening potential compound inhibitors targeting endometriosis. Results: Ten endometriosis and 10 normal samples were included. EML4 was significantly upregulated in endometriosis (p < 0.05). Thirty significantly correlated pathways involving 18 positive and 12 negative correlations, including GLYCOSAMINOGLYCAN_BIOSYNTHESIS_HEPARAN_SULFATE and GLYCOSPHINGOLIPID_BIOSYNTHESIS_GANGLIO_SERIES were screened between EML4 and endometriosis. Immunocorrelation analysis showed a significant difference in immune-related pathways in endometriosis and normal samples (p < 0.05). In endometriosis, EML4 was associated with T-cell CD4 resting memory, activated mast cells, plasma cells, activated NK cells, M2 macrophages, and follicular helper T cells (p < 0.05). Molecular docking identified five potential inhibitors of EML4, and compound DB05104 (asimadoline) bound well to EML4 protein to exert its physiological effects. Conclusion: Differential gene expression and immune correlation analyses revealed that EML4 may affect endometriosis through multiple targets and pathways, the mechanism of which involved immune cell activation and infiltration. Molecular docking and dynamics simulation verified DB05104 as a potential inhibitor of EML4 and a powerful target for endometriosis treatment.Graphical Abstract
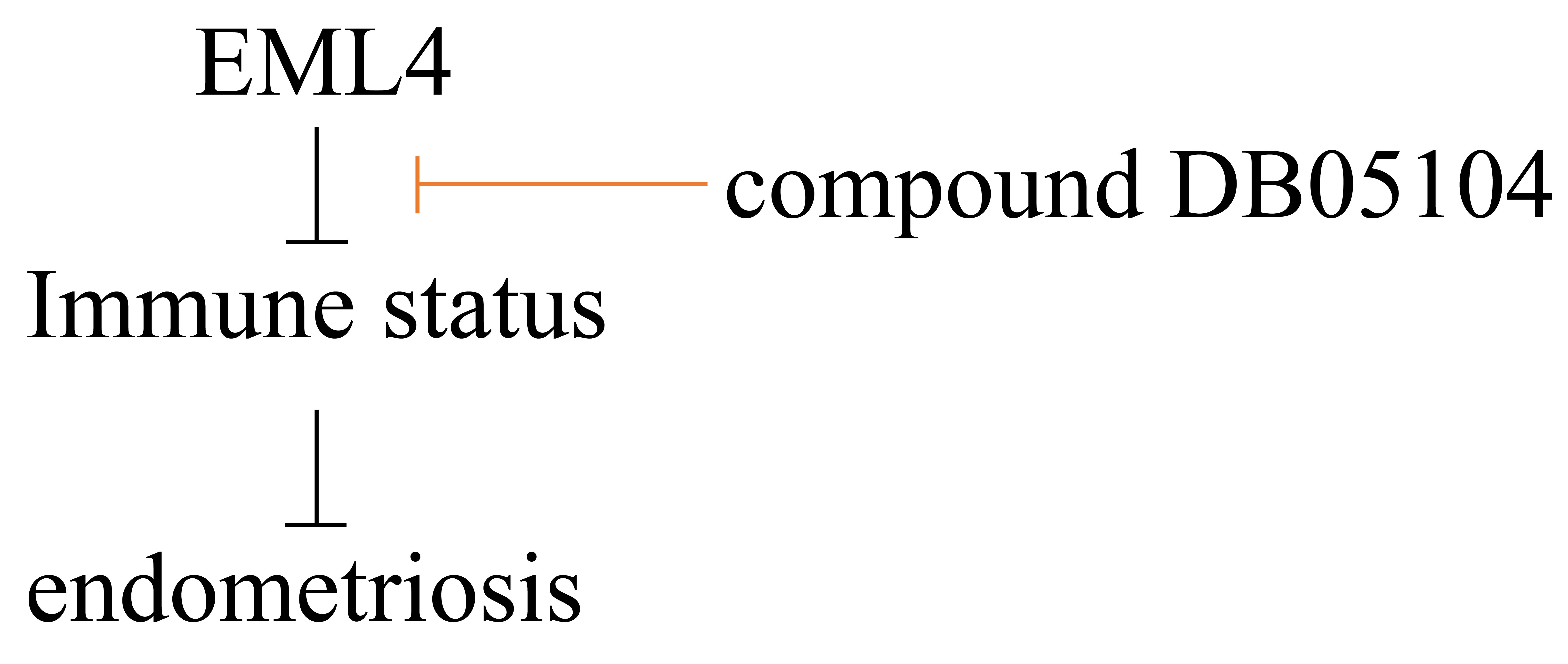
Keywords
Endometriosis is a condition that affects women in their reproductive age and affects 5%–10% of women in that age period (Simoens et al., 2012). Nearly 50%–80% of patients with pelvic pain suffer from endometriosis and more than 176 million women worldwide have endometriosis (Giudice, 2010). Despite a high prevalence, impercipient understanding of endometriosis contributes to a late diagnosis ranging from 4 to 11 years, and up to 65% of women are initially misdiagnosed (Greene et al., 2009). Progesterone and combination contraceptives are currently the first-line treatment for endometriosis (Ferrero et al., 2018). Second-line drugs such as oxalagoride are approved for the treatment of moderate to severe pain associated with endometriosis. Almost all current treatments available for endometriosis are suppressive rather than curative. With further study of the molecular mechanism related to endometriosis, molecular targeted drugs may lead to new thinking on the treatment of endometriosis. Therefore, an in-depth exploration of the pathogenesis of endometriosis is necessary to provide a theoretical basis for the development of novel therapeutic targets.
Recent studies have confirmed that endometriosis is closely related to immune functions (Crispim et al., 2021; Kolanska et al., 2021). Decreased clearance of endometrial reflux and immune response by immune active cells in the pelvis are important mechanisms for the survival of ectopic foci. Once endometriotic foci are formed, endometrial cells produce autoimmune antibodies, resulting in subsequent changes in the biological properties of ectopic foci and endothelial cells of the official cavity (Jeljeli et al., 2020; Symons et al., 2018). The function of the normal human endometrium depends on the interaction between immune cells and is regulated by NK-T cells, B cells, T cells, etc., and cytokines in the presence of steroid hormones (Kim et al., 2019; Szukiewicz, 2022; Zhou et al., 2019). Therefore, endometriosis is a result of reduced immune recognition of retrograde endothelial tissue by locally immunologically active cells and dysfunctional immune regulation. Theoretically, targeting immune cells in endometriosis can cause “pseudo-menopause” or “pseudo-pregnancy” in the body, reduce abnormal endometrial proliferation and control the recurrence of lesions.
The development of high-throughput technologies has enabled the discovery of the genes involved in endometriosis. This research explored the association of endometriosis with immunity by comparing endometriosis and normal samples and identified EML4 as a key gene in the regulation of immune cells in endometriosis. Drug prediction and molecular docking assays identified the compound DB05104 (asimadoline) as a potential targeted endometriosis inhibitor, pointing out a new direction for the subsequent basic research and clinical drug development of endometriosis.
Sample source and data processing
“Endometriosis,” “Immune,” “Expression profiling,” and “drug” were used as search terms to download endometriosis sequencing datasets from the Gene Expression Omnibus (GEO) database. The scRNA-seq (GSE175453) dataset was selected, and the “Top Organisms” dataset was selected as “Homo sapiens,” with the sample size being set to ≥20. GSE7305 containing 10 endometriosis and 10 normal endometrial samples, was included.
The gene expression profile files and gene annotation files on the annotation platform GPL570 were downloaded. One probe corresponding to multiple gene names was removed, but for multiple probes corresponding to one gene name, the mean value was taken.
Acquisition of the EML4 gene and its expression in endometriosis
We first calculated the gene expression differences between endometriosis and normal endometrial samples using the “limma” package in R language, and LogFC (fold change) >2 and p < 0.05 was set as the statistical threshold criteria. Then we used the "GSVA" package to calculate the Molecular Signatures Database (MSigDB) hallmark pathway score for the genes with the most significant expression differences. After setting |cor| > 0.5 and a false discovery rate (FDR) adjusting p < 0.05 as statistically significant differences, the correlation of pathways and genes with significant differences in endometriosis and normal endometrium were calculated by Spearman’s correlation analysis.
Relationship between immunity and endometriosis and EML4 genes
The three scores (StromalScore, ImmuneScore, ESTIMATEScore) of the GSE7305 dataset were assessed by the “ESTIMATE” R package (Yoshihara et al., 2013), and the “ssGSEA” package was applied to calculate the scores of 22 immune cell types. Pearson correlation coefficients of immune scores with key genes EML4 were then determined and visualized (Yi et al., 2020). To explore the correlation of EML4 with the pathway, we downloaded the immune pathway-related genes from the Kyoto Encyclopedia of Genes and Genomes (KEGG) website and visualized them using the “pheatmap” package (Diao et al., 2018).
Screening of potential regulatory pathways and related gene sets of EML4 gene
Single sample gene set enrichment analysis (ssGSEA) was performed on the pathway sets in the HALLMARK database using the “GSVA” package, followed by the correlation analysis between EML4 genes and pathway scores using the rcorr function in the “Hmisc” package under the statistical criteria of |cor| > 0.3 and a FDR adjusted p < 0.05. Then, we used “WebGestaltR” package to enrich the EML4 gene set and screened the key enriched genes by FDR adjusted p < 0.05. The “clusterprofiler” was utilized to perform Gene Ontology (GO) enrichment analysis on the screened gene sets.
Prediction of relevant gene sets and potential target therapeutics
The use of key genes as potential therapeutic targets is a cornerstone in developing therapeutics for endometriosis. The proximity of drugs and endometriosis was calculated based on drug-target pairs and protein-protein interaction (PPI) network (threshold score of 400) from the Drugbank database (https://go.drugbank.com/). Given S (endometriosis-related set of genes), T (drug target gene set), D (degree of related gene set nodes in the PPI), and the distance d(s,t) as the shortest path between node s and node t (where s ∈ S, the EE-related genes; t ∈ T, the drug target genes), the calculation is shown as the formula (1) below:
where ω indicates the weight of the target gene. If the target gene was a gene in the set of EE-related genes, it was calculated as ω = −ln (D+1), otherwise ω = 0.
Subsequently, the distance d(S,R) between these simulated drug targets and the key gene set was measured. After 10,000 random iterations, simulated reference distributions were generated. The mean and standard deviation of the reference distribution and the corresponding actual observed distances was converted to standardized scoring with μd(S, R) and σd(S, R), that is, the proximity Z, using the formula (2) as follows:
Finally, distance density distribution plot of the gene set was generated by normalizing the scoring of the distance.
Molecular docking facilitates drug design based on the interaction between receptors and drug molecules and the characteristics of receptors. As a simulation method with a focus on intermolecular (e.g., ligand and receptor) interactions and prediction of their binding modes and affinity, molecular docking has emerged as a useful computer-aided drug research technique (Lohning et al., 2017; Saikia and Bordoloi, 2019). The SWISS-MODEL website (https://swissmodel.expasy.org/) was used for homology modeling to obtain the 3D structure of the echinoderm microtubule associated protein-like 4 (EML4) protein. The coordinates of the predicted active sites were 163.95, 194.57, and 336.10 Å. Autodock Vina software was employed for molecular docking. All the input files were prepared on AutoDockTools 1.5.6. The pdb files of the proteins were obtained from Protein Data Bank with PDB ID 6SUK. Water molecules and protein B chains, potassium ions were eliminated from the system but polar hydrogens were added. The PDBQT file of the receptor protein species was set to +2.0. During the molecular docking, the coordinates of the grid box (grid) in each direction of XYZ were −19.5, 74.5, and 34.8 Å. The length in each XYZ direction was 20 Å. The strongest binding mode of the ligand molecule was determined using the Lamarckian algorithm. The exhaustiveness was 8, the maximum number of conformations output was 10, and the maximum energy range was set to 3 kcal/mol. The results were processed in Pymol.
Gene expression differences between endometriosis and normal endometrial samples were compared by Student’s t-test. Spearman’s test was used to examine the correlation and significance of the pathways and genes significantly different between the two sample types. The “clusterprofiler” package was used for enrichment pathway analysis, and the “GSVA” package was used for immunocorrelation analysis. Enrichment analysis on the EML4 gene set was performed in the “WebGestaltR” package. Statistical analyses were operated in R (version 4.2.1, https://www.r-project.org/), with FDR-adjusted p < 0.05 as a statistically significant difference.
Association of EML4 gene with endometriosis
To detect the differences in gene expression between endometriosis and normal endometrial samples, we first compared the expression of differential expression genes, in which EML4 was significantly upregulated in endometriosis (p < 0.05, Fig. 1A). After that, we calculated the scores of genes with significantly differential expression by performing the KEGG pathways in the “GSVA” package and found a total of 125 significantly enriched pathways (Fig. 1B), including BETA_ALANINE_METABOLISM, TAURINE_AND_HYPOTAURINE_METABOLISM, GLYCOSPHINGOLIPID_BIOSYNTHESIS_GANGLIO_SERIES, ABC_TRANSPORTERS, etc. Further analysis of the relationship between EML4 and the 125 pathways was conducted using the Spearman correlation test. EML4 was observed to be significantly correlated with a total of 30 pathways (18 positive and 12 negative correlations), including GLYCOSAMINOGLYCAN_BIOSYNTHESIS_ HEPARAN_SULFATE, GLYCOSPHINGOLIPID_BIOSYNTHESIS_GANGLIO_SERIES, PRIMARY_BILE_ACID_BIOSYNTHESI (Fig. 1C), etc.
Figure 1: Relationship between the EML4 gene and endometriosis. (A) Comparison of EML4 gene expression in endometriosis (EE) and normal controls (t.test). (B) Heat map of pathway enrichment scores with significance in endometriosis and normal controls. (C) Heat map of correlation of pathways significantly associated with the EML4 gene. *p < 0.05; **p < 0.01; ***p < 0.001; ****p < 0.0001.
Correlation analysis of EML4 and immune
To investigate whether endometriosis was immune-related, the expression differences of immune-related genes in endometriosis and normal endometrium samples were compared. Here we observed significant differences (p < 0.05) in genes in immune-related pathways between endometriosis and normal endometrium samples, such as GNG4, CXCL8, CXCL2, CCL21, ELMO1, etc. (see the heat map for more details; Fig. 2). After that, the composition of 22 immune cells between the two types of samples were analyzed, and it has been found that T cells CD4 resting memory, plasma cells, NK cells activated, T cells follicular helper, mast cells activated, M2 macrophages were significantly different between endometriosis samples and the normal ones (p < 0.05, Fig. 3A). Furthermore, StromalScore, ImmuneScore, and ESTIMATEScore were significantly higher in endometriosis than in the normal ones (p < 0.05, Fig. 3B), suggesting a higher degree of immune infiltration in endometriosis patients. To further explore the correlation between the EML4 gene and immune, the relationship of the EML4 gene expression with immune score was assessed by Spearman’s method, and we observed that a higher expression of EML4 gene was positively related to a higher degree of immune infiltration (Fig. 3C). Meanwhile, there was a significant positive correlation between EML4, T cells CD4 resting memory, and macrophages M2 cells (Cor > 0.4, p < 0.05).
Figure 2: Heatmap of the difference in expression of immune-related pathway genes in endometriosis vs. normal control. Darker colors indicate greater significant differences.
Figure 3: Relationship between EML4 gene and iimmunity. (A) Comparison of 22 immune cell scores in endometriosis and normal controls (CE). (B) Degree of immune infiltration in endometriosis compared with CE. (C) Correlation analysis of immune score and the EML4 gene expression; (D) Correlation analysis of 22 immune cell scores and EML4 gene expression. *p < 0.05; **p < 0.01; ***p < 0.001; ****p < 0.0001.
Screening of potential regulatory pathways and related gene sets for the EML4 gene
To further explore the mechanism underlying EML4 in contributing to the development of endometriosis, we first investigated the correlation between EML4 and gene pathways using “GSVA,” and found 20 significantly correlated pathways, including 16 positive and 4 negative pathways, for example, ALLOGRAFT_REJECTION, APICAL_JUNCTION, APOPTOSIS, COMPLEMENT, etc. (Cor > 0.4, p < 0.05, Fig. 4). The above results indicated that the EML4 gene was likely to be a key gene in the occurrence of endometriosis. Further, we analyzed the correlation between EML4 gene, and genes in GSE7305 dataset by the rcorr function of the “Hmisc” package and detected 386 significantly related genes under threshold value of cor > 0.6 and p < 0.05. GO enrichment analysis on the EML4 gene set demonstrated that EML4 was mainly associated with immune response (Fig. 5A), among which there were 6,7,72 pathways in Molecular Function (MF, Fig. 5B), Biological Process (BP, Fig. 5C), and Cellular Component (CC, Fig. 5D), including protein serine/threonine kinase activity, endocytosis, and microtubule organizing center.
Figure 4: Heat map of the correlation between the EML4 gene expression and pathway. The darker color indicates the more obvious difference. *p < 0.05; **p < 0.01; ***p < 0.001; ****p < 0.0001.
Figure 5: EML4 gene set enrichment analysis. (A) Bubble diagram of Gene Ontology (GO), Biological Process (BP) enrichment analysis; (B) Bubble diagram of GO, Cellular Component (CC) enrichment analysis; (C) Bubble diagram of GO, CC enrichment analysis; (D) Bubble diagram of Kyoto Encyclopedia of Genes and Genomes (KEGG) enrichment analysis.
Prediction of potential drugs targeting the EML4-associated gene set
As previously mentioned, EML4 was found to be a key gene leading to endometriosis, and therefore it could be reasonably speculated that targeting EML4 may be a potential target for endometriosis treatment. To this end, we calculated the proximity of EML4 to endometriosis according to formula (1) and converted the observed distance into a normalized scoring according to formula (2), following the previously described methods. Both the EML4-related gene sets and our randomly selected gene sets were subjected to multiple hypothesis testing according to the random data obtained, and drugs with distances and FDR < 0.05 were selected. Fig. 6 shows the distance density plot of drugs to the EML4-related gene set. The horizontal axis represents the distance from the gene to the drug, and the vertical axis represents the density between the drug and the gene.
Figure 6: The distance density distribution plot of the drug-to-EML4-related gene set.
We used the SWISS-MODEL (https://swissmodel.expasy.org/) website to perform EML4 homology modeling to obtain the 3D structure of EML4 protein. The Deepsite (https://www.playmolecule.com/deepsite/) website predicted EML4 protein activity (Jiménez et al., 2017). Moreover, Gromacs 2019 software package was applied for prediction of potential small molecule compounds, and a total of five small molecule compounds were identified by calculating the root-mean-square deviation values, namely DB05155, DB05104, DB01113, and DB02942, DB15091 (Table 1). Taken together, DB05104 showed the highest molecular docking score and therefore had higher potential as a potential inhibitor of EML4 protein. Next, we performed a detailed analysis of the interaction of compound DB05104 with EML4 protein, as shown in Fig. 7. DB05104 could bind well to EML4 protein with a relatively reasonable molecular docking score of −8.6 kcal/mol. The compound was able to produce hydrogen bond interaction with GLN551 of EML4 protein and favorable hydrophobic interactions with PHE584 and TRP619. A relatively high molecular docking score and favorable interaction of DB05104 with the EML4 protein suggested that the compound had a great potential to bind to EML4 under physiological conditions to exert corresponding physiological effects.
Figure 7: Binding pattern diagram of EML4 protein with compound DB05104. (A) Surface binding pattern of compound DB05104 with EML4 protein. (B) 2D interaction diagram of compound DB05104 with EML4 protein. (C) Detailed interaction of compound DB05104 with EML4 protein. The protein backbone is shown as a cyan band, compound DB05104 is shown as a yellow stick, the amino acid residues that produce the interaction are shown as cyan sticks, and the color of the heteroatoms in the compound and amino acid residues are shown by element type. Hydrogen bonds are shown as green dashed lines. π-π stacked and π-π T-shaped interactions are shown as magenta dashed lines.
Endometriosis is a relatively common disease among women at their reproductive age, with an annual incidence of 0.1% in females aged 15–49 years old. More than 33% of patients with acute pelvic pain also suffer from endometriosis. Epidemiological findings on women with chronic pelvic pain showed that endometriosis is also closely associated with neoplasia and infertility (Borghese et al., 2018; Viganò et al., 2004). Endometriosis is a multifactorial disease with external environmental, genetic, menstrual, and immune factors involved. Several studies have reported a significant association between immune/autoimmune-mediated diseases and endometriosis (Harris et al., 2016; Nielsen et al., 2011). Studies with different populations have indicated that endometriosis should be considered as much of a concern as other immune disorders and 262 immune-related factors have been identified (Parazzini et al., 2017; Saunders and Horne, 2021; Sinaii et al., 2002).
EML4 consists of a WD repeat protein, a hydrophobic echinoderm microtubule-associated protein-like (HELP) structural domain, and N-terminal basic region (isoelectric point, 10.2). EML4 is a member of the echinoderm microtubule-associated protein-like family. In the predicted fusion proteins, the N terminus of EML4, which contains part of the basic region, HELP region, and WD repeat region, fuse to the intracellular paramembrane region of anaplastic lymphoma kinase (ALK) (Eichenmuller et al., 2002; Smith et al., 1999). Currently, EML4 research mostly focuses on oncology but less on non-tumor aspects; however, a number of studies have confirmed that EML4 plays a significant role in immune system diseases (Osoegawa et al., 2010; Palmer et al., 2009). As shown by de Seny et al. (2021) EML4 is significantly high-expressed in immune diseases, which is also correlated with histological inflammation scores. Consistently, we observed that EML4 expression was upregulated in endometriosis, possibly through the GLYCOSAMINOGLYCAN_BIOSYNTHESIS_ HEPARAN_SULFATE, GLYCOSPHINGOLIPID_BIOSYNTHESIS_GANGLIO_SERIES as well as other pathways to influence the development of endometriosis.
T lymphocyte, as a group of cells with an important role in the immune regulation of the body, suppresses B-cell antibody immune responses, T-lymphocyte proliferative responses, and various effector functions and attenuates the killing activity of T cells and NK cells (Chapman et al., 2020; Dong, 2021; Pallet et al., 2018). Hsu et al. (1997) analyzed peripheral blood and pelvic fluid derived from 36 endometriosis patients and observed a significantly higher level of T-cell secretory factor IL-4 in endometriosis patients than in normal controls, whereas IL-4 decreased to the normal level after laparoscopic surgery and drug treatment. Such findings suggest a role of T cells in the endometriosis immune response, but the exact mechanism is unclear (Hsu et al., 1997). Our study demonstrated that T cells promoted endometriosis through EML4-mediated immune infiltration. Meanwhile, correlation analysis indicated that EML4-mediated T cells may be significantly positively correlated with Macrophages M2 cells, which together influenced endometriosis progression. The enrichment analysis revealed that the above regulation was associated with protein serine/threonine kinase activity, endocytosis, microtubule organizing center, etc.
The current findings supported the role of EML4 as a key gene in affecting endometriosis, and further elucidation of the molecular structure of EML4 will bring a new light on endometriosis management. Inhibition of EML4 is a sensitive target for non-small cell lung cancer treatment, and some patients even survived longer than 99 months (Takahashi et al., 2010). Similarly, a small amount of EML4 expression in non-tumor tissues was detected, and experimental methods such as fluorescence in situ hybridization, western blotting, and immunoprecipitation (Martelli et al., 2009) confirmed a non-tumor-specific nature of EML4, suggesting that EML4 could be a key target for the treatment of both tumor and non-tumor diseases. Improvements in gene profile expression technologies have expanded EML4 drug development to non-tumor areas. Online prediction and homology modeling demonstrated the highest molecular docking score between DB05104 and EML4, suggesting that DB05104 could bind well to EML4 and therefore is a potential inhibitor of EML4 protein and compound for targeting endometriosis.
However, the current research had some limitations. Firstly, the target and signaling pathway of EML4 in the initiation and development of endometriosis was unclear. Though enrichment analysis can help predict potential key molecules and pathways, narrow the scope of study, and improve the efficiency of study, current findings still require validation from genetic data in basic and clinical settings. Second, the current results were based on extrapolation of the raw signal algorithm without independent database validation; in addition, the immune relationship between EML4 and endometriosis has not been fully established and needs to be supported by further laboratory and clinical evidence.
Through genetic and immune correlation analysis, this study primarily revealed that EML4 affects endometriosis through multiple targets and pathways, the mechanism of which is correlated with immune cell activation and infiltration. Subsequently, molecular docking and dynamics simulations verified that the compound DB05104 was a potential inhibitor of EML4 and a potent target for the treatment of endometriosis.
Acknowledgement: Not applicable.
Funding Statement: The subject was funded by the Role and Mechanism of EML4 in Regulating Oocyte Meiosis and Leading to the Infertility Project (SDFEYJGL2103).
Author Contributions: XBF and MYT conducted statistical analyses of the data and prepared the manuscript draft. ZYY and JQD edited the manuscript. CC and HZ provided critical comments to the manuscript. All authors checked and proofread the final version of the manuscript.
Availability of Data and Materials: All data generated or analyzed during this study are included in this published article.
Ethics Approval: Not applicable.
Conflicts of Interest: The authors declare that they have no conflicts of interest to report regarding the present study.
References
Borghese B, Santulli P, Marcellin L, Chapron C (2018). Definition, description, clinicopathological features, pathogenesis and natural history of endometriosis: CNGOF-HAS endometriosis guidelines. Gynecologie Obstetrique Fertilite et Senologie 46: 156–167. [Google Scholar]
Chapman NM, Boothby MR, Chi H (2020). Metabolic coordination of T cell quiescence and activation. Nature Reviews Immunology 20: 55–70. [Google Scholar]
Crispim PCA, Jammal MP, Murta EFC, Nomelini RS (2021). Endometriosis: What is the influence of immune cells? Immunological Investigations 50: 372–388. [Google Scholar]
de Seny D, Baiwir D, Bianchi E, Cobraiville G, Deroyer C et al. (2021). New proteins contributing to immune cell infiltration and pannus formation of synovial membrane from arthritis diseases. International Journal of Molecular Sciences 23: 434. [Google Scholar]
Diao C, Xi Y, Xiao T (2018). Identification and analysis of key genes in osteosarcoma using bioinformatics. Oncology Letters 15: 2789–2794. [Google Scholar]
Dong C (2021). Cytokine regulation and function in T cells. Annual Review of Immunology 39: 51–76. [Google Scholar]
Eichenmuller B, Everley P, Palange J, Lepley D, Suprenant KA (2002). The human EMAP-like protein-70 (ELP70) is a microtubule destabilizer that localizes to the mitotic apparatus. Journal of Biological Chemistry 277: 1301–1309. [Google Scholar]
Ferrero S, Barra F, Leone Roberti Maggiore U (2018). Current and emerging therapeutics for the management of endometriosis. Drugs 78: 995–1012. [Google Scholar]
Giudice LC (2010). Clinical practice. Endometriosis. New England Journal of Medicine 362: 2389–2398. [Google Scholar]
Greene R, Stratton P, Cleary SD, Ballweg ML, Sinaii N (2009). Diagnostic experience among 4,334 women reporting surgically diagnosed endometriosis. Fertility and Sterility 91: 32–39. [Google Scholar]
Harris HR, Costenbader KH, Mu F, Kvaskoff M, Malspeis S, Karlson EW, Missmer SA (2016). Endometriosis and the risks of systemic lupus erythematosus and rheumatoid arthritis in the nurses’ health study II. Annals of the Rheumatic Diseases 75: 1279–1284. [Google Scholar]
Hsu CC, Yang BC, Wu MH, Huang KE (1997). Enhanced interleukin-4 expression in patients with endometriosis. Fertility and Sterility 67: 1059–1064. [Google Scholar]
Jeljeli M, Riccio L.G. C, Chouzenoux S, Moresi F, Toullec L et al. (2020). Macrophage immune memory controls endometriosis in mice and humans. Cell Reports 33: 108325. [Google Scholar]
Jiménez J, Doerr S, Martínez-Rosell G, Rose AS, De Fabritiis G (2017). DeepSite: Protein-binding site predictor using 3D-convolutional neural networks. Bioinformatics 33: 3036–3042. [Google Scholar]
Kim JS, Choi YS, Park JH, Yun J, Kim S et al. (2019). Role of B-cell translocation gene 1 in the pathogenesis of endometriosis. International Journal of Molecular Sciences 20: 3372. [Google Scholar]
Kolanska K, Alijotas-Reig J, Cohen J, Cheloufi M, Selleret L et al. (2021). Endometriosis with infertility: A comprehensive review on the role of immune deregulation and immunomodulation therapy. American Journal of Reproductive Immunology 85: e13384. [Google Scholar]
Lohning AE, Levonis SM, Williams-Noonan B, Schweiker SS (2017). A practical guide to molecular docking and homology modelling for medicinal chemists. Current Topics in Medicinal Chemistry 17: 2023–2040. [Google Scholar]
Martelli MP, Sozzi G, Hernandez L, Pettirossi V, Navarro A et al. (2009). EML4-ALK rearrangement in non-small cell lung cancer and non-tumor lung tissues. American Journal of Pathology 174: 661–670. [Google Scholar]
Nielsen NM, Jørgensen KT, Pedersen BV, Rostgaard K, Frisch M (2011). The co-occurrence of endometriosis with multiple sclerosis, systemic lupus erythematosus and Sjogren syndrome. Human Reproduction 26: 1555–1559. [Google Scholar]
Osoegawa A, Nosaki K, Miyamoto H, Kometani T, Hirai F et al. (2010). Incidentally proven pulmonary “ALKoma”. Internal Medicine 49: 603–606. [Google Scholar]
Pallet N, Fernández-Ramos AA, Loriot MA (2018). Impact of immunosuppressive drugs on the metabolism of T cells. International Review of Cell and Molecular Biology 341: 169–200. [Google Scholar]
Palmer RH, Vernersson E, Grabbe C, Hallberg B (2009). Anaplastic lymphoma kinase: Signalling in development and disease. Biochemical Journal 420: 345–361. [Google Scholar]
Parazzini F, Esposito G, Tozzi L, Noli S, Bianchi S (2017). Epidemiology of endometriosis and its comorbidities. European Journal of Obstetrics, Gynecology, and Reproductive Biology 209: 3–7. [Google Scholar]
Saikia S, Bordoloi M (2019). Molecular docking: Challenges, advances and its use in drug discovery perspective. Current Drug Targets 20: 501–521. [Google Scholar]
Saunders PTK, Horne AW (2021). Endometriosis: Etiology, pathobiology, and therapeutic prospects. Cell 184: 2807–2824. [Google Scholar]
Simoens S, Dunselman G, Dirksen C, Hummelshoj L, Bokor A et al. (2012). The burden of endometriosis: Costs and quality of life of women with endometriosis and treated in referral centres. Human Reproduction 27: 1292–1299. [Google Scholar]
Sinaii N, Cleary SD, Ballweg ML, Nieman LK, Stratton P (2002). High rates of autoimmune and endocrine disorders, fibromyalgia, chronic fatigue syndrome and atopic diseases among women with endometriosis: A survey analysis. Human Reproduction 17: 2715–2724. [Google Scholar]
Smith TF, Gaitatzes C, Saxena K, Neer EJ (1999). The WD repeat: A common architecture for diverse functions. Trends in Biochemical Sciences 24: 181–185. [Google Scholar]
Symons LK, Miller JE, Kay VR, Marks RM, Liblik K, Koti M, Tayade C (2018). The immunopathophysiology of endometriosis. Trends in Molecular Medicine 24: 748–762. [Google Scholar]
Szukiewicz D (2022). Epigenetic regulation and T-cell responses in endometriosis—something other than autoimmunity. Frontiers in Immunology 13: 943839. [Google Scholar]
Takahashi T, Sonobe M, Kobayashi M, Yoshizawa A, Menju T et al. (2010). Clinicopathologic features of non-small-cell lung cancer with EML4-ALK fusion gene. Annals of Surgical Oncology 17: 889–897. https://doi.org/10.1245/s10434-009-0808-7 [Google Scholar] [CrossRef]
Viganò P, Parazzini F, Somigliana E, Vercellini P (2004). Endometriosis: Epidemiology and aetiological factors. Best Practice & Research Clinical Obstetrics & Gynaecology 18: 177–200. [Google Scholar]
Yi M, Nissley DV, McCormick F, Stephens RM (2020). ssGSEA score-based Ras dependency indexes derived from gene expression data reveal potential Ras addiction mechanisms with possible clinical implications. Scientific Reports 10: 10258. [Google Scholar]
Yoshihara K, Shahmoradgoli M, Martínez E, Vegesna R, Kim H et al. (2013). Inferring tumour purity and stromal and immune cell admixture from expression data. Nature Communication 4: 2612. [Google Scholar]
Zhou WJ, Yang HL, Shao J, Mei J, Chang KK, Zhu R, Li MQ (2019). Anti-inflammatory cytokines in endometriosis. Cellular and Molecular Life Sciences 76: 2111–2132. [Google Scholar]
Cite This Article
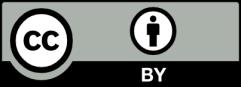